Chat: Cascade Hole-Aware Transformers with Geometric Spatial Consistency for Accurate Monocular Endoscopic Depth Estimation
ICASSP 2024 - 2024 IEEE International Conference on Acoustics, Speech and Signal Processing (ICASSP)(2024)
摘要
Monocular endoscopic depth estimation is essential for surgical navigation. Current deeply learned estimation methods still suffer from lack of real data labels and porous, artifacts (e.g., bubbles), illumination variations (e.g., specular highlight), and weak texture in endoscopic video images. This paper proposes a new deep learning framework of cascade hole-aware transformers with geometric spatial consistency for accurate endoscopic depth estimation without using any image annotation. Specifically, this framework employs cascade hole-aware encoders to powerfully extract structural features of deep and shallow holes, while it further introduces multiscale filtering decoders to suppress non-hole region features, addressing the problems of specular highlights, weak textures or bubbles. Additionally, a geometric spatial consistency loss can strongly perceive geometric information and suppress the color difference between virtual and real images. We generated virtual endoscopic image data to train our network architecture and test it on both virtual and real endoscopic video images, with the experimental results showing that our method is robust to zero-shot evaluation of real data. Particularly, our method can attain lower root mean square error 1.551±1.147 mm and mean absolute error 1.004±0.632 mm than state-of-the-art deep learning approaches.
更多查看译文
关键词
Monocular Depth Estimation,Bronchoscopic Navigation,Vision Transformers,Virtual Endoscopy
AI 理解论文
溯源树
样例
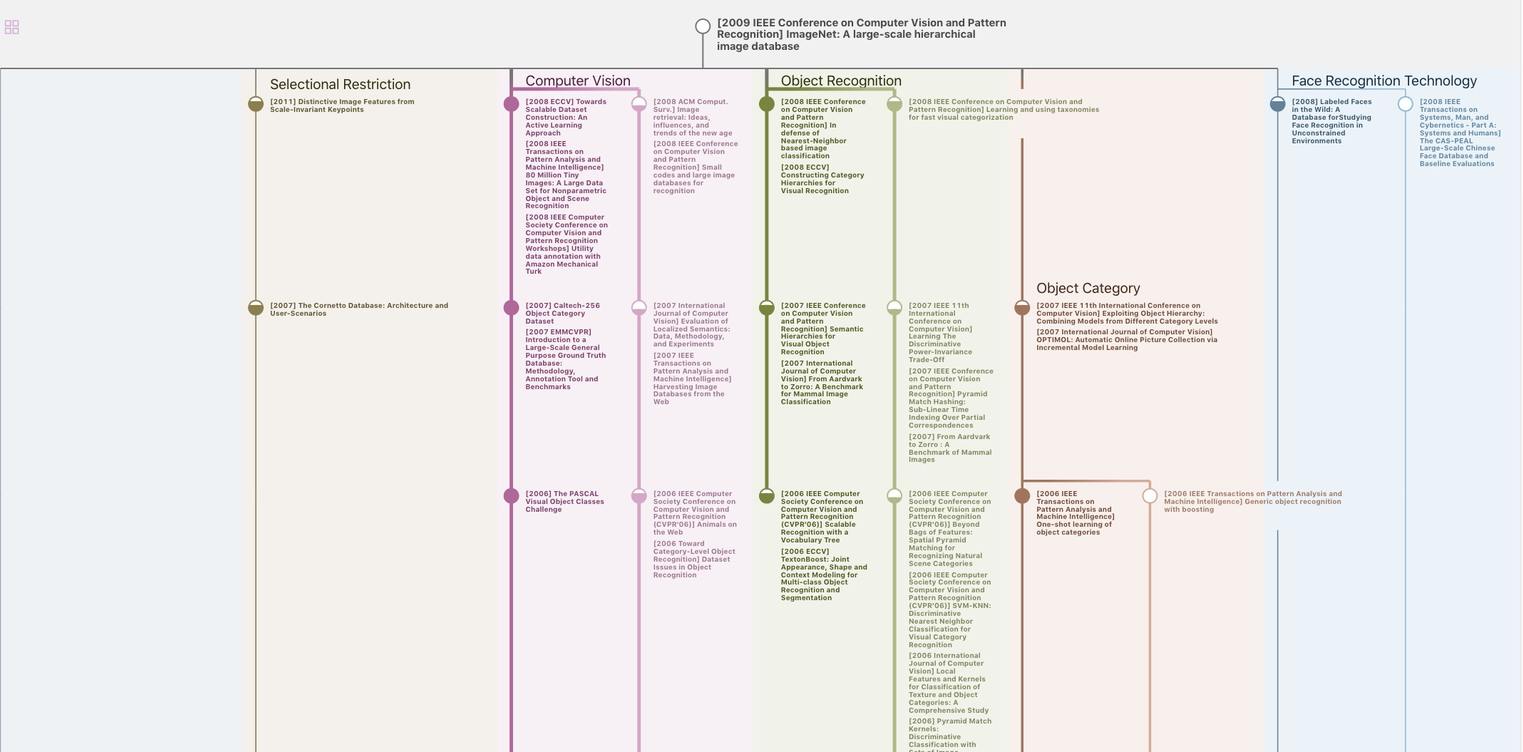
生成溯源树,研究论文发展脉络
Chat Paper
正在生成论文摘要