Improving Speed/Accuracy Tradeoff for Online Streaming ASR via Real-Valued and Trainable Strides
ICASSP 2024 - 2024 IEEE International Conference on Acoustics, Speech and Signal Processing (ICASSP)(2024)
摘要
The Conformer Transducer (CT) is arguably the most popular architecture for online streaming end-to-end (E2E) ASR systems. Since it has quadratic complexity in the input sequence length for computing the attention weights, downsampling the input sequence to reduce its length is an effective way to mitigate the computing cost and speed up the inference process. However, in the traditional downsampling approach, the sampling factor (i.e. stride) has to be a pre-defined integer value. The speed up achieved by such kind of downsampling often comes with significant accuracy degradation, because it lacks the flexibility of trading accuracy with speed at fine-grained level. In this paper, we apply the spectral pooling and DiffStride techniques to the CT based online E2E ASR system. This makes the stride a real-valued trainable parameter. We optimize the implementation of these techniques for CT based ASR systems and develop recipes to train the stride together with the model parameters. We conduct experiments on an internal medical conversation dataset. Our results show that we can achieve better tradeoff between recognition accuracy and inference speed by training real-valued stride parameter. Compared to using decimation with integer stride value, our approach reduces real-time factor by 15.6 % on a medical dataset with less than 1 % relative accuracy degradation.
更多查看译文
关键词
Spectral pooling,DiffStride,online (streaming) ASR
AI 理解论文
溯源树
样例
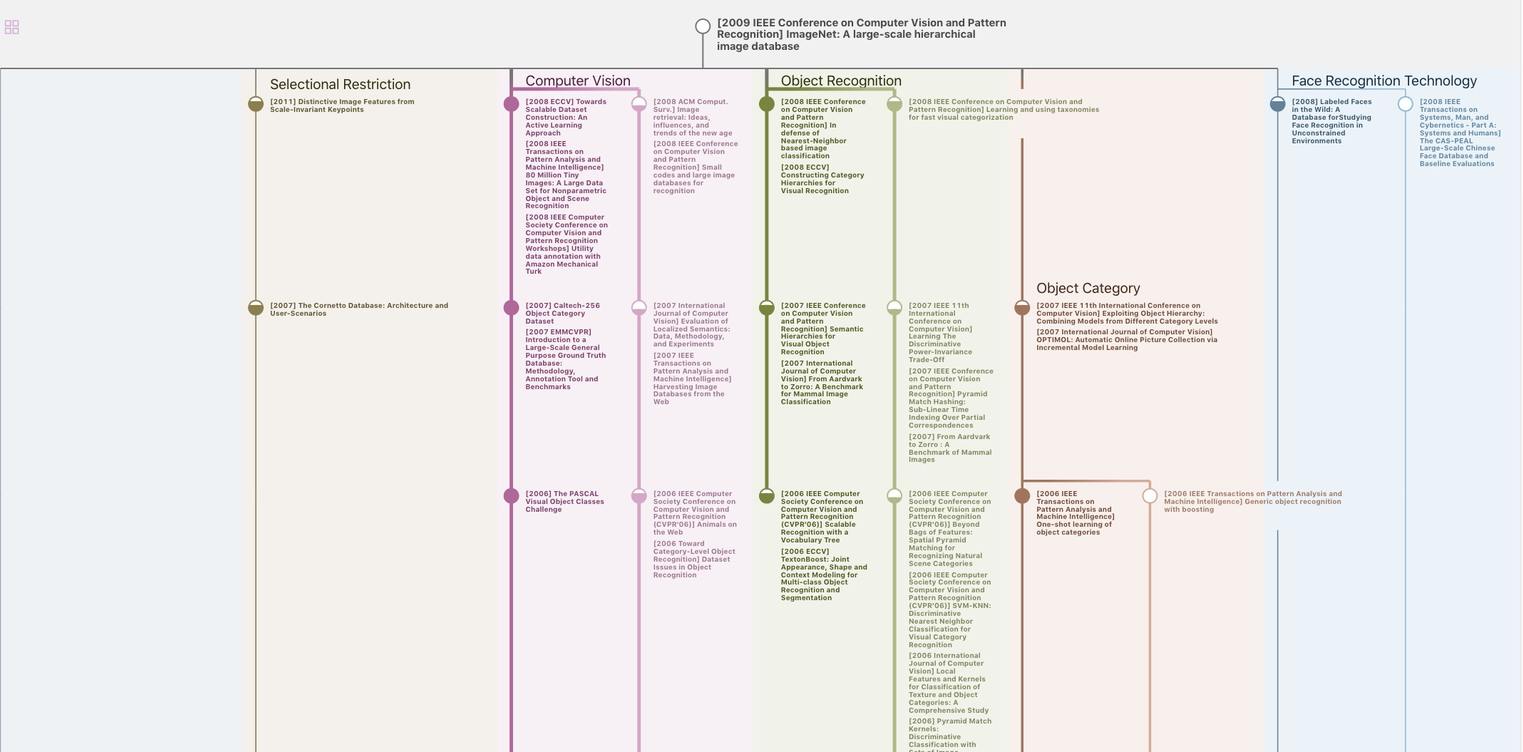
生成溯源树,研究论文发展脉络
Chat Paper
正在生成论文摘要