Real-time surface roughness estimation and automatic regrinding of ground workpieces using a data-driven model and grinding force inputs
The International Journal of Advanced Manufacturing Technology(2024)
摘要
This study reports a methodology for predicting surface roughness using data-driven models with grinding force as the input data. Prior to the model training process, the critical grinding parameters for brass material were selected and optimized using the Taguchi method. The experimental grinding force data were then collected and preprocessed into three features: the raw feature as the baseline feature, the statistical feature, and the fast Fourier transform (FFT) feature. The data were imported into a linear regression model as the baseline model and a deep neural network (DNN) model as the proposed strategy. The widely used surface roughness (Ra) of the ground workpiece was experimentally measured and served as the performance index. The model’s performance was evaluated based on the mean absolute percentage error (MAPE) between the predicted and measured Ra values. The validation of the Ra prediction revealed that, among all test combinations, the DNN model with four hidden layers and the FFT feature as the input had the best performance of surface roughness prediction, with a MAPE of 3.17
更多查看译文
关键词
Grind,Contact force,Surface roughness,Fast Fourier transform (FFT),Linear regression,Deep neural network (DNN)
AI 理解论文
溯源树
样例
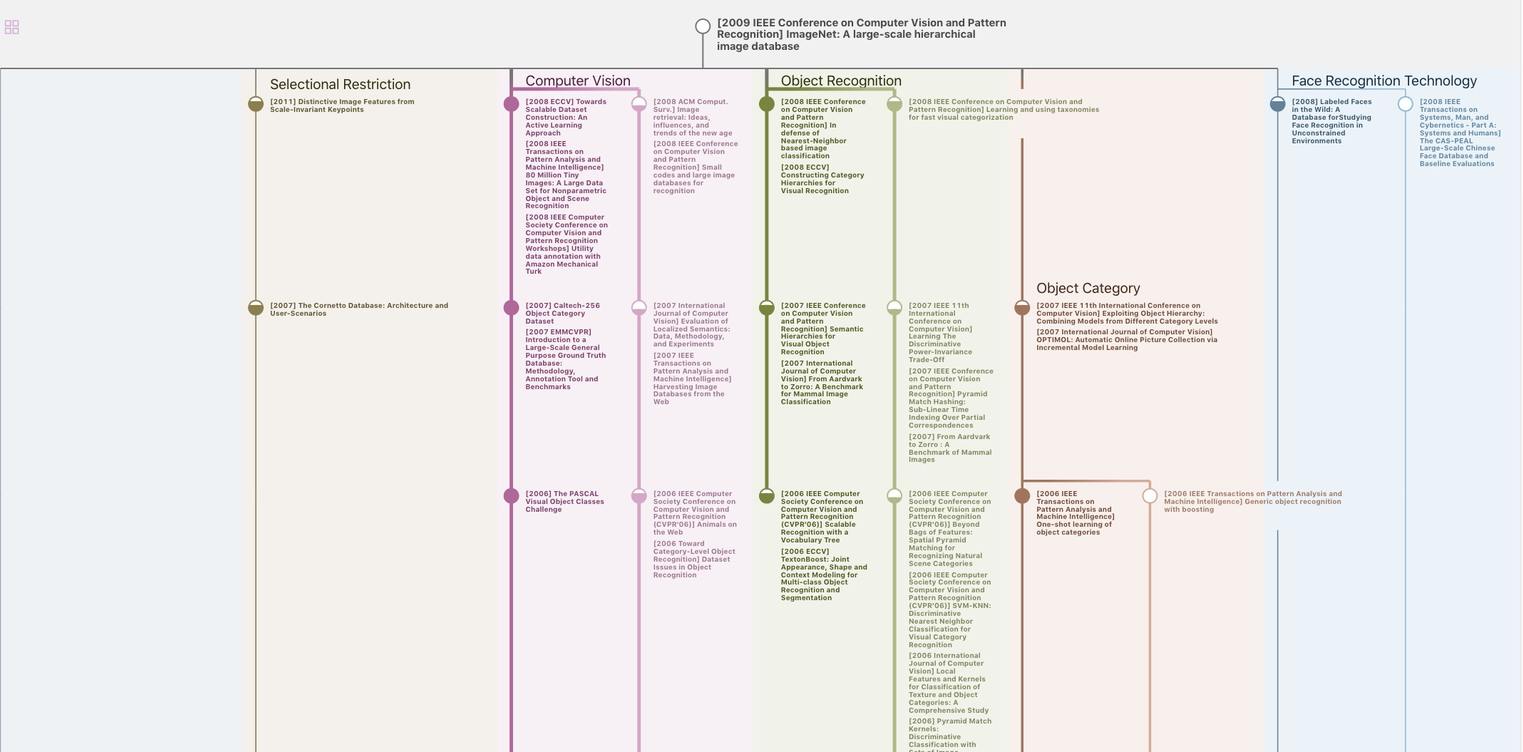
生成溯源树,研究论文发展脉络
Chat Paper
正在生成论文摘要