Leveraging High-Density EMG to Investigate Bipolar Electrode Placement for Gait Prediction Models
IEEE TRANSACTIONS ON HUMAN-MACHINE SYSTEMS(2024)
摘要
To control wearable robotic systems, it is critical to obtain a prediction of the user's motion intent with high accuracy. Surface electromyography (sEMG) recordings have often been used as inputs for these devices, however bipolar sEMG electrodes are highly sensitive to their location. Positional shifts of electrodes after training gait prediction models can therefore result in severe performance degradation. This study uses high-density sEMG (HD-sEMG) electrodes to simulate various bipolar electrode signals from four leg muscles during steady-state walking. The bipolar signals were ranked based on the consistency of the corresponding sEMG envelope's activity and timing across gait cycles. The locations were then compared by evaluating the performance of an offline temporal convolutional network (TCN) that mapped sEMG signals to knee angles. The results showed that electrode locations with consistent sEMG envelopes resulted in greater prediction accuracy compared to hand-aligned placements (p < 0.01). However, performance gains through this process were limited, and did not resolve the position shift issue. Instead of training a model for a single location, we showed that randomly sampling bipolar combinations across the HD-sEMG grid during training mitigated this effect. Models trained with this method generalized over all positions, and achieved 70% less prediction error than location specific models over the entire area of the grid. Therefore, the use of HD-sEMG grids to build training datasets could enable the development of models robust to spatial variations, and reduce the impact of muscle-specific electrode placement on accuracy.
更多查看译文
关键词
Electrodes,Muscles,Measurement,Predictive models,Knee,Electromyography,Standards,Artificial intelligence (AI) and machine learning,human-robot interaction,rehabilitation robotics,sensor networks,signal processing
AI 理解论文
溯源树
样例
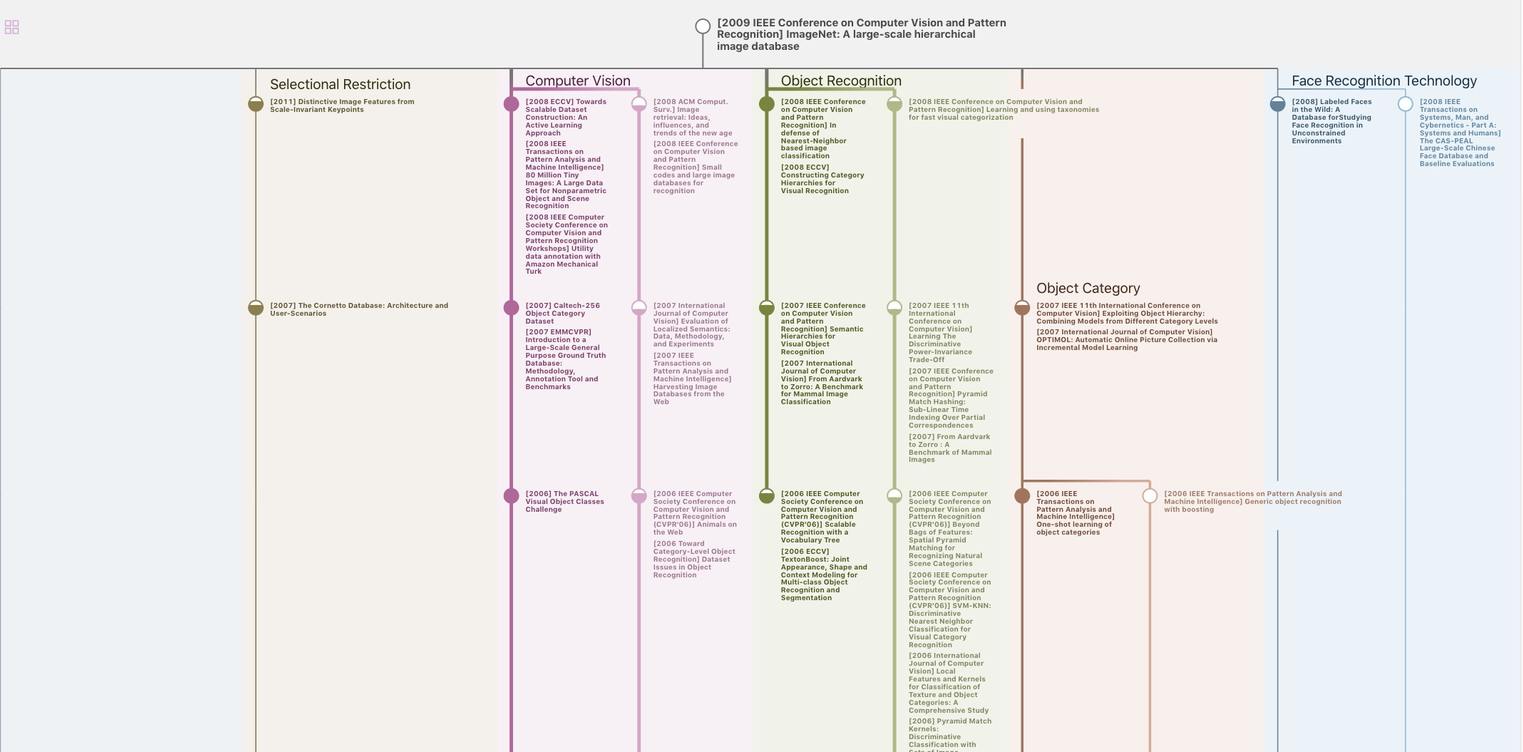
生成溯源树,研究论文发展脉络
Chat Paper
正在生成论文摘要