YOLOv8-QSD: An Improved Small Object Detection Algorithm for Autonomous Vehicles Based on YOLOv8
IEEE TRANSACTIONS ON INSTRUMENTATION AND MEASUREMENT(2024)
摘要
As self-driving vehicles become more prevalent, the speed and accuracy of detecting surrounding objects through onboard sensing technology have become increasingly important. The YOLOv8-QSD network is a novel anchor-free driving scene detection network that builds on YOLOv8 and ensures detection accuracy while maintaining efficiency. The network's backbone employs structural reparameterization techniques to transform the diverse branch block (DBB)-based model. To accurately detect small objects, it integrates features of different scales and implements a bidirectional feature pyramid network (BiFPN)-based feature pyramid after the backbone. To address the challenge of long-range detection in driving scenarios, a query-based model with a new pipeline structure is introduced. The test results demonstrate that this algorithm outperforms YOLOv8 on the large-scale small object detection dataset (SODA-A) in terms of both speed and accuracy. With an accuracy rate of 64.5% and reduced computational requirements of 7.1 GFLOPs, it satisfies the speed, precision, and cost-effectiveness requirements for commercial vehicles in high-speed road driving scenarios.
更多查看译文
关键词
2-D object detection,autonomous driving,highway traffic,image recognition
AI 理解论文
溯源树
样例
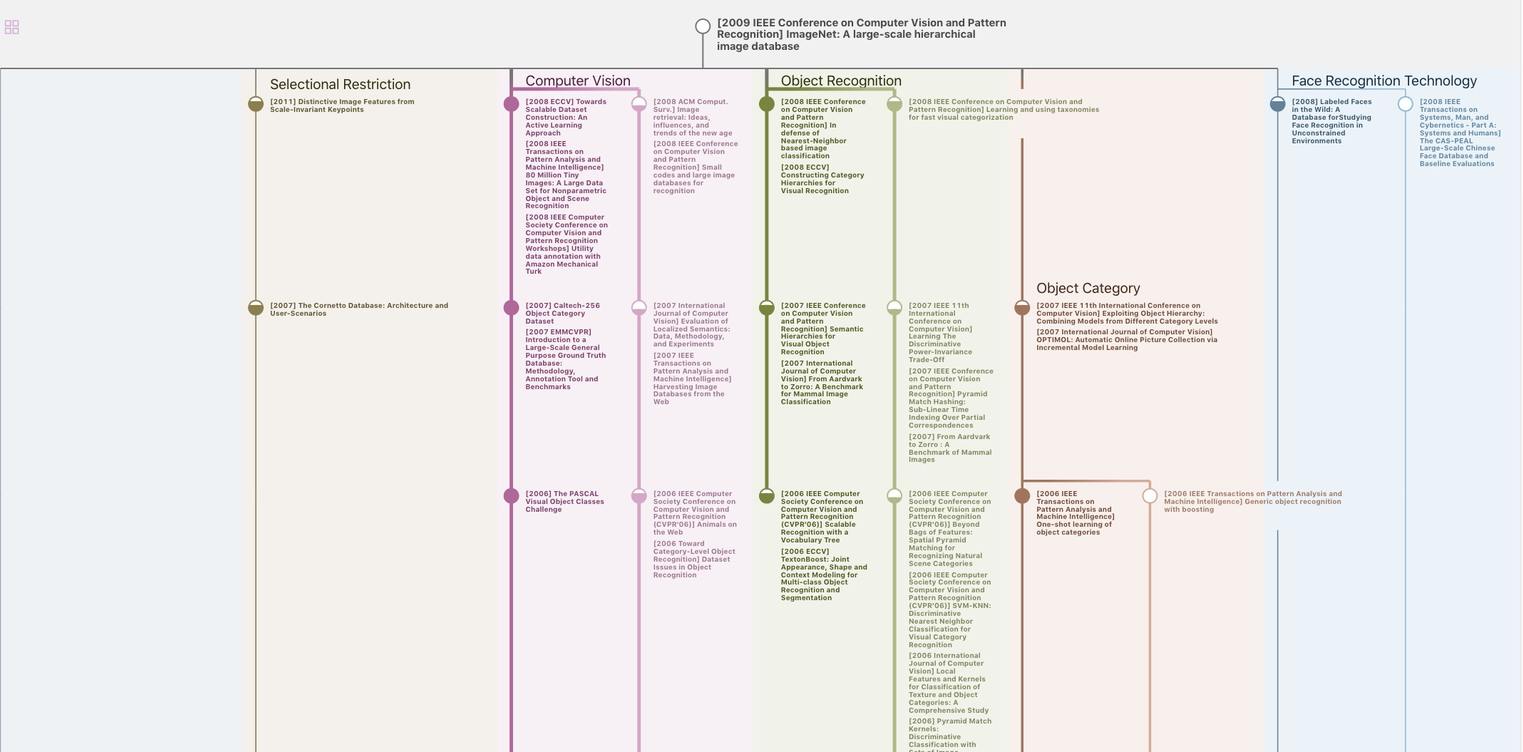
生成溯源树,研究论文发展脉络
Chat Paper
正在生成论文摘要