KD-Former: Transformer Knowledge Distillation for Image Matting
ICASSP 2024 - 2024 IEEE International Conference on Acoustics, Speech and Signal Processing (ICASSP)(2024)
摘要
Vision transformers (ViTs) have been outstanding in multiple dense prediction tasks, including image matting. However, the high computational and training costs of ViTs lead to a bottleneck for applications on low computing power devices. In this paper, we propose a novel transformer-specific knowledge distillation (KD-Former) framework for image matting that can effectively transfer core attribute information to improve the lightweight transformer model. To enhance the information transfer effectiveness in each stage of Vits, we rethink transformer knowledge distillation via dual attribute distillation modules - Token Embedding Alignment (TEA) and Cross-Level Feature Distillation (CLFD). Extensive experiments demonstrate the effectiveness of our KD-Former framework and each proposed key component. Our lightweight transformer-based model outperforms the state-of-the-art (SOTA) matting models on multiple datasets.
更多查看译文
关键词
matting,dense prediction,knowledge distillation
AI 理解论文
溯源树
样例
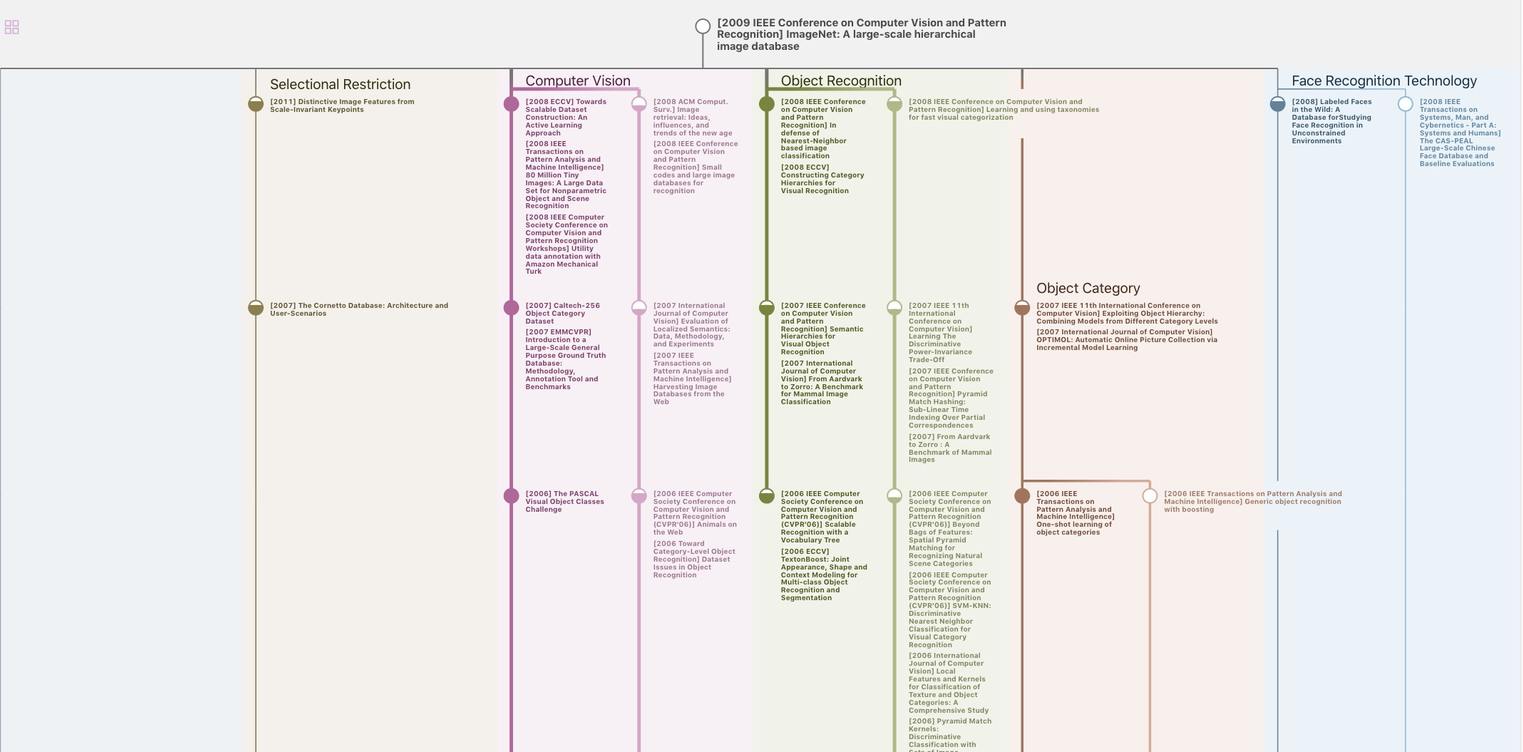
生成溯源树,研究论文发展脉络
Chat Paper
正在生成论文摘要