Task-Wise Prompt Query Function for Rehearsal-Free Continual Learning
ICASSP 2024 - 2024 IEEE International Conference on Acoustics, Speech and Signal Processing (ICASSP)(2024)
摘要
Continual learning (CL) aims to enable a model to retain knowledge of old tasks while learning new ones. One effective approach to CL is based on data rehearsal method. However, this approach increases the cost of storing data and cannot be used when data from old tasks is unavailable for some reason. Recently, with the emergence of large- scale pre-trained transformer models, prompt-based methods have become an alternative to data rehearsal. These methods rely on a query mechanism to generate prompts and have demonstrated resistance to forgetting in CL scenarios without rehearsal. However, these methods generate prompts in a task-wise way while queries for samples in an instance-wise way, and usually directly use pre-trained models as the encoding function for generating queries. This may lead to data retrieval errors and failure to match the correct prompts. In contrast, we propose building a new task-wise prompt query function that can continuously learn as the task progresses, thereby avoiding the issue of pre-trained models being unable to correctly match appropriate sample-prompt pairs. Our approach improves the effectiveness of the current state-of- the-art methods and has been verified on a series of datasets through our experimental results.
更多查看译文
关键词
Continual learning,Incremental learning
AI 理解论文
溯源树
样例
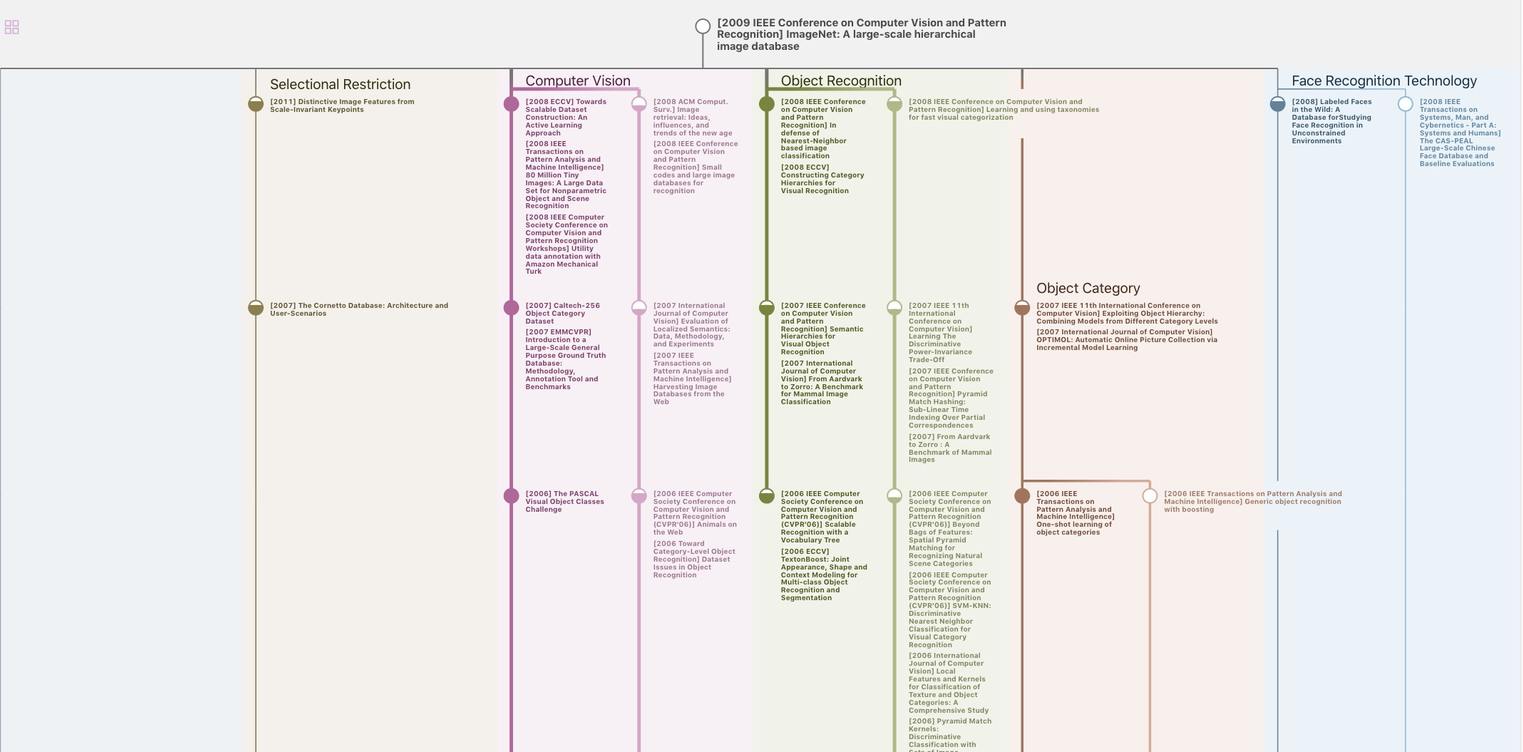
生成溯源树,研究论文发展脉络
Chat Paper
正在生成论文摘要