CKT-RCM: Clip-Based Knowledge Transfer and Relational Context Mining for Unbiased Panoptic Scene Graph Generation
ICASSP 2024 - 2024 IEEE International Conference on Acoustics, Speech and Signal Processing (ICASSP)(2024)
摘要
Panoptic Scene Graph (PSG) generation aims to generate a scene graph representing pairwise relationship between objects within an image. Its use of pixel-wise segmentation mask and inclusion of background regions in relationship inference make it quickly become a popular approach. However, it has an intrinsic challenge that the trained relationship predictors are either of low value or of low quality due to the long-tail distribution of typical datasets. Inspired by how humans use prior knowledge to greatly simplify this problem, we bring in two novel designs, using a pre-trained vision-language model to correct the data skewness, and using conditional prior distribution on contexts to further refine the prediction quality. Specifically, the approach named CKT-RCM first exploits relation-associated visual features from the image encoder and constructs a relation classifier by extracting text embeddings for all relationships from the text encoder of the vision-language model. It also utilizes rich relational context from subject-object pairs to facilitate informative relation predictions via a cross-attention mechanism. We conduct comprehensive experiments on the OpenPSG dataset and achieve state-of-the-art performance.
更多查看译文
关键词
Scene graph generation,panoptic segmentation,visual-linguistic knowledge,attention mechanism
AI 理解论文
溯源树
样例
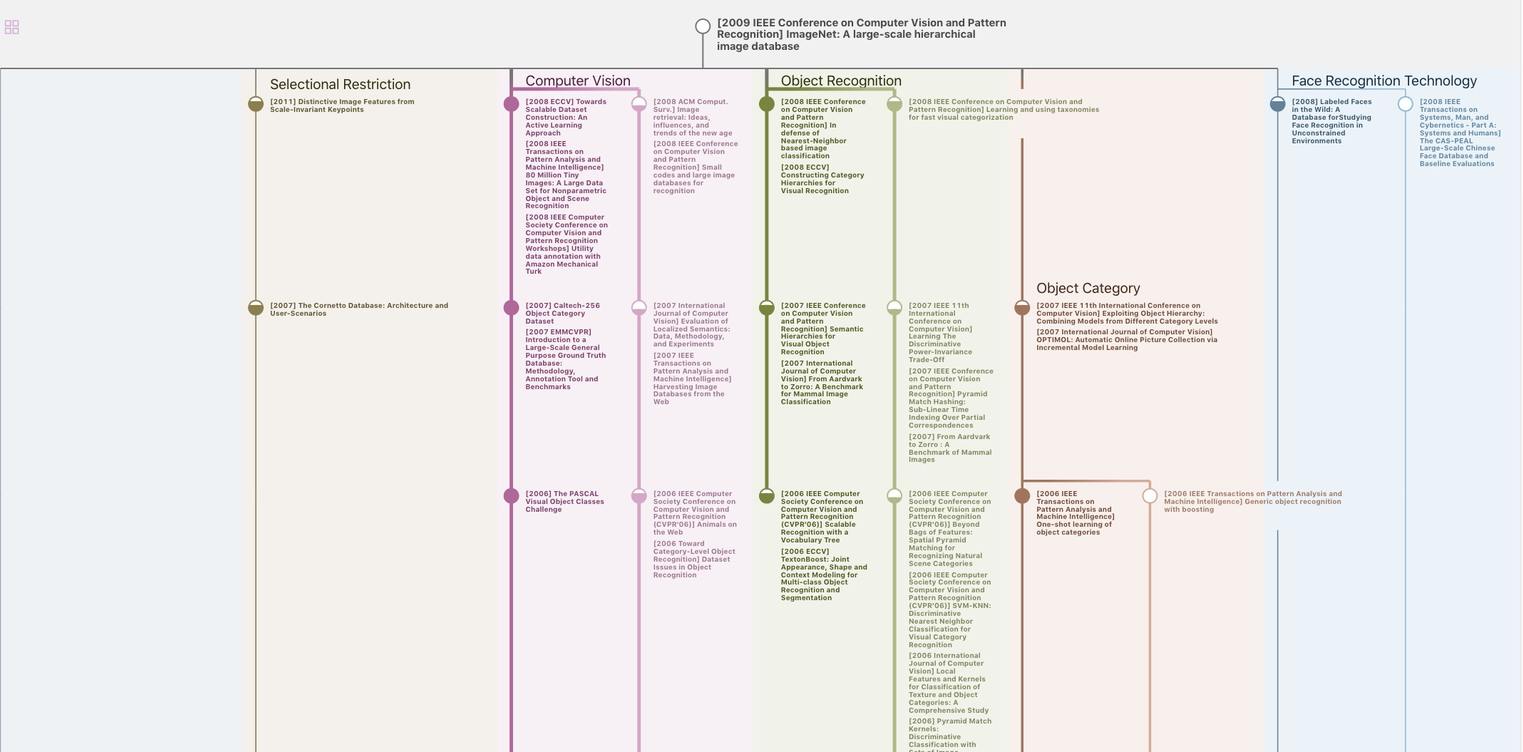
生成溯源树,研究论文发展脉络
Chat Paper
正在生成论文摘要