Anchor-Guided GAN with Contrastive Loss for Low-Resource Out-of-Domain Detection
ICASSP 2024 - 2024 IEEE International Conference on Acoustics, Speech and Signal Processing (ICASSP)(2024)
摘要
Out-of-domain (OOD) detection plays an important role in spoken language understanding (SLU). It can help dialog systems reduce confusion between in-domain (ID) and OOD utterances. Many dialog systems train their model to achieve this goal by collecting annotated OOD and ID data. However, acquiring large-scale OOD datasets can be costly. Recent generative adversarial networks (GANs) based OOD detection methods aim to mitigate this problem. However, their performance in low-resource scenarios remains limited due to a lack of diversity in generated samples and the information contained in the distribution of real samples doesn’t get fully exploited. To address these issues, we propose an Anchor-guided GAN with Contrastive Loss (AGCL) for low-resource OOD detection. In this model, two distinct anchor distributions are established as ground-truth distributions to guide GAN training, which prevents the model from collapsing to a narrow criterion. Furthermore, we introduce an extra contrastive loss for the generator to increase the distinction between the features of generated OOD samples and the limited real OOD samples provided by the dataset, thereby enhancing their diversity. This modification subsequently results in better performance of the anchor-guided GAN. Experimental results demonstrate that our proposed method outperforms existing methods in low-resource scenarios.
更多查看译文
AI 理解论文
溯源树
样例
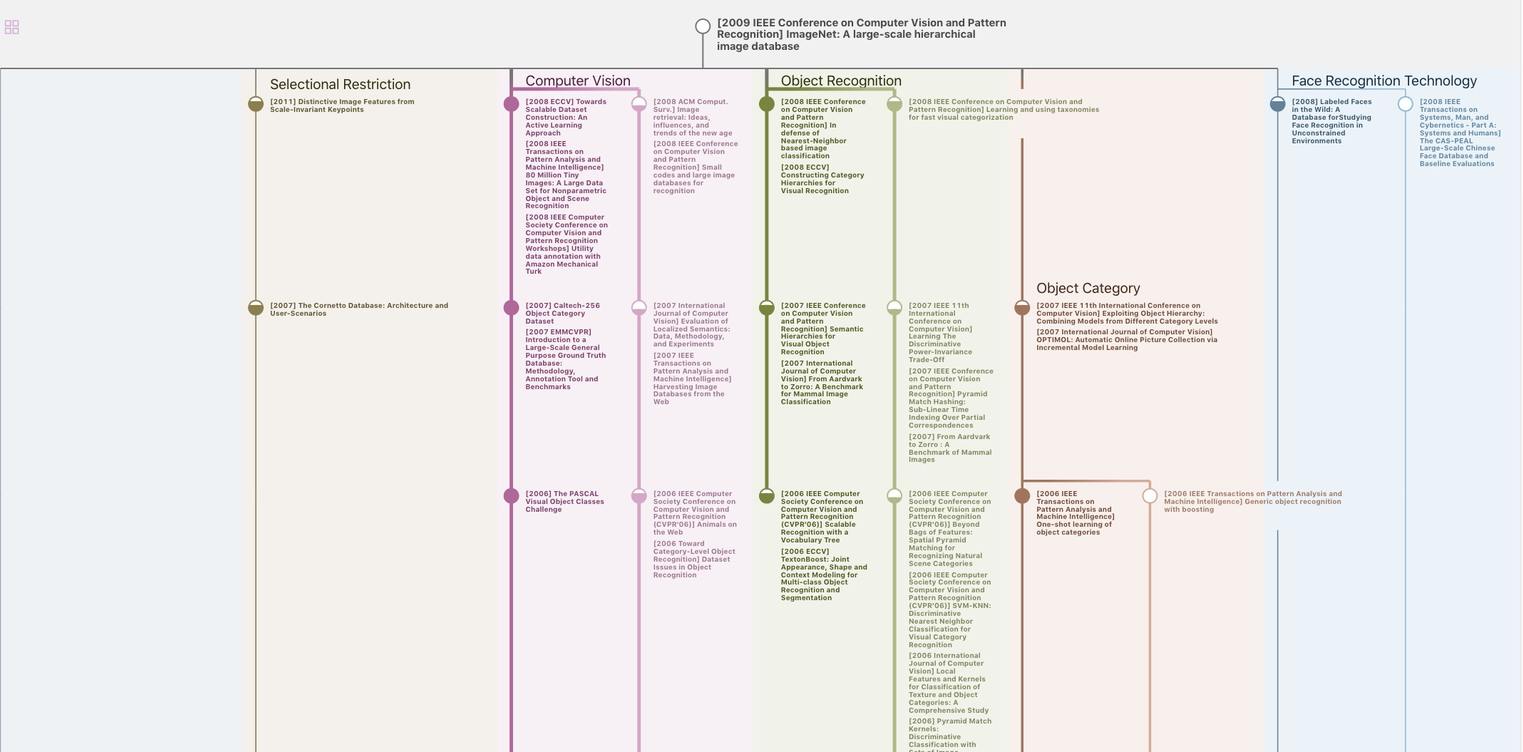
生成溯源树,研究论文发展脉络
Chat Paper
正在生成论文摘要