Localization in Sensor Networks Using Distributed Low-Rank Matrix Completion
ICASSP 2024 - 2024 IEEE International Conference on Acoustics, Speech and Signal Processing (ICASSP)(2024)
摘要
Localization in terrestrial and non-terrestrial networks plays an important role in various applications, such as autonomous driving, robotics, and unmanned aerial vehicles. Although the relative distances between neighboring devices can be directly detected by embedded sensors, the relative distances between non-neighboring devices are usually not available, which results in a sparse version of the Euclidean Distance Matrix (EDM). Since the complete EDM is low-rank and the information of the relative distances is distributed over the network, we consider a distributed localization approach based on the low-rank matrix completion using the singular value thresholding algorithm. The proposed approach is carried out in a distributed manner as the singular values and singular vectors are estimated by the distributed eigenvalue decomposition. It avoids gathering all information in a central server and only requires direct communication and distance estimation between neighboring sensors. Hence, the distance information acquired in each sensor and the coordinates of the sensors are kept private to the sensor. The proposed distributed low-rank matrix completion approach is also applicable, e.g., in the distributed recommendation system.
更多查看译文
关键词
Distributed localization,low-rank matrix completion,distributed eigenvalue decomposition
AI 理解论文
溯源树
样例
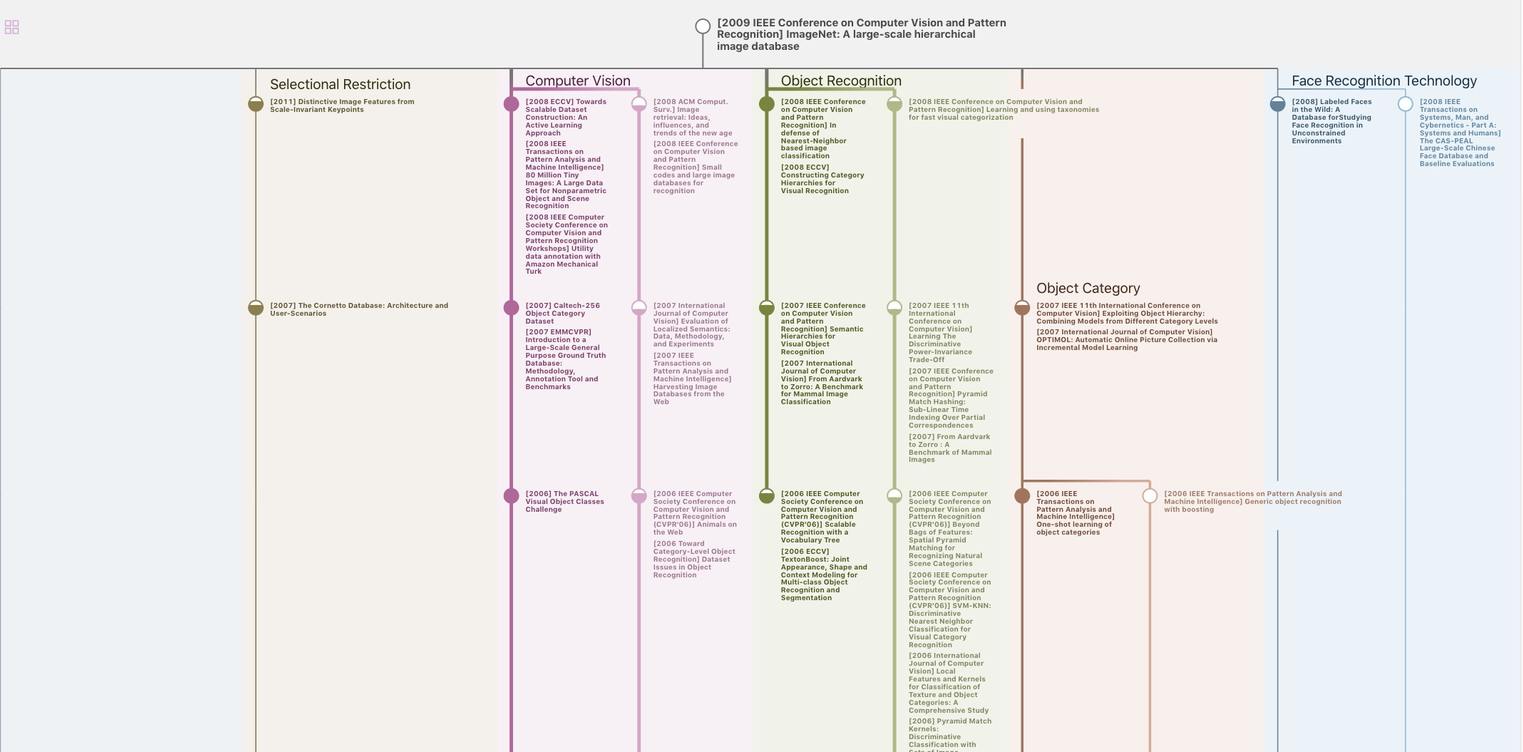
生成溯源树,研究论文发展脉络
Chat Paper
正在生成论文摘要