DDoS Attacks in Communication: Analysis and Mitigation of Unreliable Clients in Federated Learning
2024 IEEE 21st Consumer Communications & Networking Conference (CCNC)(2024)
摘要
In communication, network traffic prediction is the process of forecasting future network traffic based on historical data. This information can be used to improve network quality, optimize resource allocation, and prevent attacks. With the advent of Federated Learning (FL), the ability to harness insights from distributed data sources while preserving data privacy has revolutionized this field. However, the presence of malicious or unreliable data sources can impact the performance and reliability of the collaboratively trained Machine Learning (ML) models. One such situation is the presence of Distributed Denial of Service (DDoS) traffic data in FL training. In this work, the analysis and mitigation of clients with DDoS traffic data in FL for network traffic prediction are performed. The negative impact of such data on the performance of the global model is presented. Several mitigation techniques, such as client selection, data filtering, and model aggregation, are discussed. The results indicate that the Median aggregation technique is able to mitigate the effect of DDoS data. It is also suited to real-time scenarios as there is no need for client selection or data filtering, making it fast.
更多查看译文
AI 理解论文
溯源树
样例
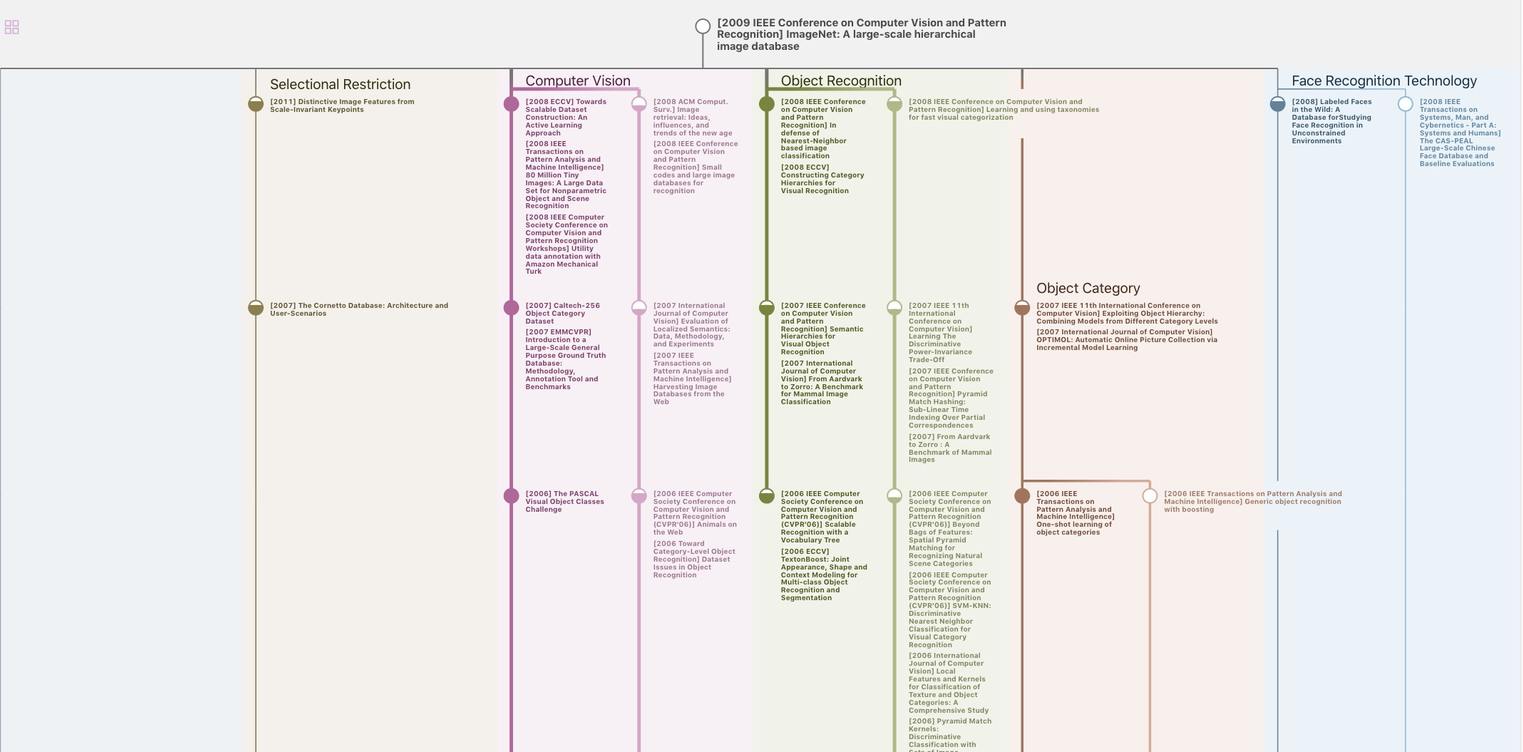
生成溯源树,研究论文发展脉络
Chat Paper
正在生成论文摘要