Electricity Theft Detection Approach Using One-Class Classification for AMI
2024 IEEE 21ST CONSUMER COMMUNICATIONS & NETWORKING CONFERENCE, CCNC(2024)
摘要
The utilization of Advanced Metering Infrastructure (AMI) technology is for recording and billing customers for electricity consumption. This technology is vulnerable to cyberattacks where customers under report their electricity usage, causing financial losses for electricity providers. Machine learning (ML) can be used to detect electricity theft, but it is challenging due to the absence of malicious data. To address this challenge, most of the existing works proposed specific attacks, however, these works are only effective on the proposed attacks and fail on new attacks. Some works proposed using anomaly detectors trained only on begin dataset. However, they rely on specific attacks to set classification thresholds, leading to failure in detecting zero-day attacks. Therefore, this paper proposes a one-class classification approach for electricity theft detection depending only on benign data and without assuming any attacks. First, the paper re-evaluates an existing detector that sets a reconstruction error threshold. Then, it proposes a detector combining decisions from three one-class ML models, including a one-class support vector machine (OC-SVM) trained on benign data, an OC-SVM trained on the bottleneck outputs of an autoencoder trained only on benign data, an OC-SVM trained on the mean squared errors of the reconstructed data of the autoencoder. The evaluation results confirm the superiority of the proposed detector over its individual components and the existing detectors.
更多查看译文
关键词
AMI,electricity theft,machine learning,OC-SVM,autoencoders
AI 理解论文
溯源树
样例
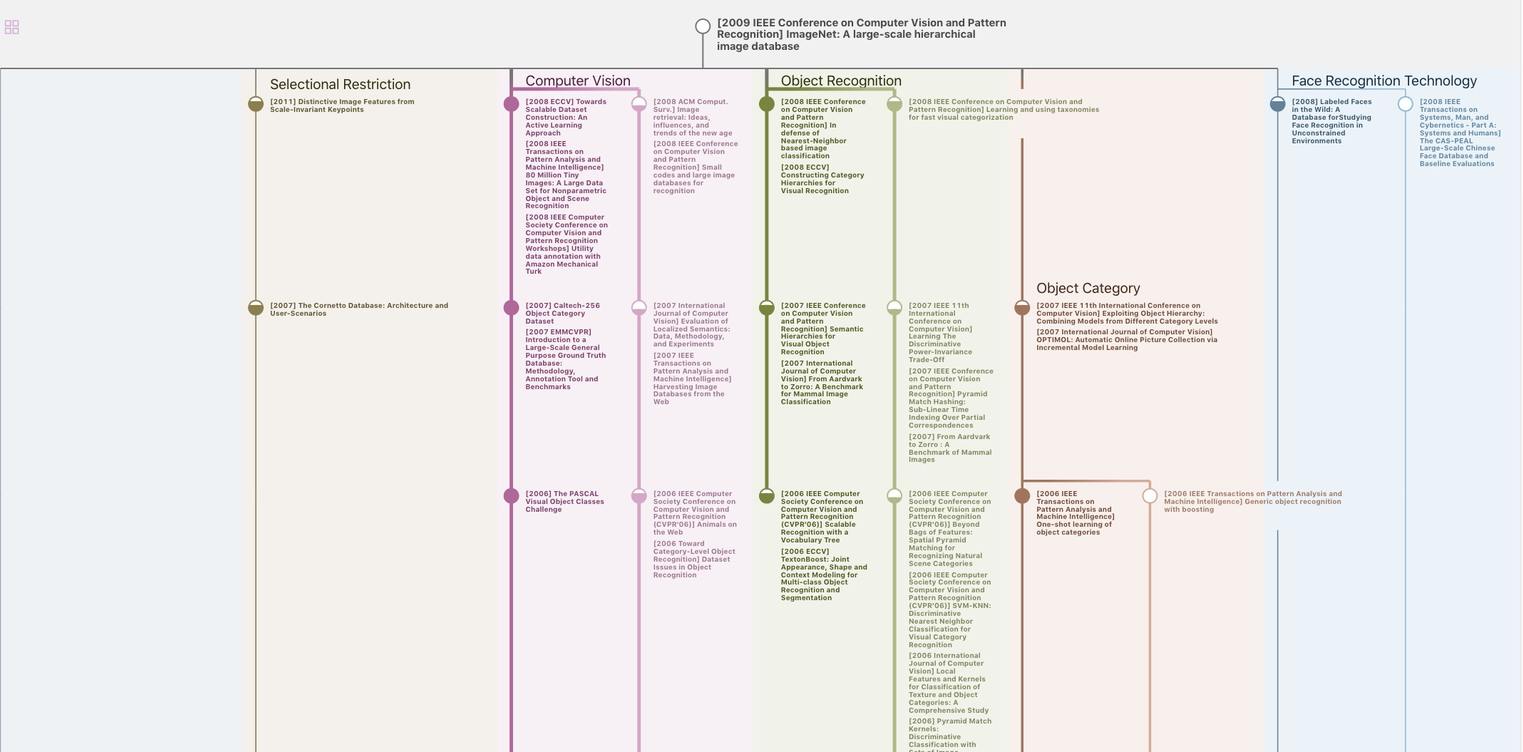
生成溯源树,研究论文发展脉络
Chat Paper
正在生成论文摘要