VL-FAS: Domain Generalization via Vision-Language Model For Face Anti-Spoofing
ICASSP 2024 - 2024 IEEE International Conference on Acoustics, Speech and Signal Processing (ICASSP)(2024)
摘要
Recent approaches have demonstrated the effectiveness of Vision Transformer (ViT) with attention mechanisms for domain generalization of Face Anti-Spoofing (FAS). However, current attention algorithms highlight all the salient objects (e.g., background objects, hair, glasses), which results in the feature learned by the model containing face-irrelevant noisy information. Inspired by existing Vision-language works, we propose the VL-FAS to extract more generalized and cleaner discriminative features. Specifically, we leverage fine-grained natural language descriptions of the face region to act as a task-oriented teacher, directing the model’s attention towards the face region through top-down attention regulation. Furthermore, to enhance the domain generalization ability of the model, we propose a Sample-Level Vision-Text optimization module (SLVT). SLVT uses sample-level image-text pairs for contrastive learning, allowing the visual coder to comprehend the intrinsic semantics of each image sample, thereby reducing the dependence on domain information. Extensive experiments show that our approach significantly outperforms the state-of-the-art and improves the performance of the ViT by about twice.
更多查看译文
关键词
Face Anti-spoofing,Attention Regulation,Vision Language,Domain Generalization
AI 理解论文
溯源树
样例
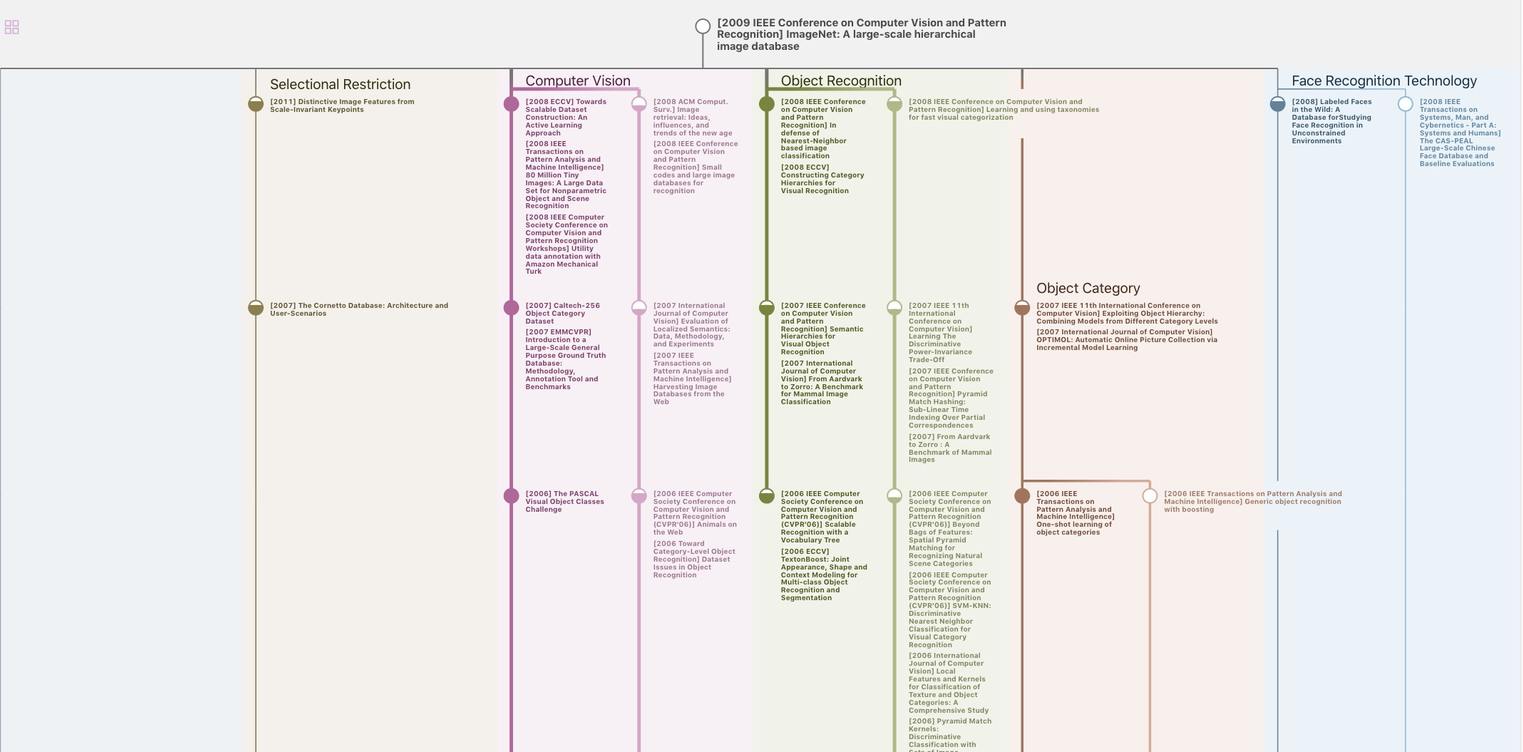
生成溯源树,研究论文发展脉络
Chat Paper
正在生成论文摘要