Multiple Imputation for Robust Cluster Analysis to Address Missingness in Medical Data
IEEE ACCESS(2024)
摘要
Cluster analysis has been applied to a wide range of problems as an exploratory tool to enhance knowledge discovery. Clustering aids disease subtyping, i.e. identifying homogeneous patient subgroups, in medical data. Missing data is a common problem in medical research and could bias clustering results if not properly handled. Yet, multiple imputation has been under-utilized to address missingness, when clustering medical data. Its limited integration in clustering of medical data, despite the known advantages and benefits of multiple imputation, could be attributed to many factors. This includes methodological complexity, difficulties in pooling results to obtain a consensus clustering, uncertainty regarding quality metrics, and a lack of accepted pipelines. A few studies have examined the feasibility of implementing multiple imputation for cluster analysis on simulated/small datasets. While these studies have begun to address how to pool imputed values and quantify uncertainty in clustering due to imputation, a need remains for a complete framework that integrates MI in the clustering of complex medical data and sophisticated cluster algorithms. We propose a cluster analysis framework that mitigates bias and addresses these limitations. It includes methods to pool multiple imputed datasets, create a consensus cluster solution by ensemble methods, and select an optimal number of clusters based on validity indices. It also estimates uncertainty about cluster membership attributable to the imputation and identifies features that characterize the derived clusters. The utility of this framework is illustrated by its application to a traumatic brain injury dataset with missing data. Our analysis revealed six multifaceted clusters that differed with respect to Glasgow Coma Score (GCS), mechanism of injury, sociodemographics, vitals, lab values, and radiological presentation. The most severe cluster consisted of single, relatively young patients injured by motor accident, with higher GCS severity scores. Comparative analysis with the miclust R package, along with statistical validation of cluster characterization, demonstrates its robust performance.
更多查看译文
关键词
Multiple data imputation,clustering,ensemble learning,canonical discriminant analysis,mixture models,traumatic brain injury,missingness
AI 理解论文
溯源树
样例
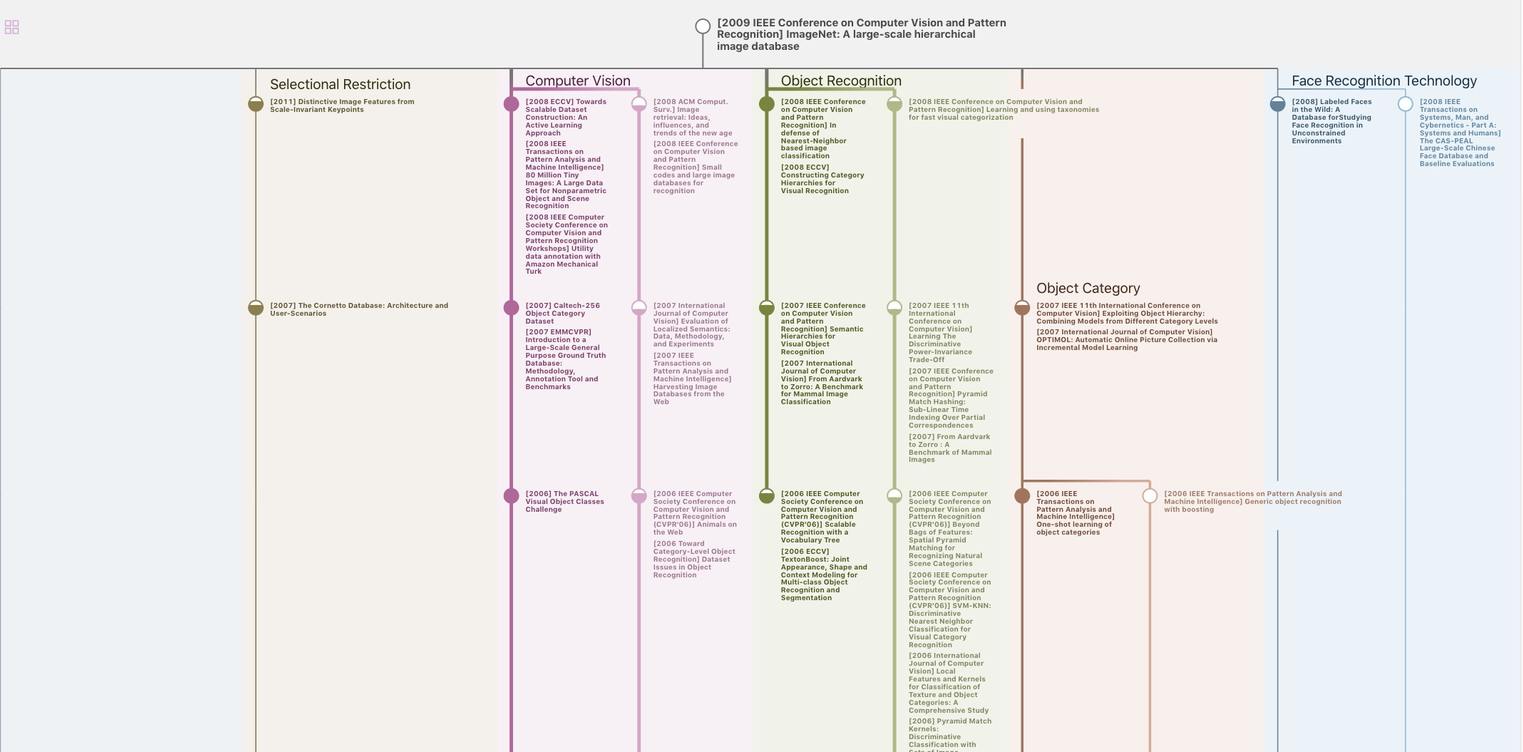
生成溯源树,研究论文发展脉络
Chat Paper
正在生成论文摘要