Active Learning for Sound Event Classification Using Bayesian Neural Networks with Gaussian Variational Posterior
ICASSP 2024 - 2024 IEEE International Conference on Acoustics, Speech and Signal Processing (ICASSP)(2024)
摘要
Manual annotation of audio material is cumbersome. Active learning aims at minimizing the annotation effort by iteratively selecting an acquisition batch of unlabeled data, asking a human to annotate the selected data and re-training a classifier until an annotation budget is depleted. In this paper we propose the Gaussian-dense active learning (GDAL) algorithm to train a sound event classifier. The classifier is a Bayesian neural network where the weights are normally distributed. This is in contrast to conventional neural networks where weights are not distributed, but have assigned values. The Bayesian nature of the classifier empowers GDAL to select acquisition batches from a set of unlabeled audio clips based on their estimated informativeness. Evaluation results on the UrbanSound8k dataset show that GDAL outperforms a state-of-the-art algorithm based on medoid active learning for all considered annotation budgets and an algorithm based on dropout active learning for sufficiently large annotation budgets.
更多查看译文
关键词
sound event classification,active learning,Bayesian neural networks,PANN embeddings
AI 理解论文
溯源树
样例
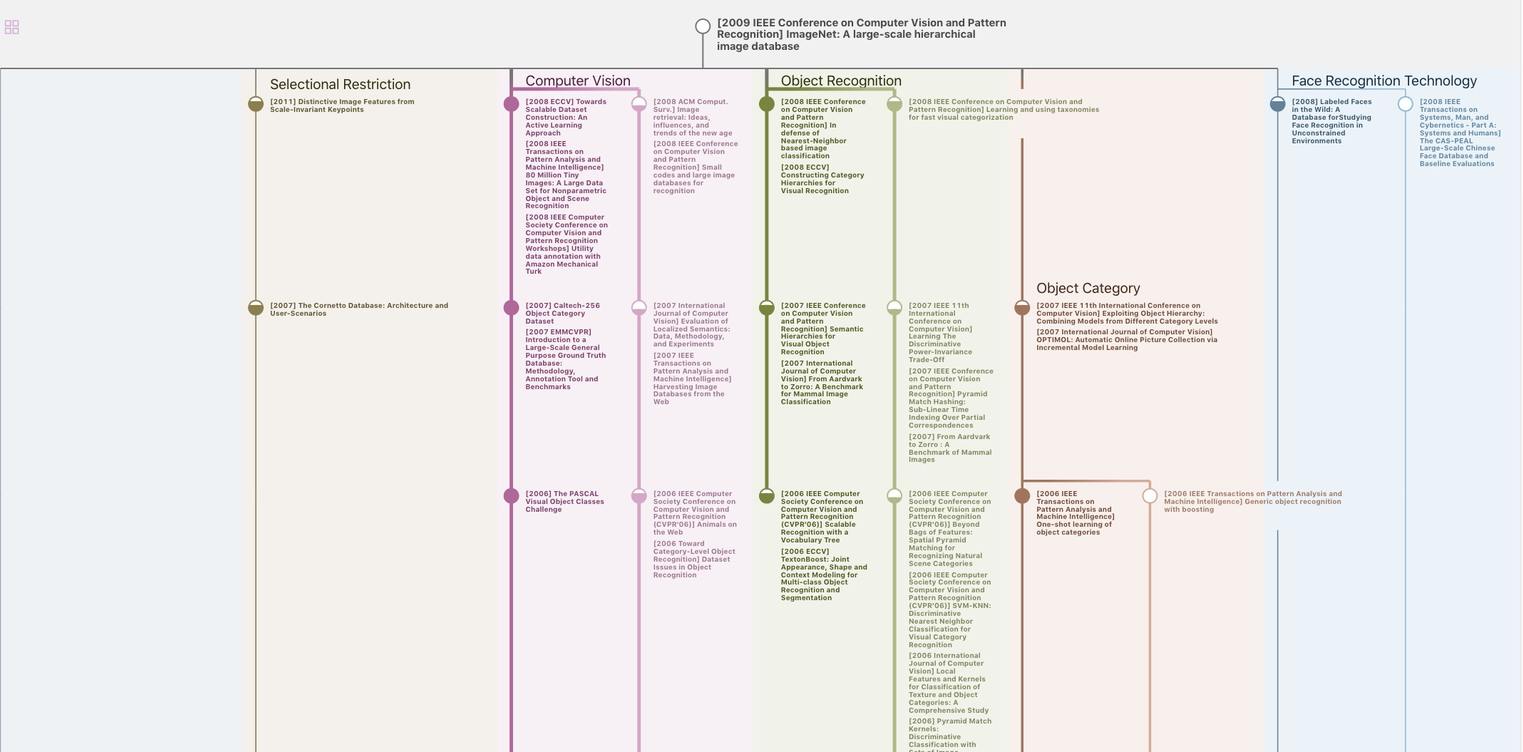
生成溯源树,研究论文发展脉络
Chat Paper
正在生成论文摘要