Capturing Detail Variations for Lightweight Neural Radiance Fields
ICASSP 2024 - 2024 IEEE International Conference on Acoustics, Speech and Signal Processing (ICASSP)(2024)
摘要
Neural Radiance Fields (NeRF) has recently overhauled novel view synthesis, but it requires extensive computations for training and captures variations in detail with difficulty. In this paper, we propose a novel framework, termed CD-TDRF, to mitigate these dilemmas. CD-TDRF factorizes a density voxel grid into a core tensor and three matrices via Tucker decomposition, reducing memory usage and accelerating training. To better capture variations in complex scenes, CD-TDRF uses a fully convolutional network to extract prior information from the training images. Moreover, three learnable appearance planes are constructed to preserve information about scene details, which enhances the rendering quality significantly. Our experimental results demonstrate that CD-TDRF has achieved competitive rendering quality on three popular datasets and speeds up training compared with traditional NeRF models.
更多查看译文
关键词
Novel view synthesis,neural radiance fields,neural rendering
AI 理解论文
溯源树
样例
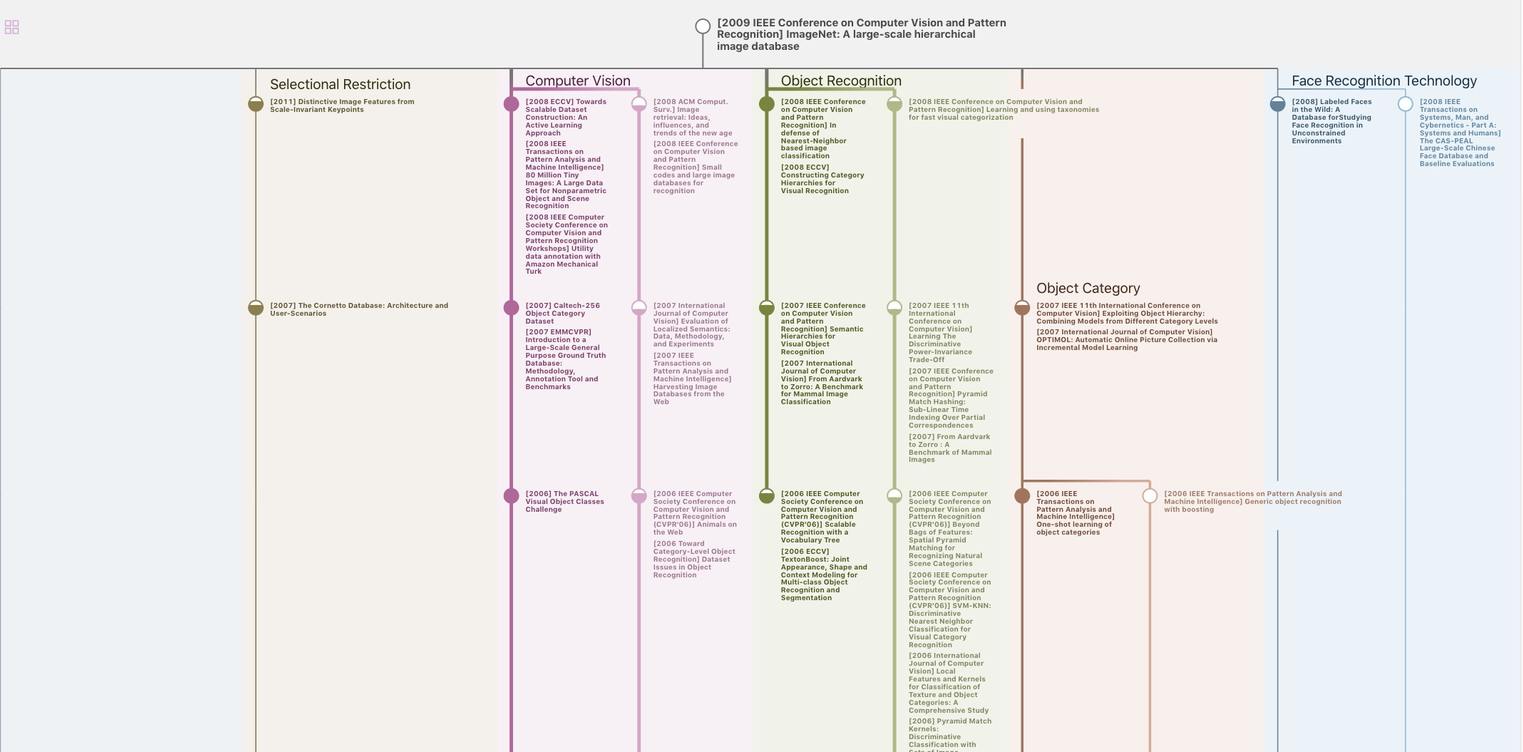
生成溯源树,研究论文发展脉络
Chat Paper
正在生成论文摘要