FEDKA: Federated Knowledge Augmentation for Multi-Center Medical Image Segmentation on non-IID Data
ICASSP 2024 - 2024 IEEE International Conference on Acoustics, Speech and Signal Processing (ICASSP)(2024)
摘要
Federated learning (FL) allows decentralized medical institutions to collaboratively learn a shared global model without breaching data privacy. However, in the context of medical image segmentation, data distributions across centers may vary a lot due to the diverse imaging protocols, vendors and partial annotation, which usually hampers the optimization convergence and the performance of FL. In this paper, we propose a novel approach called federated knowledge augmentation (FedKA) to address the non-IID (non-independent and identically distributed) problem in medical image segmentation within FL. FedKA first designs a pixel-wise knowledge augmentation method to preserve the knowledge of globally labeled regions for the local model during training, and augments each local feature statistical knowledge based on a mixture of Gaussian distribution. Our experiments on public datasets show the superiority of FedKA over the state-of-the-art methods in test performance.
更多查看译文
关键词
Federated learning,Knowledge augmentation,non-IID data
AI 理解论文
溯源树
样例
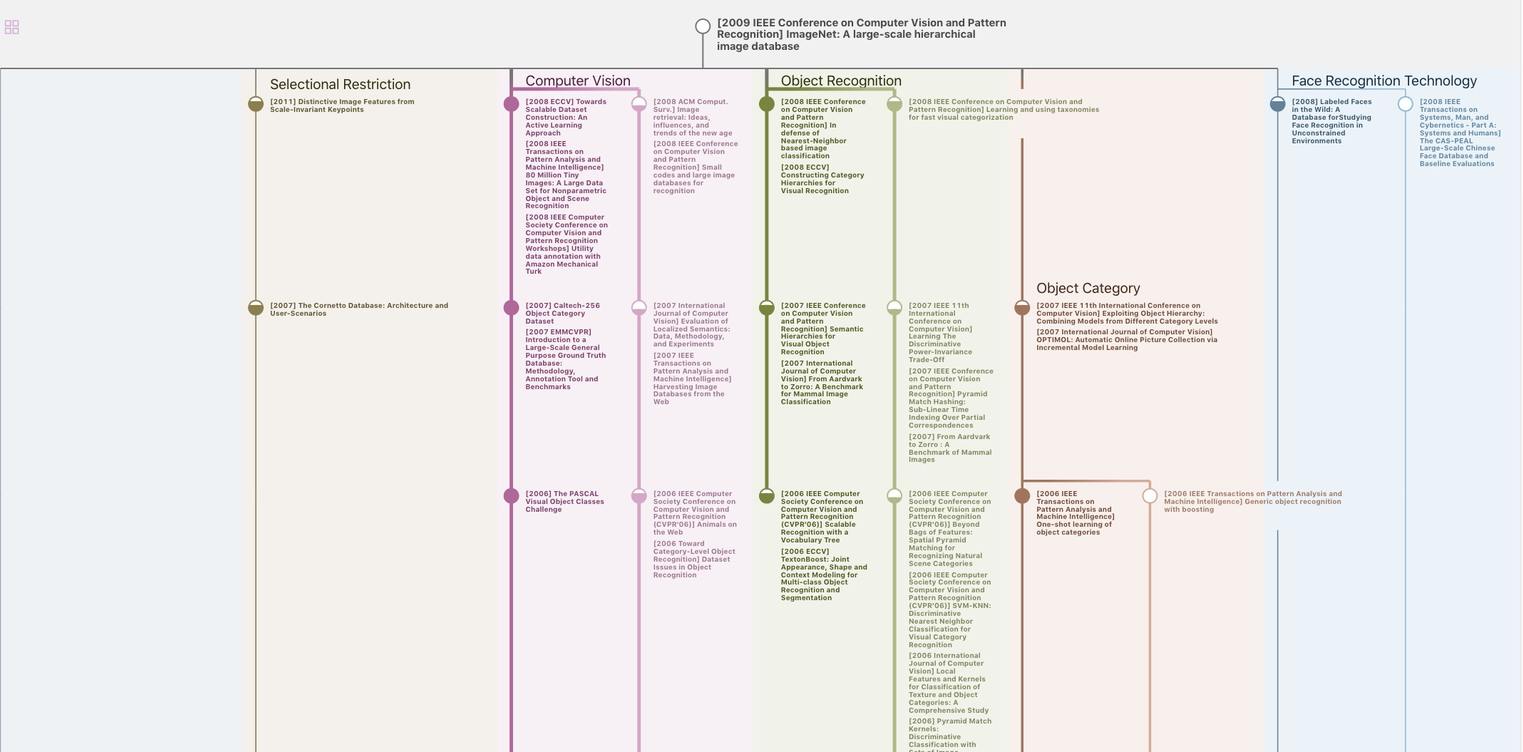
生成溯源树,研究论文发展脉络
Chat Paper
正在生成论文摘要