FAMIM: A Novel Frequency-Domain Augmentation Masked Image Model Framework for Domain Generalizable Face Anti-Spoofing
ICASSP 2024 - 2024 IEEE International Conference on Acoustics, Speech and Signal Processing (ICASSP)(2024)
摘要
While existing face anti-spoofing (FAS) methods have achieved high performance on in-domain datasets, good generalization is crucial for their real-world application. Previous domain generalizable FAS methods have attempted to identify common features of live samples from different domains in the spatial domain, but finding such features is challenging. To address this issue, we propose a solution to the FAS problem in the frequency domain. Specifically, we propose a novel Frequency-domain Augmentation Masked Image Model Framework (FAMIM) for domain generalizable face anti-spoofing. Our approach involves mixing different style information into the input face image in the frequency domain and then reconstructing the original face image. This reconstruction task is designed to make our encoder insensitive to the domain style information of the input face image. Additionally, we design a label fusion module in FAMIM to ensure that the encoder extracts liveness or spoof features in the masked image. Our extensive experiments on four widely used domain generalizable FAS datasets demonstrate that our method achieves state-of-the-art performance.
更多查看译文
关键词
Face anti-spoofing,Domain Generalization,Masked Image Model,Frequency Domain
AI 理解论文
溯源树
样例
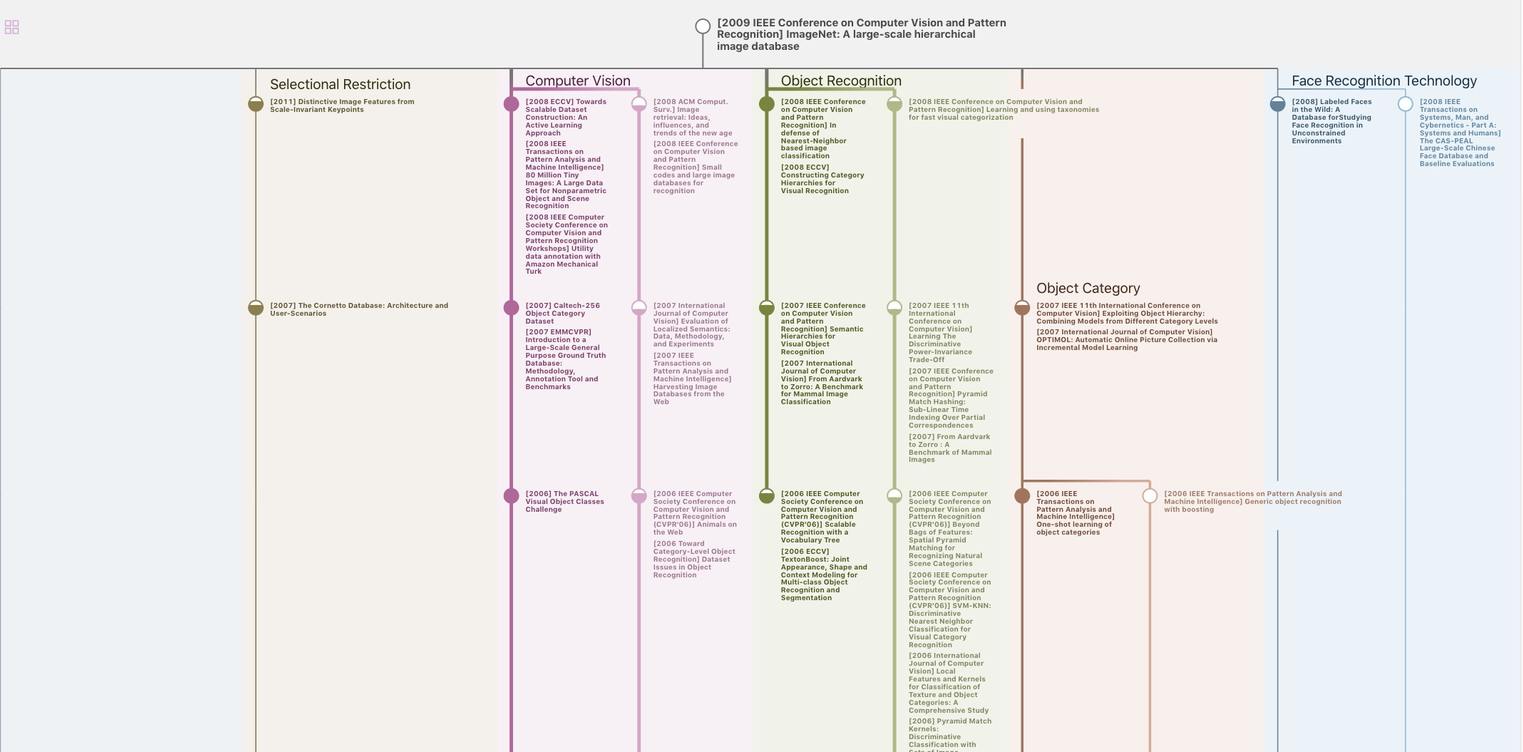
生成溯源树,研究论文发展脉络
Chat Paper
正在生成论文摘要