Dual Directional Complementary Gradient Fusion and Deep Refinement for Hyperspectral Image Super Resolution
ICASSP 2024 - 2024 IEEE International Conference on Acoustics, Speech and Signal Processing (ICASSP)(2024)
摘要
The spatial and spectral resolution trade-off in the hyperspectral imaging is a fundamental and essential issue, and automatically generating high-resolution images in both spatial and spectral domains (HR-HS) by merging a low spatial resolution hyperspectral (LR-HS) image and a high spatial resolution RGB (HR-RGB) image, which are captured by the existing commercial sensors, has recently attracted extensive attention. Motivated by the powerful representation capability of the deep learning networks, current dominated methods have devoted to design deep and complicated network architectures, and manifested great performance progress. This study aims to exploit a simple yet effective deep model by aggregating the complementary missing information into the feature learning branches and automatically modeling the relationship between the target and observations. Specifically, we incorporate the spectral gradient of the LR-HS image with the feature learning branch of the HR-RGB image while aggregate the spatial gradient of the HR-RGB image into the learning branch of the LR-HS image to enhance the representation capability in both spatial ans spectral domains. Moreover, we reconstruct a serials of target HR-HS image from the fused features of dual branches, and employ the un-recovered residuals in the observations by automatically learning the degradation procedure to further refine the former reconstruction in an asymptotic way. Comprehensive experiments have demonstrated that our proposed deep model for HR-HS image reconstruction achieves superior SR performance over state-of-the-art methods in term of quantitative metrics and perceptive quality.
更多查看译文
关键词
Dual complementary gradient fusion,Hyperspectral image super resolution,Deep refinement
AI 理解论文
溯源树
样例
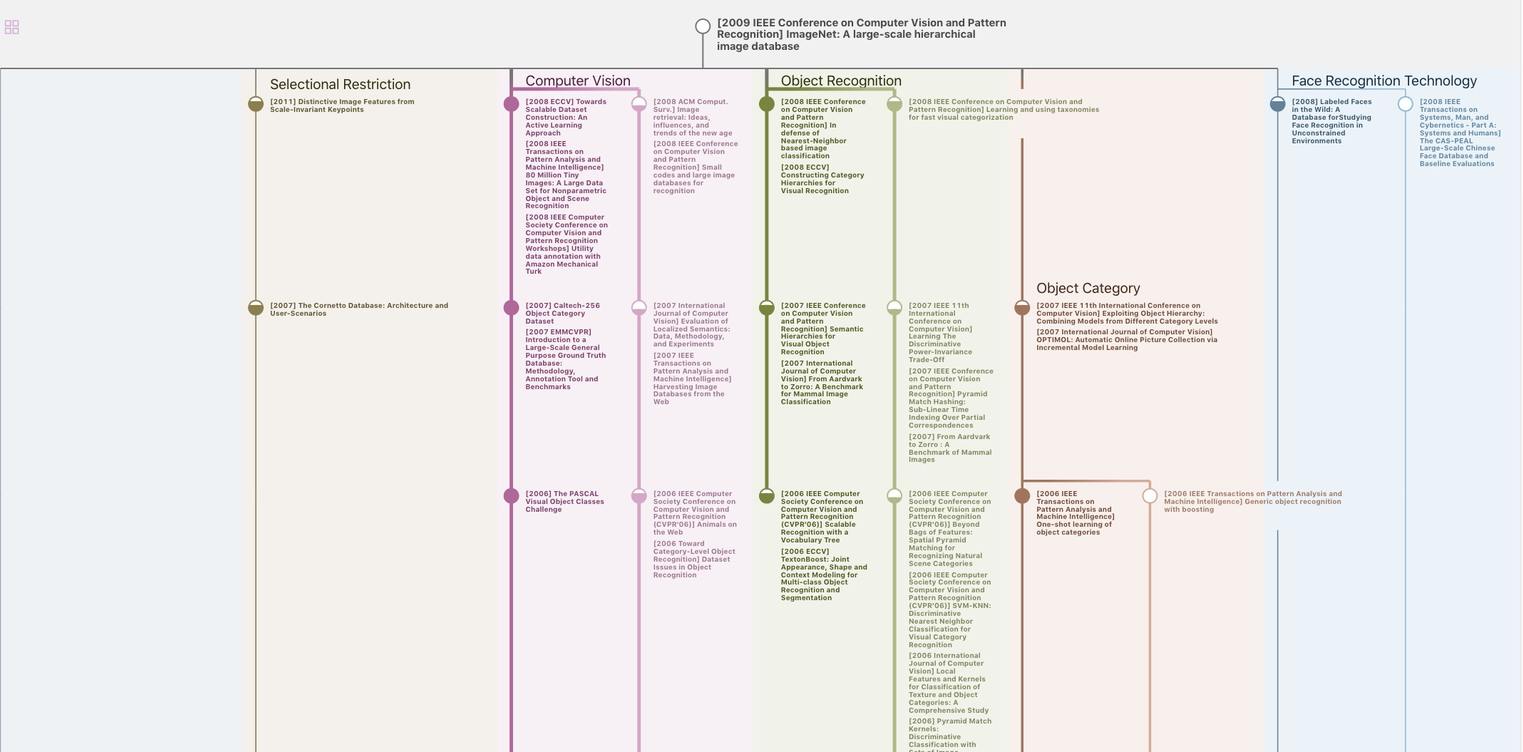
生成溯源树,研究论文发展脉络
Chat Paper
正在生成论文摘要