Geometry-Enhanced Attentive Multi-View Stereo for Challenging Matching Scenarios
IEEE Transactions on Circuits and Systems for Video Technology(2024)
摘要
Deep networks have made remarkable progress in Multi-View Stereo (MVS) task in recent years. However, the problem of finding accurate correspondences across different views under ill-posed matching situations remains unresolved and crucial. To address this issue, this paper proposes a Geometry-enhanced Attentive Multi-View Stereo (GA-MVS) network, which can access multi-view consistent feature representation and achieve accurate depth estimation in challenging situations. Specifically, we propose a geometry-enhanced feature extractor to explore illumination-invariant geometric features and incorporate them with common texture features to improve matching accuracy when dealing with view-dependent photometric effects, such as shadow and specularity. Then, we design a novel attentive learning framework to explore per-pixel adaptive supervision, effectively improving the depth estimation performance of textureless regions. The experimental results on the DTU and Tanks & Temples benchmarks demonstrate that our method achieves state-of-the-art results compared to other advanced MVS models.
更多查看译文
关键词
Multi-View Stereo,3D Reconstruction,Depth Estimation,Geometric features,Deep Learning
AI 理解论文
溯源树
样例
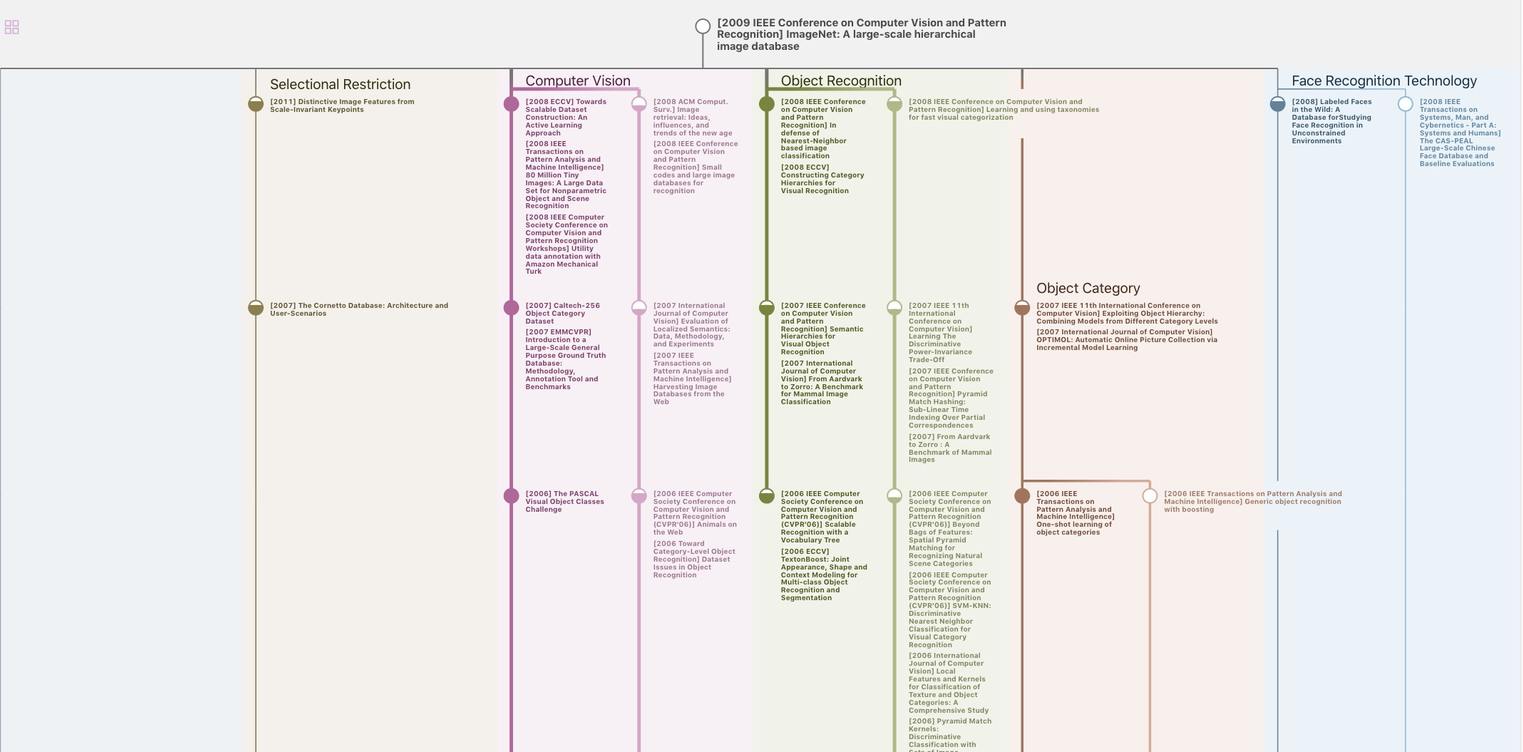
生成溯源树,研究论文发展脉络
Chat Paper
正在生成论文摘要