Language-Driven Ordinal Learning for Imbalanced Head Pose Estimation
ICASSP 2024 - 2024 IEEE International Conference on Acoustics, Speech and Signal Processing (ICASSP)(2024)
摘要
Head pose estimation aims to predict three degrees of freedom pose angles in an unconstrained environment. Conventional ordinal learning methods project the input in a one-dimensional label distribution, with preserving ordinal relationship among labels. However, this assumption frequently fails to hold in multi-dimensional head pose data, resulting in performance in imbalanced data despite extensive training. To address this issue, our model calibrates to sparse multi-dimensional distributions by forging a connection between the order concept in human language and the ordinal characteristics of pose labels. Our approach endeavors to utilize linguistic ordering properties to compensate for potential data scarcity in certain continuous labels. Specifically, we incorporate an ordinal pose prompt to leverage the inherent ranking relationship, and in turn, expand the pose regression boundary. We further endorse the use of real-valued encoding for label representation to subtly model prediction distributions, thereby achieving a balanced prediction of the training sample distribution. Experimental results across multiple datasets confirm that our method achieves compelling performance existing state-of-the-art techniques without auxiliary data.
更多查看译文
关键词
Head pose estimation,ordinal regression,imbalance classification,vision-language learning
AI 理解论文
溯源树
样例
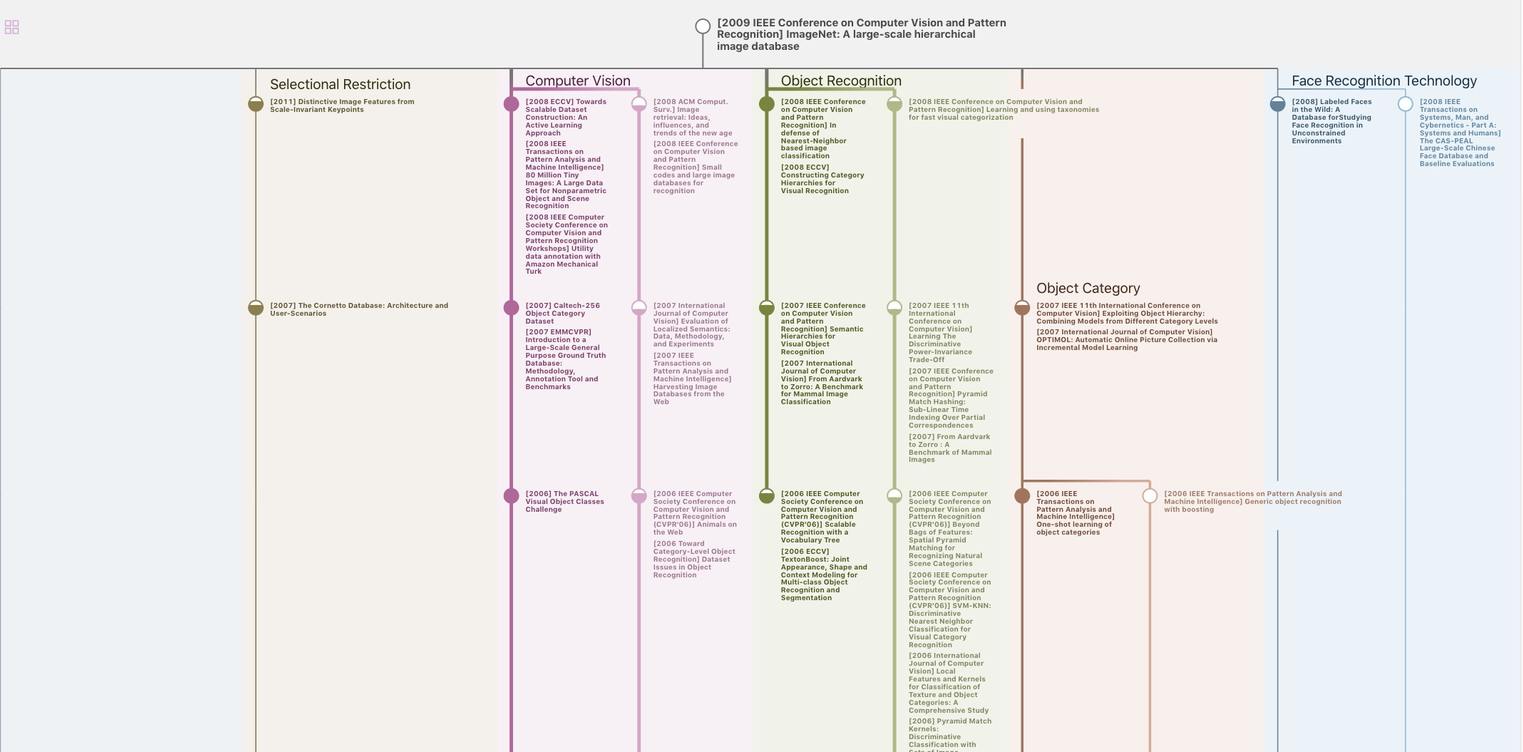
生成溯源树,研究论文发展脉络
Chat Paper
正在生成论文摘要