Parameter-Efficient Adaptation for Computational Imaging
ICASSP 2024 - 2024 IEEE International Conference on Acoustics, Speech and Signal Processing (ICASSP)(2024)
摘要
Deep learning-based methods provide remarkable performance in a number of computational imaging problems. Examples include end-to-end trained networks that map measurements to unknown signals, plug-and-play (PnP) methods that use pretrained denoisers as image prior, and model-based unrolled networks that train artifact removal blocks. Many of these methods lack robustness and fail to generalize with distribution shifts in data, measurements, and noise. In this paper, we present a simple framework to perform domain adaptation as data and measurement distribution shifts. Our method learns a small number of factors to add in a pretrained model to bridge the gap in performance. We present a number of experiments on accelerated magnetic resonance imaging (MRI) reconstruction and image deblurring to demonstrate that our method requires a small amount of memory and parameter overhead to adapt to new domains.
更多查看译文
关键词
Computer Image,Magnetic Resonance Imaging,Network Training,Image Reconstruction,Domain Shift,Performance Gap,Domain Adaptation,Number Of Performance,Neuroimaging,Convolutional Neural Network,Denoising,Convolutional Layers,Ratio Of Samples,Inverse Problem,Forward Model,Target Domain,Gaussian Blur,Input Channels,Pre-trained Network,Network Reconstruction,Noisy Measurements,Blur Kernel,Source Domain,Deblurring,Modulation Technique,Restoration Problem,Motion Blur,Element-wise Product,Measurement Noise,Restoration Tasks
AI 理解论文
溯源树
样例
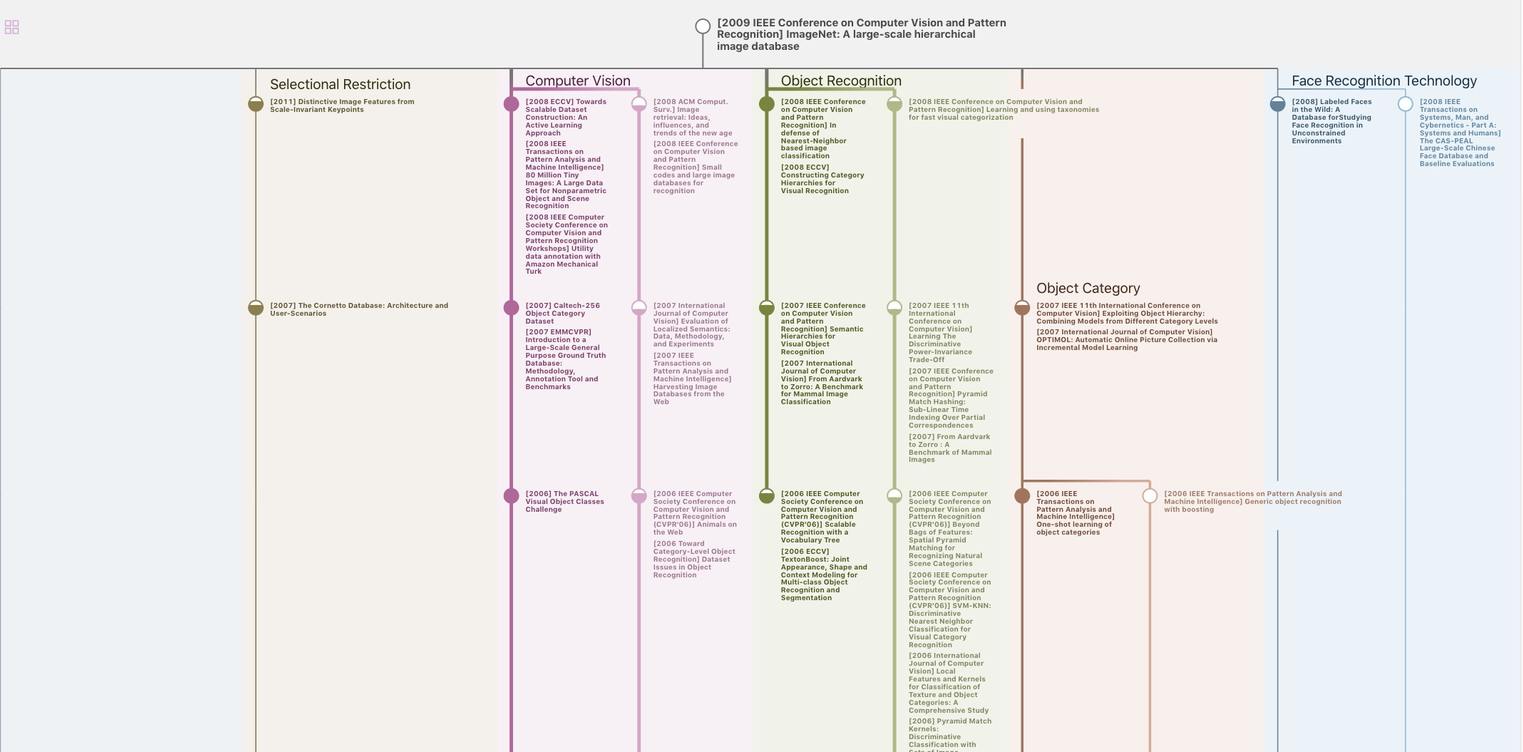
生成溯源树,研究论文发展脉络
Chat Paper
正在生成论文摘要