Multi-Weather Degradation-Aware Transformer for Image Restoration.
IEEE International Conference on Acoustics, Speech, and Signal Processing(2024)
摘要
Restoring images under different adverse weather conditions with a single model is practical in many applications. Most existing weather restoration approaches are only able to handle a specific type of degradation, which is often insufficient in real-world scenarios where the weather type is unknown. In this paper, we propose a holistic solution to solve multiple weather degradations using a single model. Specifically, we build a weather-type aware Transformer, an efficient architecture that can restore images degraded by different adverse weathers with the same set of parameters. For model training, we first use contrastive loss to train an auxiliary hypernetwork capable of extracting content-independent, distortion-aware feature embeddings. Guided by these weather-dependent features, the image restoration Transformer can adaptively modulate its parameters using hypernetworks and feature-wise linear modulation blocks, conducting both local and global operations adaptively for images with different degradations. Qualitative and quantitative results on the multi-weather benchmark demonstrate that our model achieves significant improvements compared with previous state-of-the-arts, with even less computational cost.
更多查看译文
AI 理解论文
溯源树
样例
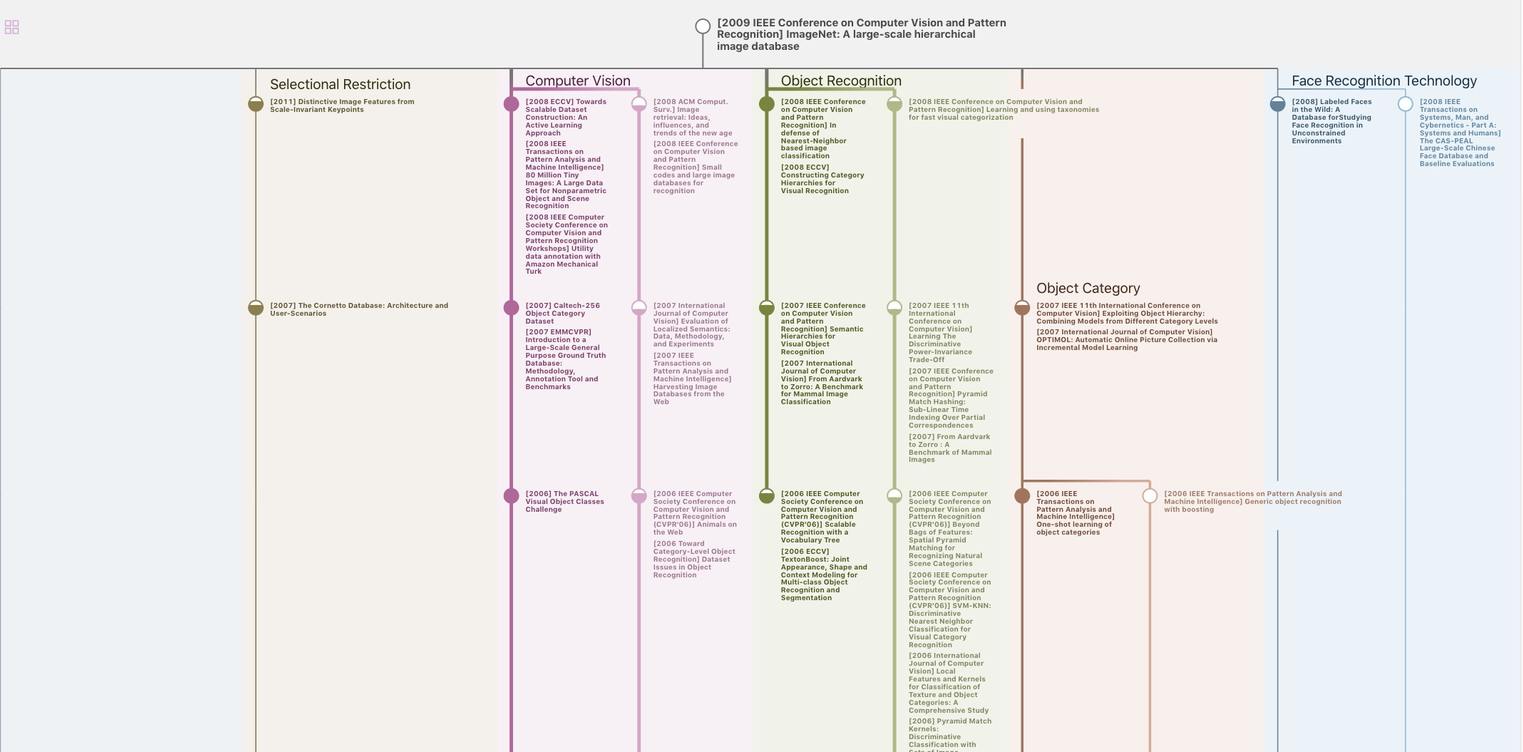
生成溯源树,研究论文发展脉络
Chat Paper
正在生成论文摘要