A Novel Multi-Atlas Fusion Model Based On Contrastive Learning For Functional Connectivity Graph Diagnosis
ICASSP 2024 - 2024 IEEE International Conference on Acoustics, Speech and Signal Processing (ICASSP)(2024)
摘要
Functional connectivity (FC) graph analysis is an important method for diagnosing brain disorders using functional magnetic resonance imaging (fMRI). Existing FC graph diagnosis approaches preprocess the brain by dividing it into specific regions using atlases. However, relying on a single atlas exclusively for data preprocessing fails to fully harness the potential of the medical prior knowledge embedded within these atlases. To address this issue, this paper proposes a functional connectivity graph representation learning method that integrates multiple atlases and multiple views. This self-supervised approach to representation learning aligns features across different atlases through contrastive learning. Building upon this, we introduce an improved finite field- of-view multi-head attention mechanism for feature fusion. This mechanism is used to initialize a spectral graph convolutional network's nodes (GCN) with fusion features obtained through cross-atlas alignment. Meanwhile, edge initialization takes into account the inherent correlations among multiple independent atlas fusion features. The proposed method is validated on multi-site datasets and demonstrates superiority across various metrics. The experimental section provides visualizations of the learned feature alignment and consistency, evaluating the effectiveness of contrastive learning.
更多查看译文
关键词
functional connectivity analysis,population based classification,contrastive learning,feature alignment
AI 理解论文
溯源树
样例
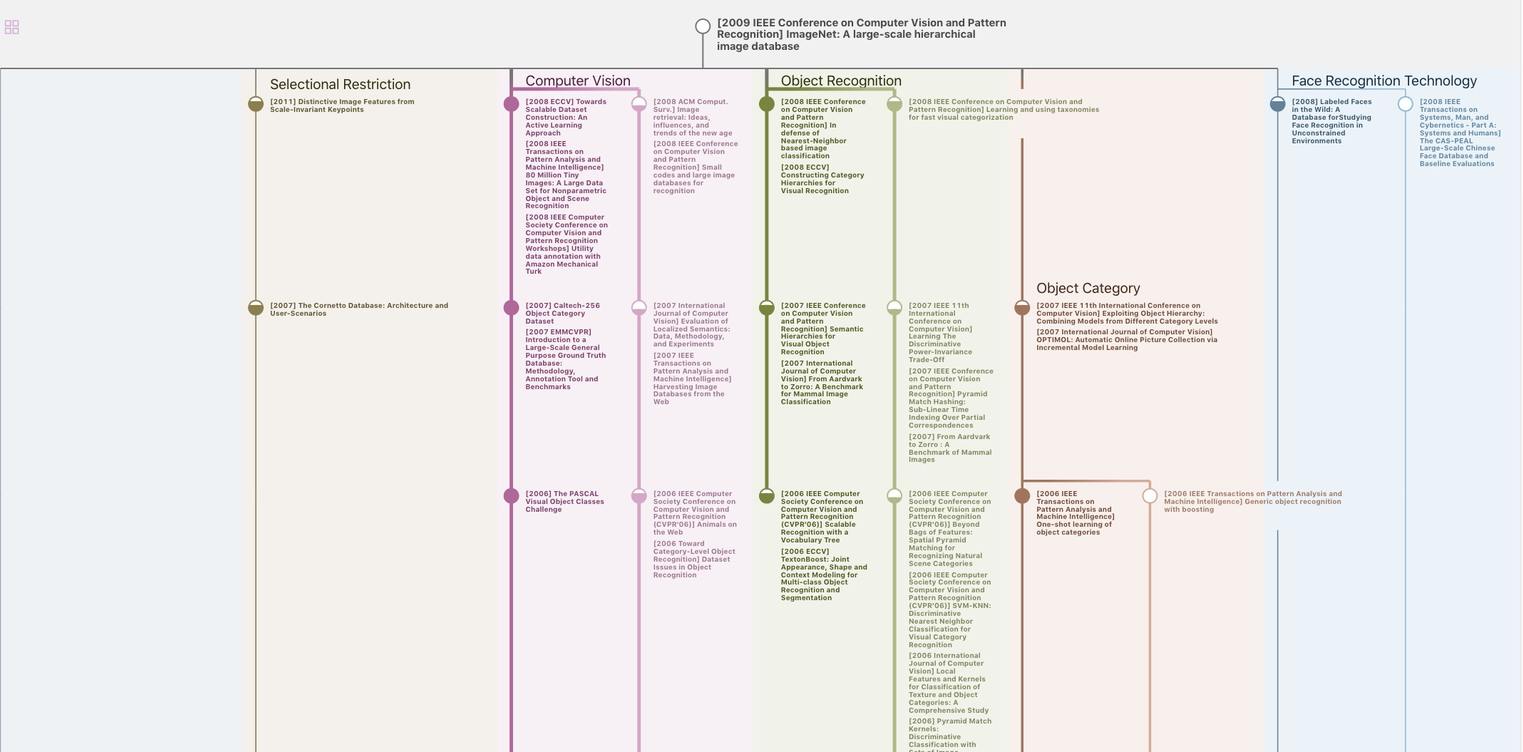
生成溯源树,研究论文发展脉络
Chat Paper
正在生成论文摘要