Investigating the Challenges and Prospects of Construction Models for Dynamic Knowledge Graphs
IEEE access(2024)
摘要
Recently, Dynamic knowledge graphs (DKGs) have been considered the foundation stone for several powerful knowledge-aware applications. DKG has a great advancement over static knowledge graph with the ability to capture the dynamicity of knowledge. The correctness and completeness of DKGs strongly affect the accuracy of the dependent application, in which many factors may have an impact, including data sources, graph construction model, and evaluation methods. Despite the increasing attention to DKGs, the literature of DKG construction is not comprehensively investigated, and the limitations are not fully revealed. In this paper, a comparative study is conducted for the emerging construction models of DKG. An extensive analysis is provided for each of the three main phases of DKG construction: entity extraction, relationship extraction and graph completion. For the different phases, we investigated the employed techniques, the used data sources, as well as the associated challenges, limitations, and evaluation metrics of each model. The learning approach is introduced as a novel categorization perspective for the employed techniques in the DKG construction. Finally, the encountered challenges and limitations are inspected to deduce the possible future directions that can be adopted for effective and advanced DKGs construction. It was found that 100% of the investigated models lack the key aspects of dynamicity in DKGs, 75% suffer from insufficient training features, 58% have a clear exposure to bias, 33% are vulnerable to changes, 25% have a performance and efficiency concerns, while lack of evaluation and comparison represented 25% and 17% of the models respectively.
更多查看译文
关键词
Dynamic knowledge graph,learning approaches,knowledge graph completion,knowledge graph construction
AI 理解论文
溯源树
样例
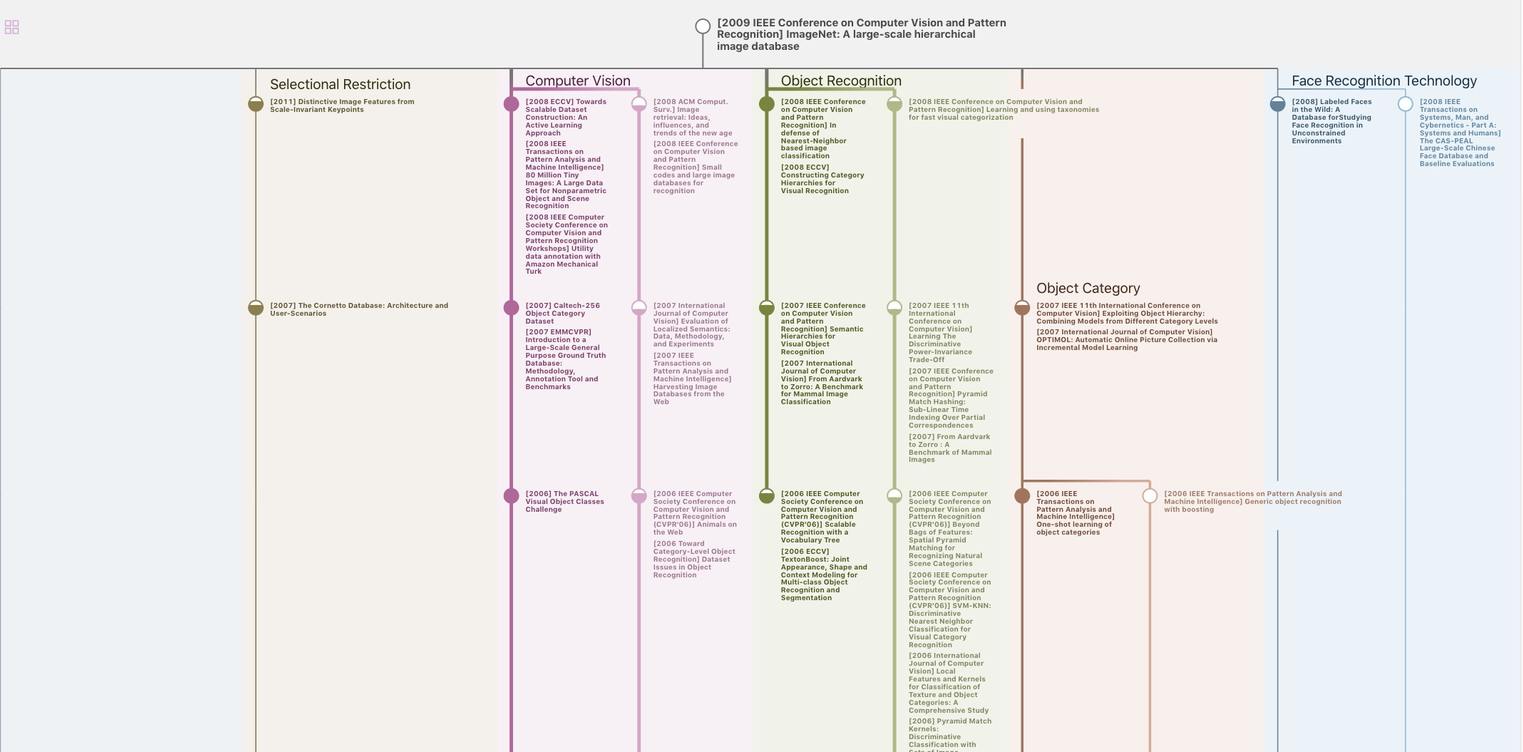
生成溯源树,研究论文发展脉络
Chat Paper
正在生成论文摘要