Cascaded Memory Network for Optical Remote Sensing Imagery Cloud Removal
IEEE TRANSACTIONS ON GEOSCIENCE AND REMOTE SENSING(2024)
摘要
Cloud removal is an inevitable task in optical remote sensing images, which aims at restoring high-quality images from cloud-contaminated images. In recent years, deep learning-based image cloud removal methods have utilized convolution neural networks to obtain clean images. However, due to the limitations of the convolution operator, these methods cannot effectively leverage the local and global information of the image. In this article, we propose a cascaded memory network (CMNet) for optical remote sensing imagery cloud removal. The CMNet recycles previously captured information to form a memory mechanism, which is composed of two cascaded components: local information memory module (LIMM) and global information auxiliary module (GIAM). The LIMM aims to obtain local spatial details information of the image via two subnetworks, and the GIAM tries to further restore the detail of the image from a global perspective. In the LIMM, two subnetworks are constructed to capture the details from coarse to fine, each of which includes continuous memory descriptors that describe the local details of the image and hierarchical memory correlation descriptors that adaptively integrate relevant features. In the GIAM, we design the Swin cloud remove transformer layer and explore an adaptive normalization (AdaNorm) to cope with unevenly distributed thin clouds, and further provide theoretical proof for the existence of the required solution. Experimental results indicate that our method can remove clouds while maintaining the detailed information of the image. The code is available at https://github.com/Lab-PANbin/.
更多查看译文
关键词
Convolution,Remote sensing,Correlation,Optical sensors,Transformers,Optical imaging,Task analysis,Cascaded memory network (CMNet),cloud removal,remote sensing images
AI 理解论文
溯源树
样例
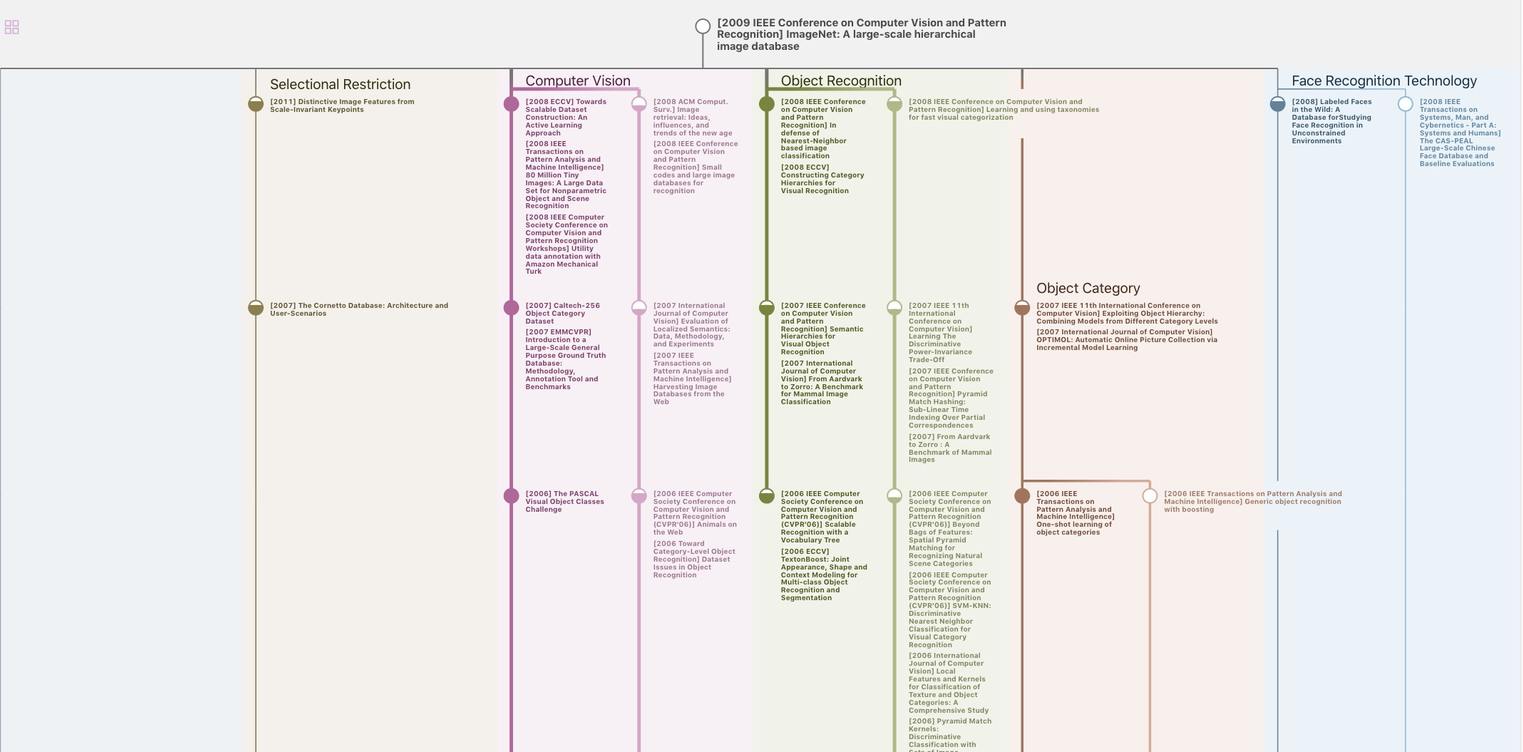
生成溯源树,研究论文发展脉络
Chat Paper
正在生成论文摘要