Zero-Shot Attribute Consistent Model for Bearing Fault Diagnosis Under Unknown Domain
IEEE TRANSACTIONS ON INSTRUMENTATION AND MEASUREMENT(2024)
摘要
Existing bearing fault diagnosis methods based on deep learning typically rely on a large amount of labeled data for training. However, acquisition of a large amount of labeled target data in practical engineering is challenging. A zero-shot attribute consistent (ZSAC) model is proposed in this study to address this issue. This diagnostic model only requires data from the known domain and does not require any data from the unknown domain during training. A fine-grained attribute description matrix is first constructed according to the various single fault types and fault impulse characteristics of bearing in this study, and it can be used to diagnose the faults in the unknown domain. A wide hybrid dilated convolutional neural network (WHDCNN) is designed for feature extraction, which can obtain more information with fewer parameters and provide more effective features for attribute classification than the existing convolutional neural networks (CNNs). An attribute consistency loss is proposed to bridge the relationship between attributes and features in the known domain. This approach can effectively avoid attribute misclassification and improve diagnostic accuracy. The performance of ZSAC model is examined using two bearing datasets. Test results show that the proposed ZSAC model can effectively diagnose the single and compound faults of bearings under the unknown working condition and have advantages over other typical zero-shot learning and transfer learning methods.
更多查看译文
关键词
Fault diagnosis,Feature extraction,Training,Zero-shot learning,Transfer learning,Task analysis,Convolution,neural networks,rolling bearing,transfer learning,zero-shot learning
AI 理解论文
溯源树
样例
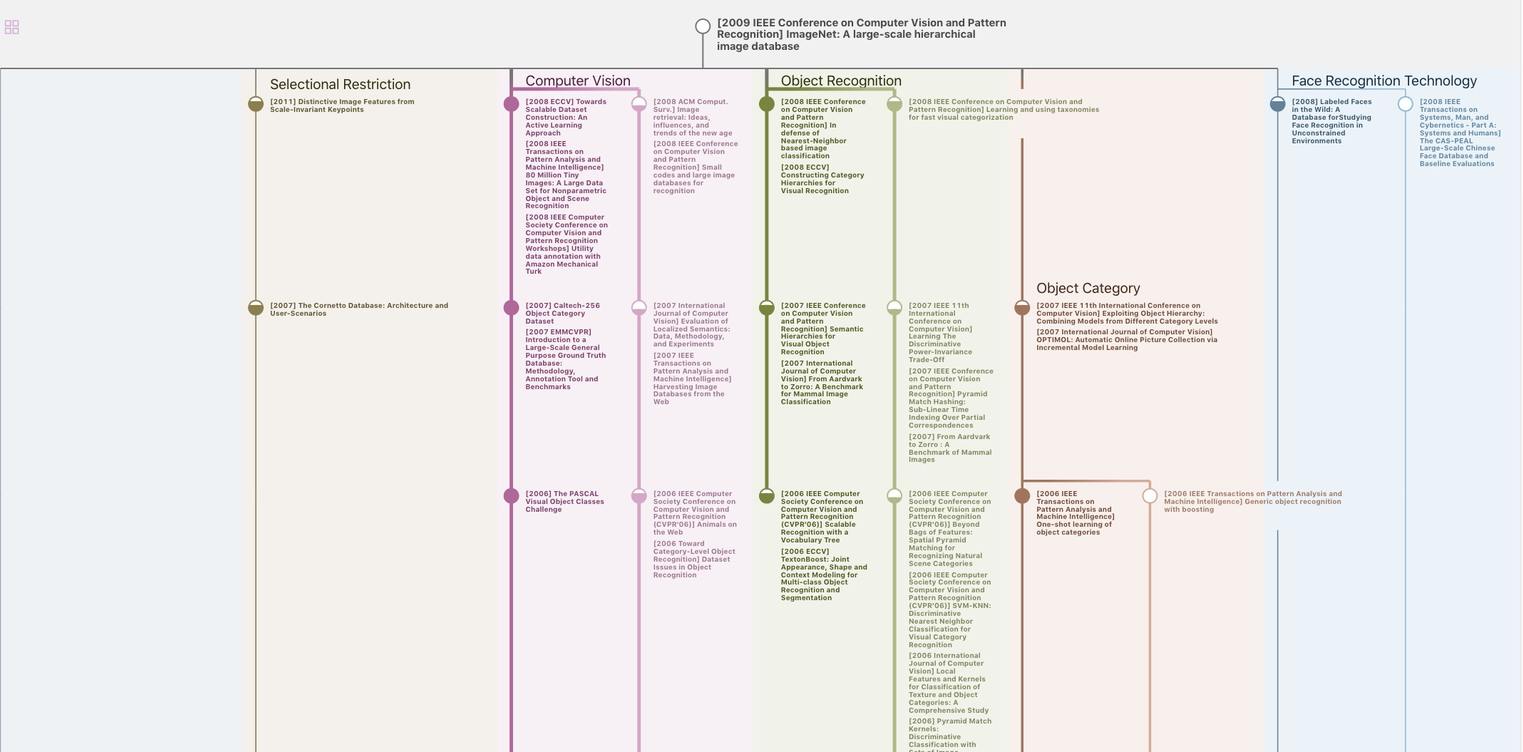
生成溯源树,研究论文发展脉络
Chat Paper
正在生成论文摘要