Audio-Journey: Open Domain Latent Diffusion Based Text-To-Audio Generation
ICASSP 2024 - 2024 IEEE International Conference on Acoustics, Speech and Signal Processing (ICASSP)(2024)
摘要
Despite recent progress, machine learning (ML) models for open-domain audio generation need to catch up to generative models for image, text, speech, and music. The lack of massive open-domain audio datasets is the main reason for this performance gap; we overcome this challenge through a novel data augmentation approach. We leverage state-of-the-art (SOTA) Large Language Models (LLMs) to enrich captions in the weakly-labeled audio dataset. We then use a SOTA video-captioning model to generate captions for the videos from which the audio data originated, and we again use LLMs to merge the audio and video captions to form a rich, large-scale dataset. We experimentally evaluate the quality of our audio-visual captions, showing a 12.5% gain in semantic score over baselines. Using our augmented dataset, we train a Latent Diffusion Model to generate in an encodec encoding latent space. Our model is novel in the current SOTA audio generation landscape due to our generation space, text encoder, noise schedule, and attention mechanism. Together, these innovations provide competitive open-domain audio generation. The samples, models, and implementation will be at https://audiojourney.github.io.
更多查看译文
关键词
Deep Learning,Open Domain Audio Generation,Audio-Visual Training,Large Language Models
AI 理解论文
溯源树
样例
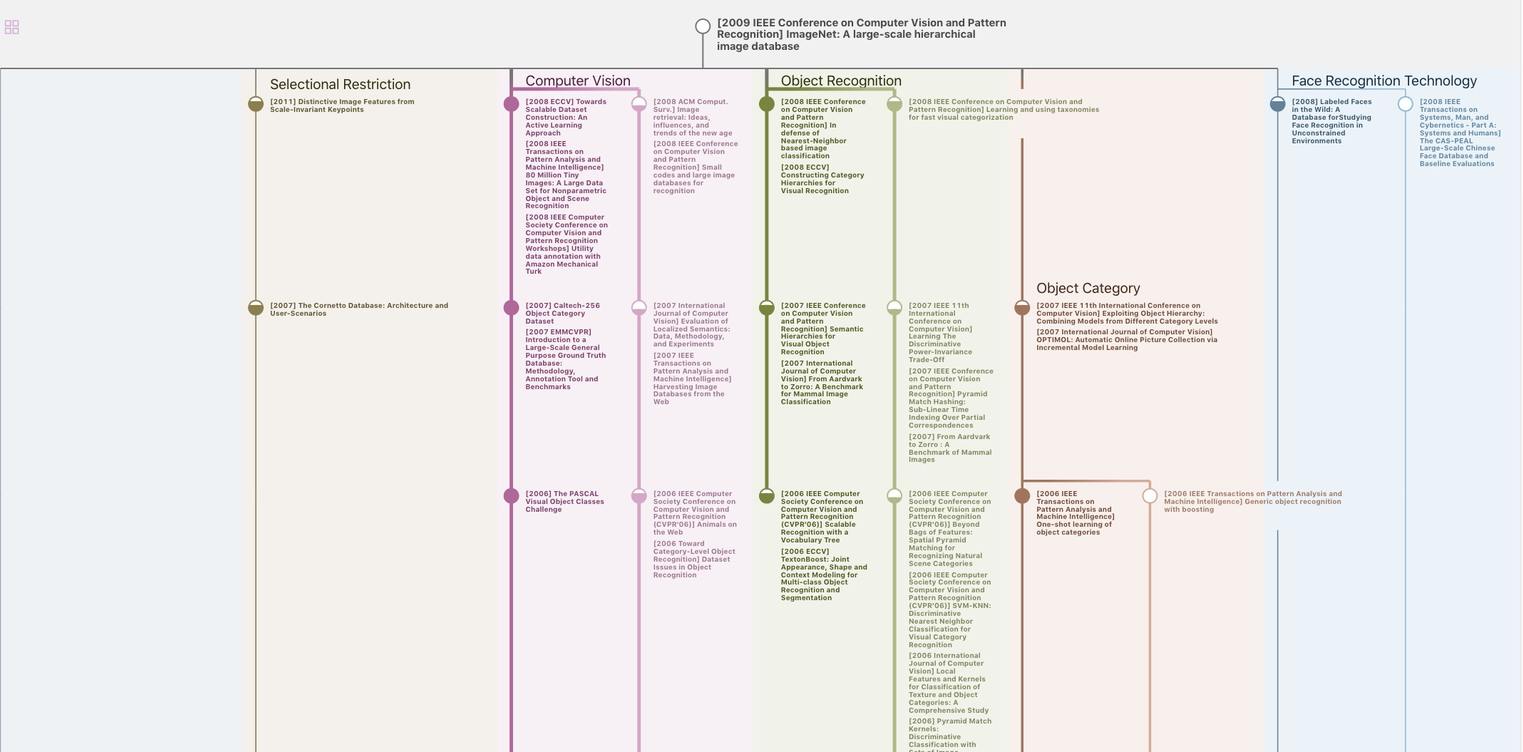
生成溯源树,研究论文发展脉络
Chat Paper
正在生成论文摘要