Significant ASR Error Detection for Conversational Voice Assistants
ICASSP 2024 - 2024 IEEE International Conference on Acoustics, Speech and Signal Processing (ICASSP)(2024)
摘要
Modern Automatic Speech Recognition (ASR) systems are evaluated with respect to Word Error Rate (WER). While WER is a useful metric for training and evaluation of speech models, it does not fully reflect the difference in semantics between predicted and ground truth transcriptions. In conversational voice assistants, the ability to sufficiently understand semantic meaning of the user request is often more important than recognizing all words correctly. In this work, we propose a system that can determine, to a high degree of accuracy, whether the semantics of a predicted and reference transcript are significantly different. This knowledge is used to identify ASR errors that can result in downstream failure in conversational voice assistants. Reliable identification of these errors can be used to inform design choices for ASR systems targeting improvement on the most harmful errors.
更多查看译文
AI 理解论文
溯源树
样例
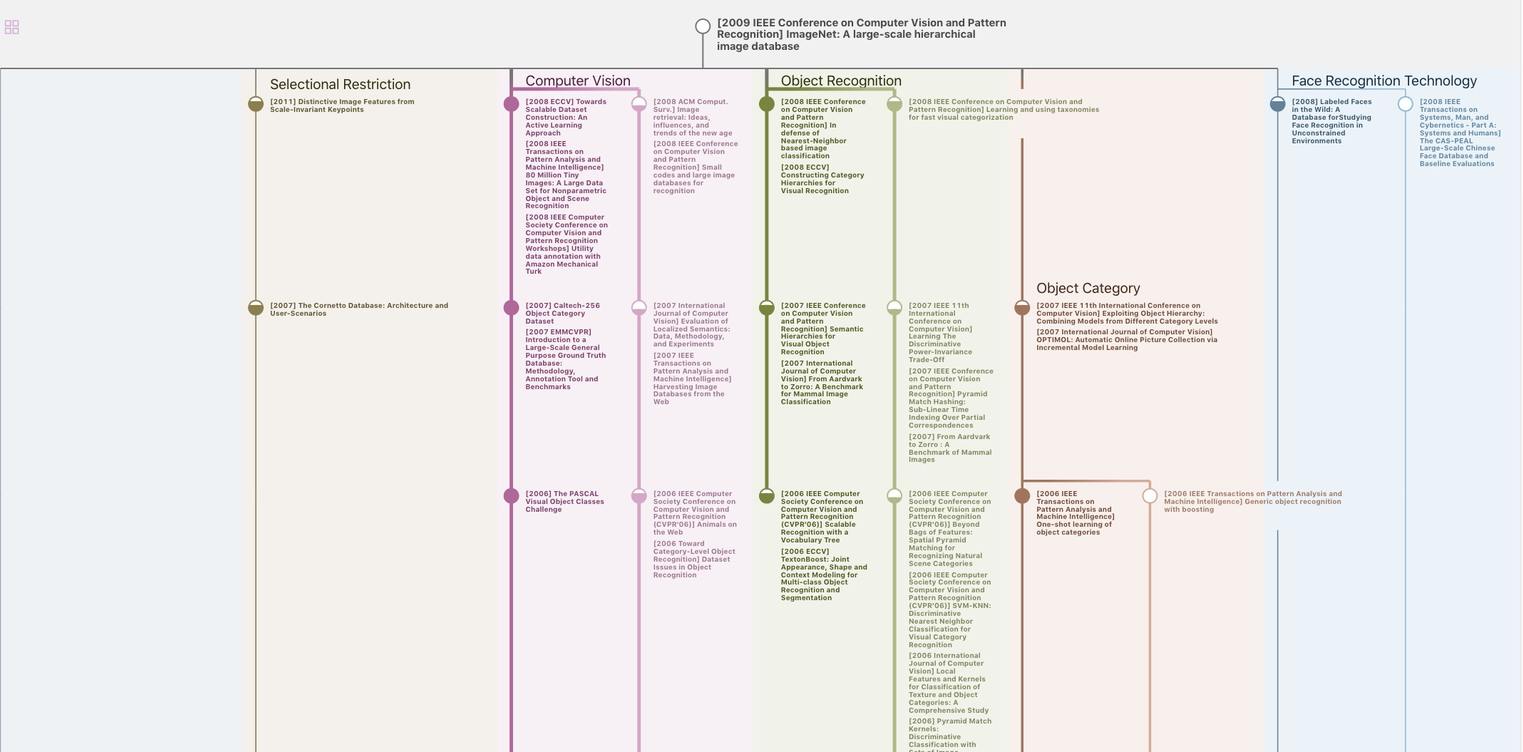
生成溯源树,研究论文发展脉络
Chat Paper
正在生成论文摘要