Joint Blind Deconvolution And Demixing Of Sparse Signals Via Factorization And Nonconvex Optimization
ICASSP 2024 - 2024 IEEE International Conference on Acoustics, Speech and Signal Processing (ICASSP)(2024)
摘要
The problem of joint blind deconvolution and demixing for sparse signals is prevalent in many signal processing areas. The goal of this problem is to recover both the sparse signals and the filters from a noisy mixture of bilinear measurements. Due to the bilinear factorization structure, the common solving approach is based on the matrix-lifting semidefinite programming. In this paper, we consider a nonconvex problem formulation for this problem based on matrix factorization. We propose a nonconvex optimization algorithm based on the block majorization-minimization (BMM) framework. In each iteration of BMM, signals and filters are updated with analytical solutions in an alternating way. In comparison to state-of-the-art algorithms, BMM has much lower per-iteration computational complexity and hence is more scalable to large-size problems. Numerical results show that BMM is able to recover the sparse signals and filters with higher precision as well as faster convergence compared to existing methods.
更多查看译文
关键词
Blind deconvolution,demixing,sparsity,nonconvex optimization,majorization-minimization
AI 理解论文
溯源树
样例
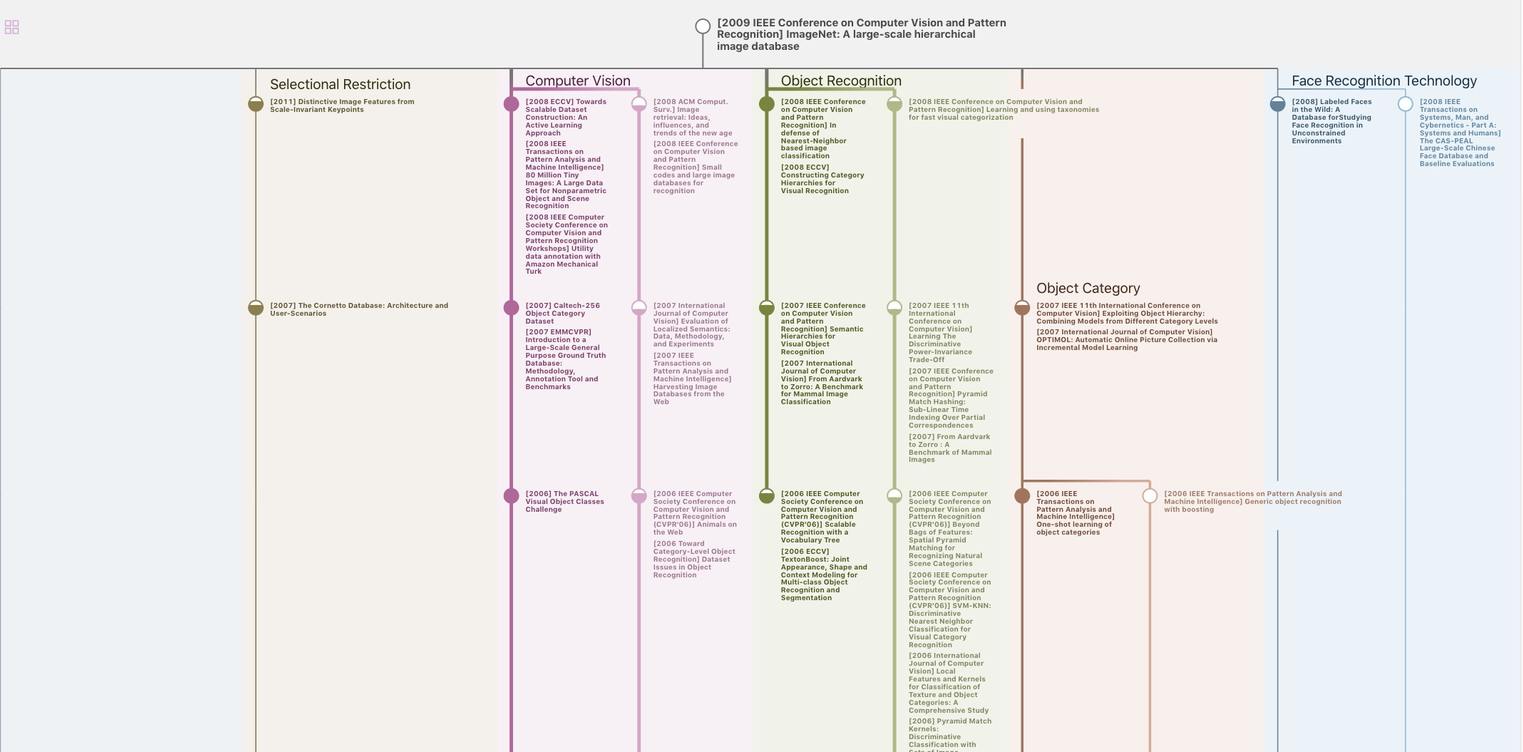
生成溯源树,研究论文发展脉络
Chat Paper
正在生成论文摘要