Offline Reinforcement Learning with Generative Adversarial Networks and Uncertainty Estimation
ICASSP 2024 - 2024 IEEE International Conference on Acoustics, Speech and Signal Processing (ICASSP)(2024)
摘要
In recent years, offline reinforcement learning has attracted considerable attention in artificial intelligence. By generating a static dataset through a behavior policy, it is unable to engage in online interactions with the environment. However, this inevitably leads to states or actions undergoing inherent distribution shifts. To address the above concerns, offline reinforcement learning with generative adversarial networks and uncertainty estimation (GANUE) is proposed. This approach parameterizes the conditional generative adversarial networks and avoids the over-constraint problem that would be introduced when using a distance metric. Estimating the Q-value through ensemble uncertainty not only relaxes the policy constraint strength but also enhances the out-of-distribution generalization ability. The experimental results show that GANUE significantly performs better on the Maze2D and Adroit tasks than multiple baselines. In addition, we perform sensitivity analysis experiments for the parameters of the perturbation model.
更多查看译文
关键词
Offline reinforcement learning,Distribution shifts,Policy constraint,Generative adversarial networks,Uncertainty estimation
AI 理解论文
溯源树
样例
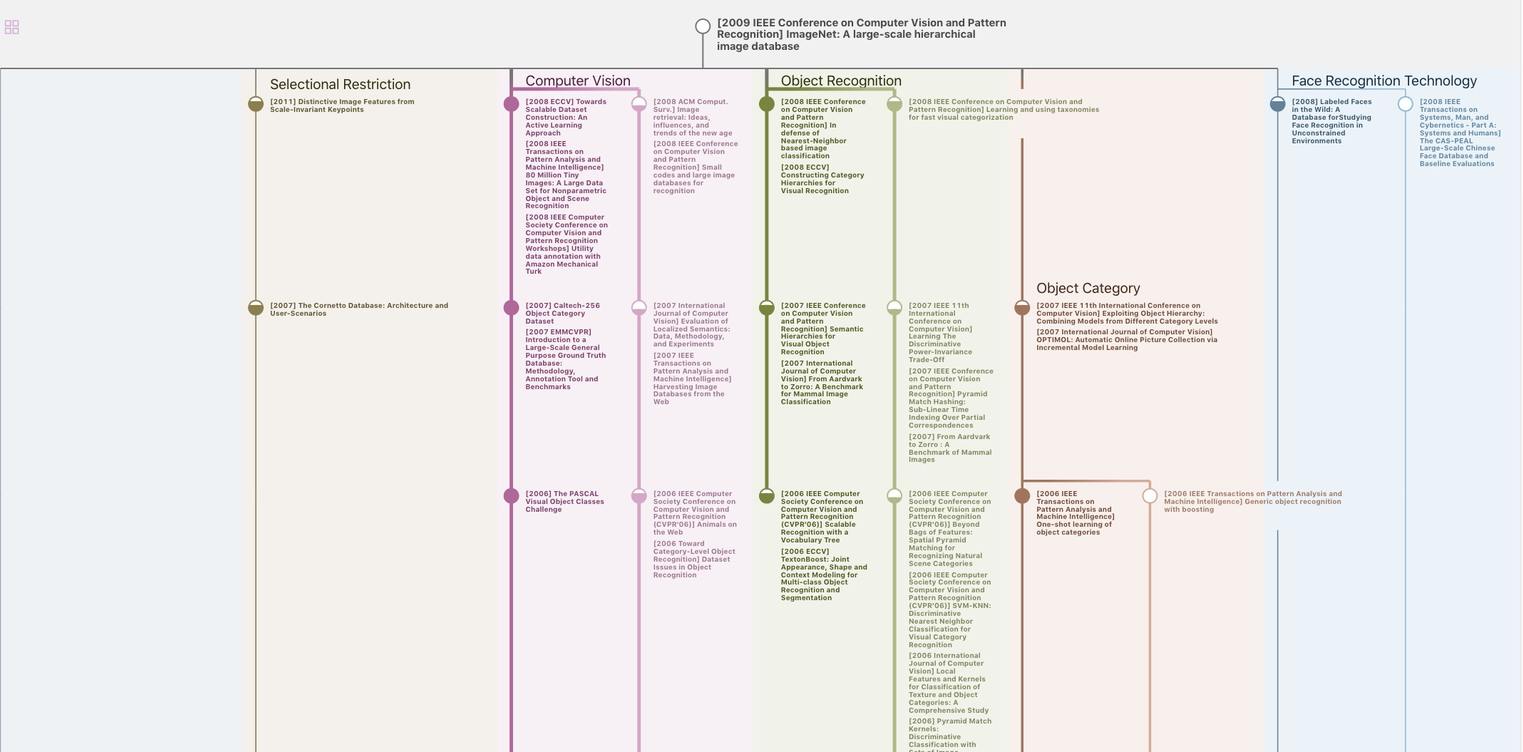
生成溯源树,研究论文发展脉络
Chat Paper
正在生成论文摘要