Optimizing Trading Strategies in Quantitative Markets Using Multi-Agent Reinforcement Learning
ICASSP 2024 - 2024 IEEE International Conference on Acoustics, Speech and Signal Processing (ICASSP)(2024)
摘要
Quantitative markets are characterized by swift dynamics and abundant uncertainties, making the pursuit of profit-driven stock trading actions inherently challenging. Within this context, Reinforcement Learning (RL) — which operates on a reward-centric mechanism for optimal control — has surfaced as a potentially effective solution to the intricate financial decision-making conundrums presented. This paper delves into the fusion of two established financial trading strategies, namely the constant proportion portfolio insurance (CPPI) and the time-invariant portfolio protection (TIPP), with the multi-agent deep deterministic policy gradient (MADDPG) framework. As a result, we introduce two novel multi-agent RL (MARL) methods: CPPI-MADDPG and TIPP-MADDPG, tailored for probing strategic trading within quantitative markets. To validate these innovations, we implemented them on a diverse selection of 100 real-market shares. Our empirical findings reveal that the CPPI-MADDPG and TIPP-MADDPG strategies consistently outpace their traditional counterparts, affirming their efficacy in the realm of quantitative trading.
更多查看译文
关键词
Quantitative trading,multi-agent reinforcement learning,constant proportion portfolio insurance,time-invariant portfolio protection
AI 理解论文
溯源树
样例
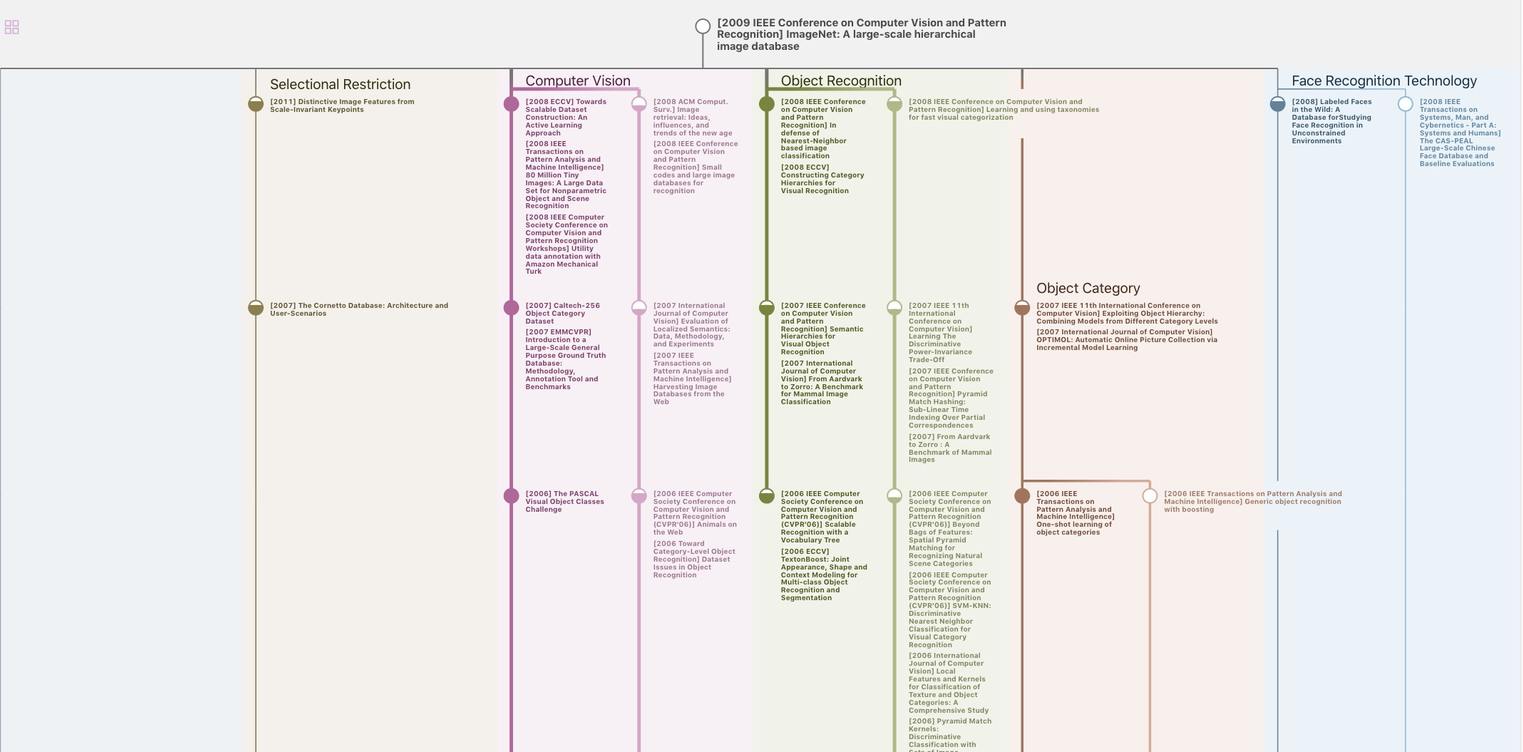
生成溯源树,研究论文发展脉络
Chat Paper
正在生成论文摘要