Mitigating Optimization Conflict in Domain Adversarial Neural Network via Uncertainty-Aware
ICASSP 2024 - 2024 IEEE International Conference on Acoustics, Speech and Signal Processing (ICASSP)(2024)
摘要
In prior studies, domain adversarial neural networks (DANNs) are used to align image-level features regardless of foreground and background. However, the conventional discriminator in DANNs may leads the feature extractor to disregard cross-domain features rather than aligning them. This phenomenon negatively impact classifier performance. We propose a novel loss reweighting technique that mitigates the optimization conflict between discriminator and classifier. The classification loss is reweighted based on the prediction uncertainty that is measured by two different bottleneck layers. This reweighting approach guides the model in determining which features should be activated or aligned, resulting in significantly improved adaptation performance. Additionally, we introduce a novel construction method of bottleneck layer based on pseudo label of target domain and differentiable architecture search to support our approach. Our method is rigorously evaluated across multiple benchmark datasets and outperforms state-of-the-art (SOTA) methods.
更多查看译文
关键词
Domain adversarial network,prediction uncertainty,unsupervised domain adaptation
AI 理解论文
溯源树
样例
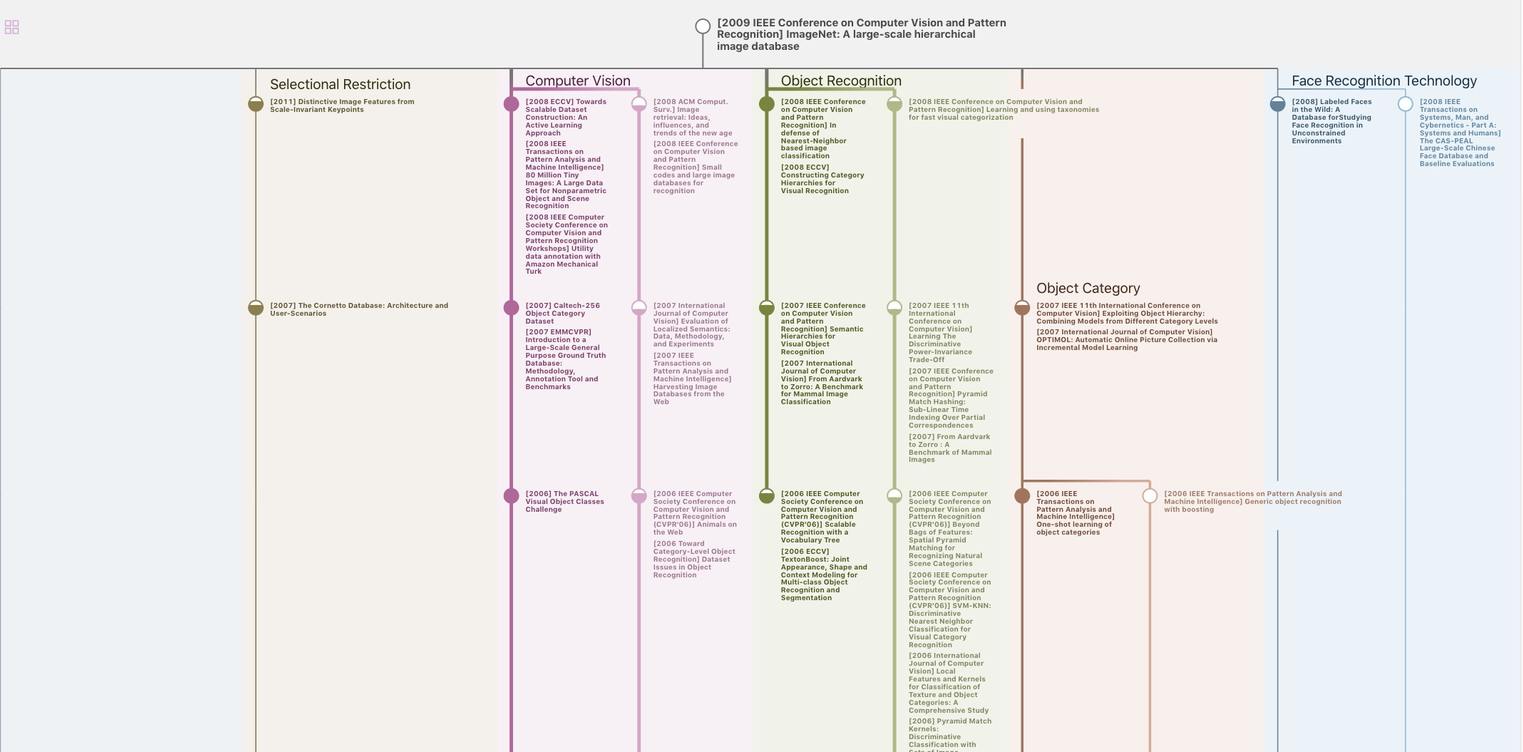
生成溯源树,研究论文发展脉络
Chat Paper
正在生成论文摘要