Model Falsification from a Bayesian Viewpoint with Applications to Parameter Inference and Model Selection of Dynamical Systems
JOURNAL OF ENGINEERING MECHANICS(2024)
摘要
This work provides a Bayesian reinterpretation of model falsification. We show that model falsification can be viewed as an approximate Bayesian computation (ABC) approach when hypotheses (models) are sampled from a prior. To achieve this, we recast model falsifiers as discrepancy metrics and density kernels such that they may be adopted within ABC and generalized ABC (GABC) methods. We call the resulting frameworks model-falsified ABC and model-falsified GABC, respectively. As a result of our reinterpretation, the set of unfalsified models can be shown to be realizations of an approximate posterior. We consider both error- and likelihood-domain model falsification. Model-falsified (G)ABC is used to address two practical types of inverse problems, although with synthetic measurements. The first two problems concern parameter estimation and include applications of ABC to the inference of a statistical model in which the likelihood can be difficult to compute, and the identification of a cubic-quintic dynamical system. The third example involves model selection for the base isolation system of a four-degree-of-freedom base-isolated structure. The performance of model-falsified ABC and GABC is compared with that of Bayesian inference. The results show that model-falsified (G)ABC can be used to solve inverse problems in a computationally efficient manner. The results are also used to compare the various falsifiers in terms of their capability to approximate the posterior and some of its important statistics. Furthermore, we show that model falsifier-based density kernels can be used in kernel regression to infer unknown model parameters and compute structural responses under epistemic uncertainty.
更多查看译文
AI 理解论文
溯源树
样例
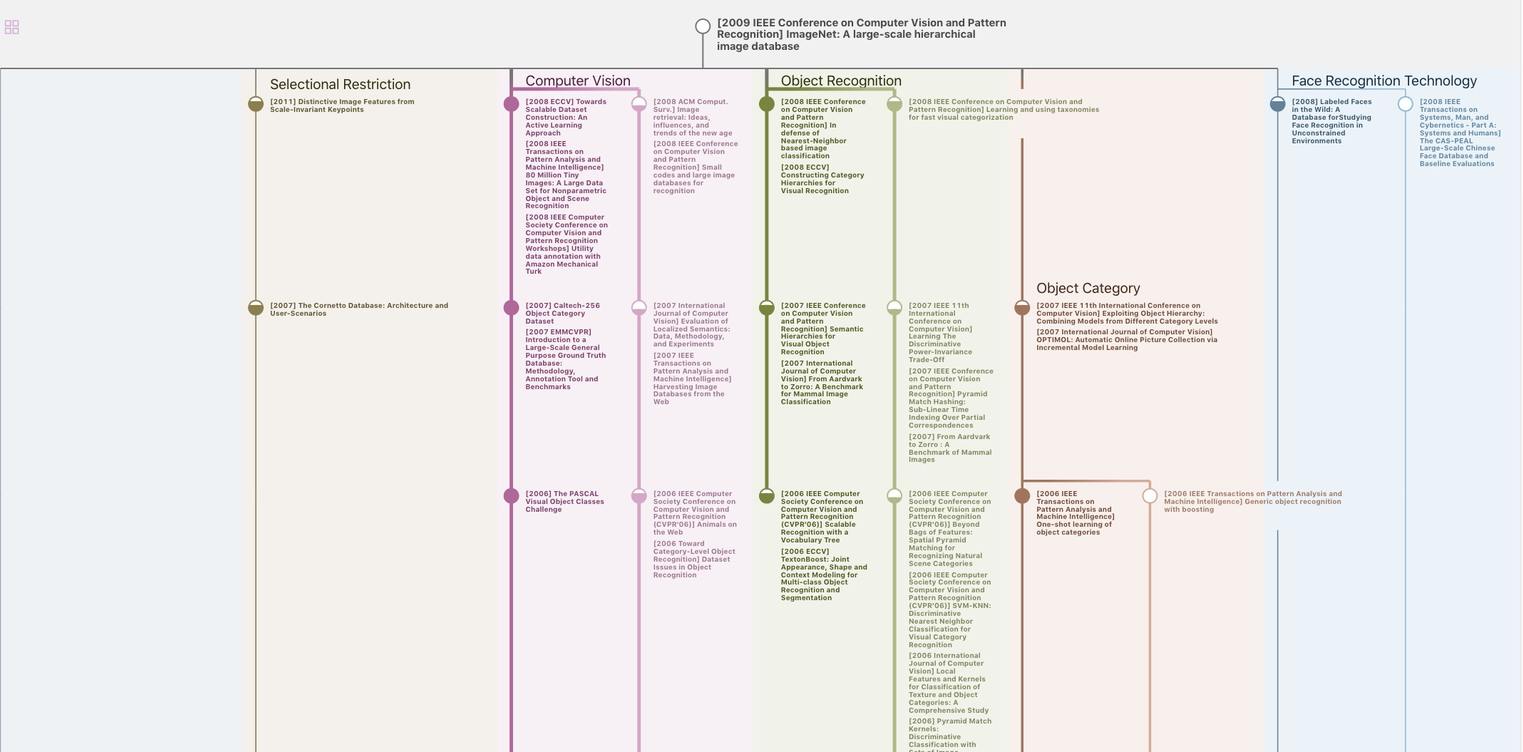
生成溯源树,研究论文发展脉络
Chat Paper
正在生成论文摘要