Self-Iterated Extracting Wavelet Transform and Its Application to Fault Diagnosis of Rotating Machinery
IEEE TRANSACTIONS ON INSTRUMENTATION AND MEASUREMENT(2024)
摘要
Time-frequency (TF) analysis (TFA) is a valuable tool for capturing nonstationary features in time-varying signals. However, for strongly amplitude-modulated and frequency-modulated (AM-FM) signals, how to achieve accurate characterization of time-varying features and how to obtain highly concentrated TF representations (TFRs) are the primary focuses. To address these issues, a novel method called the self-iterated extracting wavelet transform (SIEWT) is proposed in this article. In the proposed method, an iterative precise and high-resolution method for calculating the instantaneous frequency (IF) of a signal is presented. The SIEWT is based on the synchrosqueezing wavelet transform (SWT) and constructs an iterative IF estimator. On this basis, a self-iterated procedure is designed by the Renyi entropy to obtain a more accurate frequency estimation operator, meanwhile reducing the computational cost. After that, the acquired IF estimate is inserted into the extraction operator to further minimize energy dispersion and generate TFR with high TF resolution. The SIEWT also retains the signal reconstruction ability, its accurate estimation of IF plays a critical role in achieving precise signal reconstruction. The effectiveness of the proposed method is thoroughly confirmed by simulation and analysis of real signals, with comparisons made against classical TF analysis methods.
更多查看译文
关键词
Fault diagnosis,frequency estimation,self-iterated extracting wavelet transform (SIEWT),time-frequency analysis (TFA)
AI 理解论文
溯源树
样例
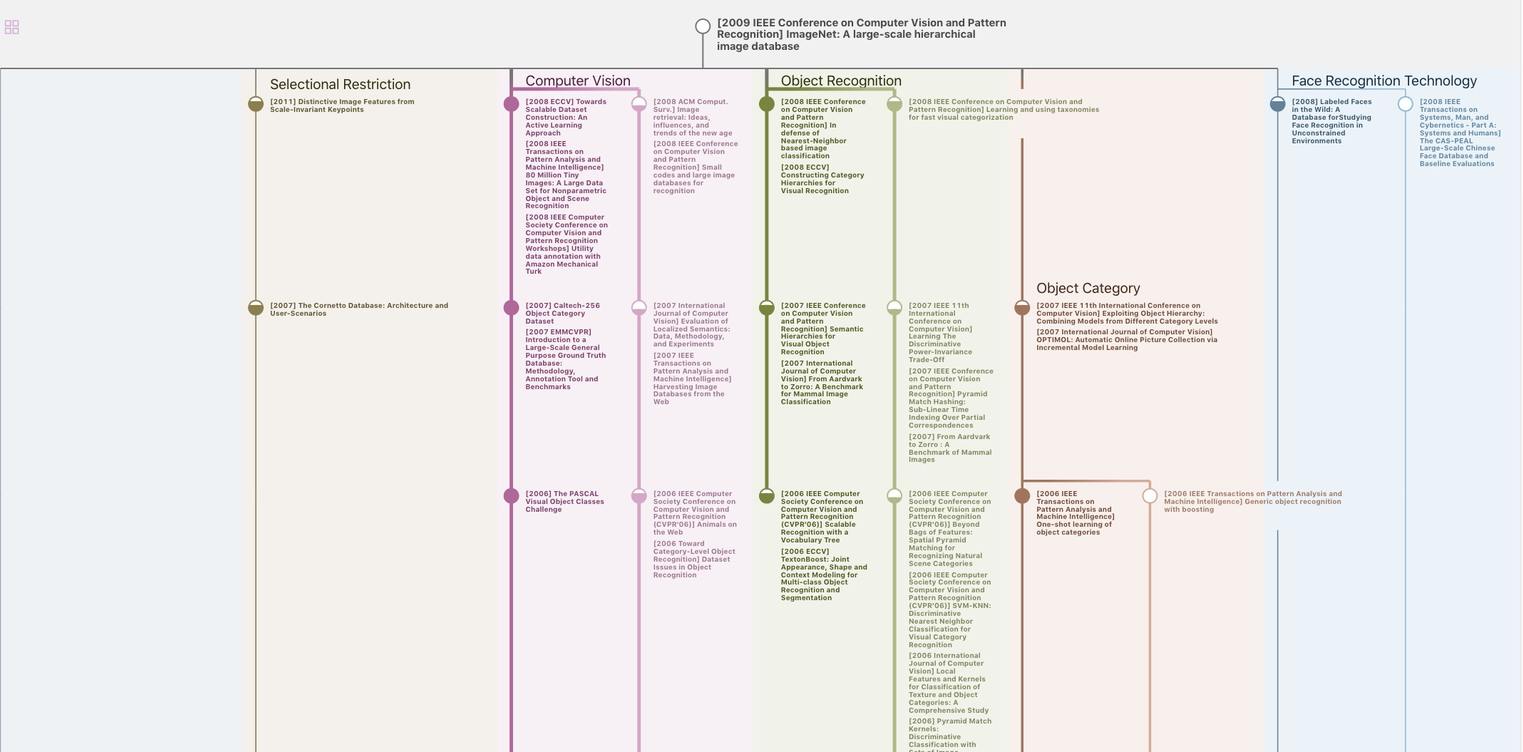
生成溯源树,研究论文发展脉络
Chat Paper
正在生成论文摘要