Efficient and Privacy-Preserving Federated Learning based on Full Homomorphic Encryption
arxiv(2024)
摘要
Since the first theoretically feasible full homomorphic encryption (FHE)
scheme was proposed in 2009, great progress has been achieved. These
improvements have made FHE schemes come off the paper and become quite useful
in solving some practical problems. In this paper, we propose a set of novel
Federated Learning Schemes by utilizing the latest homomorphic encryption
technologies, so as to improve the security, functionality and practicality at
the same time. Comparisons have been given in four practical data sets
separately from medical, business, biometric and financial fields, covering
both horizontal and vertical federated learning scenarios. The experiment
results show that our scheme achieves significant improvements in security,
efficiency and practicality, compared with classical horizontal and vertical
federated learning schemes.
更多查看译文
AI 理解论文
溯源树
样例
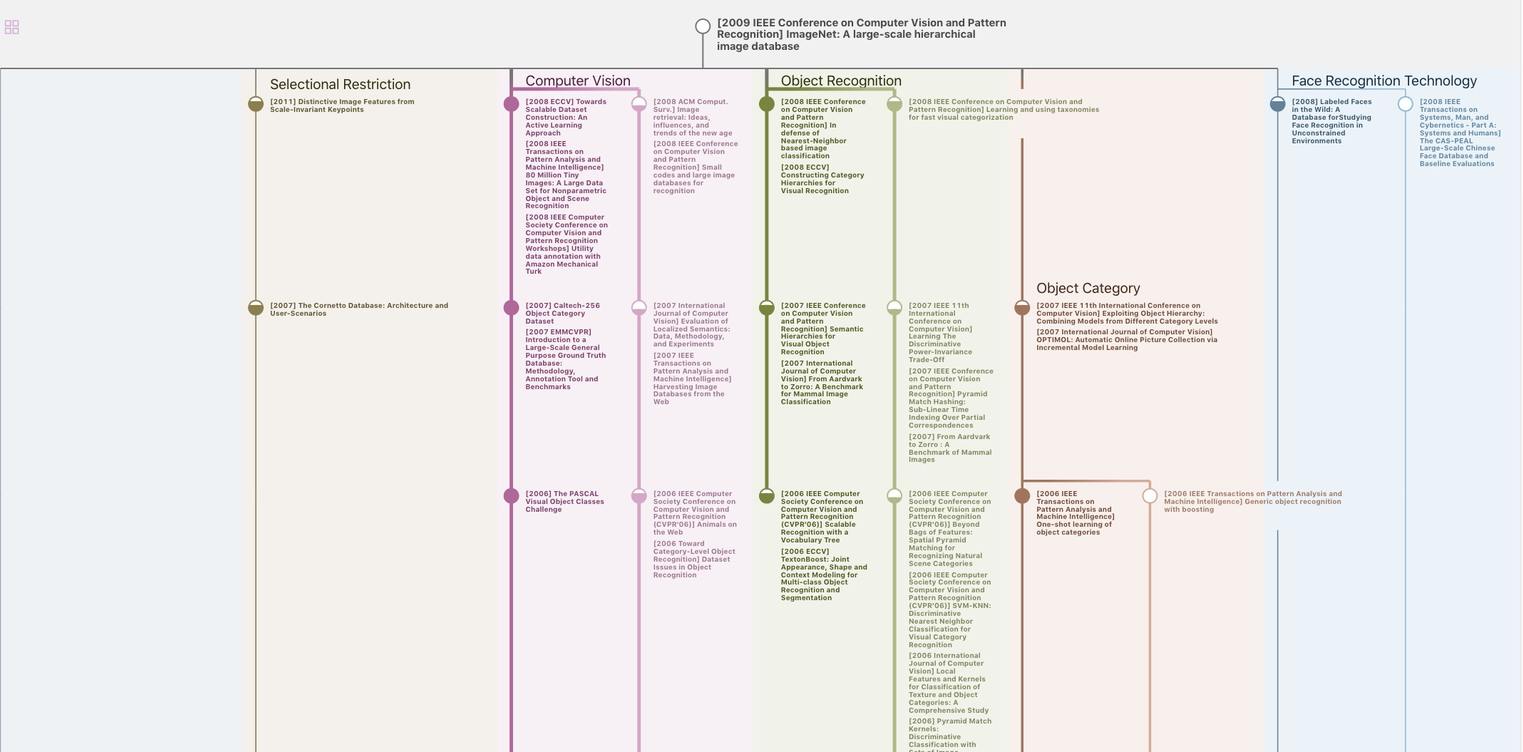
生成溯源树,研究论文发展脉络
Chat Paper
正在生成论文摘要