Elevating Visual Prompting in Transfer Learning Via Pruned Model Ensembles: No Retrain, No Pain
ICASSP 2024 - 2024 IEEE International Conference on Acoustics, Speech and Signal Processing (ICASSP)(2024)
摘要
Visual Prompting (VP) has been gaining traction in the deep learning community, yet its performance often falls short when compared to traditional finetuning methods in transfer learning. In this study, we present a novel approach to enhance VP by leveraging the insights from the lottery ticket hypothesis. In contrast to the prevailing practice of retraining pruned models, our discovery reveals that merely pruning an initially pretrained model, without any subsequent retraining, can deliver a VP on par with its dense counterpart. Building upon this valuable insight, we present an ensemble strategy that leverages VP on pruned backbone models at different sparsity levels, aiming to enhance VP accuracy. To assess the effectiveness of our approach, we conduct extensive experiments across 4 model architectures and 12 diverse datasets. Our results consistently illustrate the potency of pruning ensembles in augmenting VP performance, with accuracy enhancements spanning from 1.3% to an impressive 12.96%. This not only narrows the gap with traditional finetuning methodologies but also establishes a new benchmark for VP techniques.
更多查看译文
关键词
Visual prompting,model pruning,sparsity,model ensemble
AI 理解论文
溯源树
样例
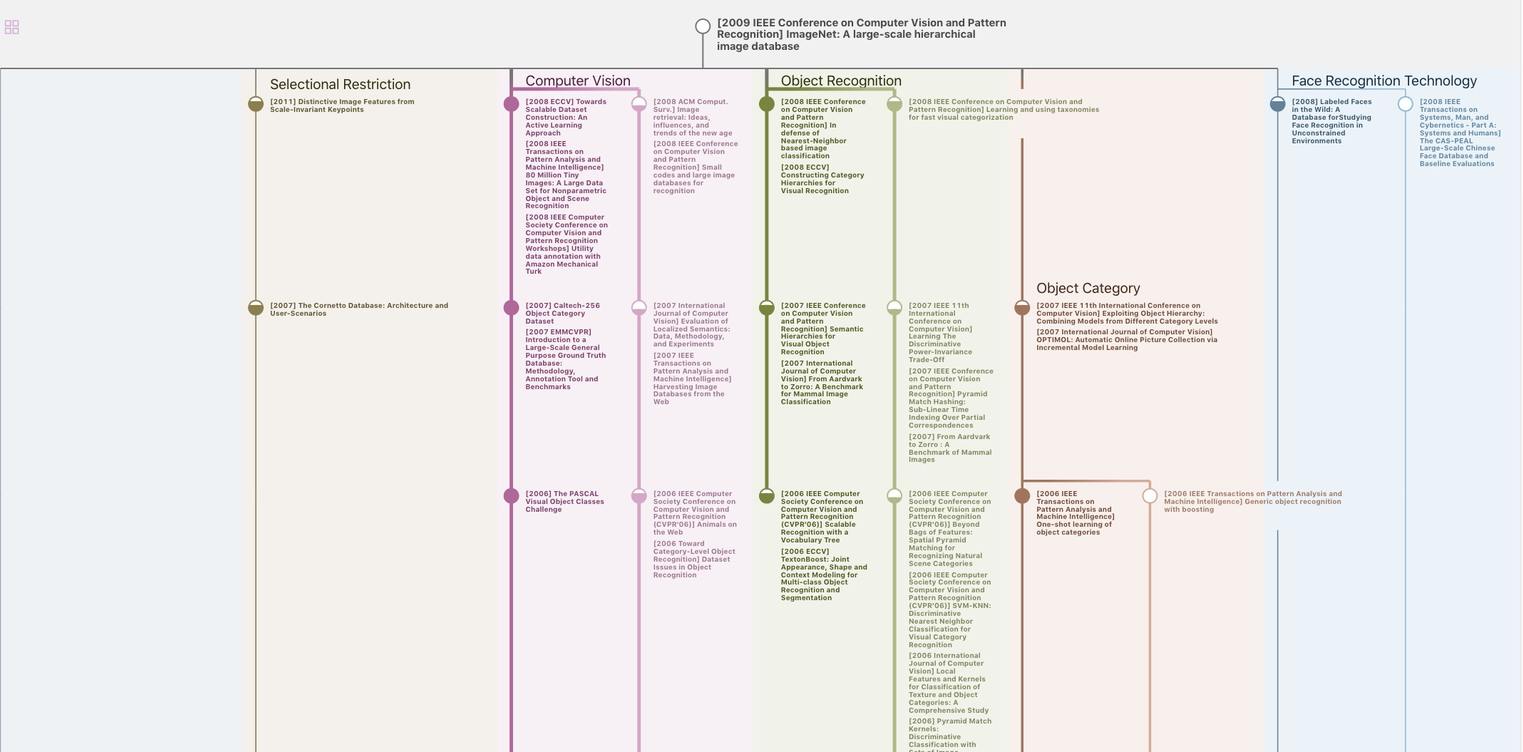
生成溯源树,研究论文发展脉络
Chat Paper
正在生成论文摘要