A robust machine learning-based framework for handling time-consuming constraints for bi-objective optimization of nonlinear steel structures
Structures(2024)
摘要
In structural design, multi-objective optimization of nonlinear steel structures simultaneously considers various design (often conflicting) purposes during the stage of construction to the operation of projects. Such optimization generally requires excessive computational efforts for the evaluation of time-consuming constraints. To overcome this drawback, this paper presents an efficient machine learning-based (ML) method to perform bi-objective optimization of nonlinear steel structures describing two conflicting objectives, namely minimization of structural weight and nodal displacements, simultaneously. The proposed method combines a nondominated sorting genetic algorithm-II (NSGA2) with an extreme gradient boosting (XGBoost) surrogate-assisted model. Furthermore, a multi-objective comparison technique (MulCT) is implemented, where a safety parameter t is introduced to reduce errors in the predictive ML model. To demonstrate the efficiency of the proposed method, two robust design examples, including a planar 10-bar truss and 47-bar transmission tower structures, are provided. In comparison with various ML algorithms, numerical results illustrate that the XGBoost model is superior in performance and requires significantly less training time. The MulCT technique enhances computational efficiency, leading to a 20% reduction in computing resources as when it was implemented with NSGA2. The proposed method by integrating both MulCT and XGBoost models with NSGA2 can save more than 50% of computational efforts, while maintaining good accuracy, as can be achieved by NSGA2.
更多查看译文
关键词
Multi-objective optimization,Inelastic analysis,Surrogate-assisted model,Metaheuristic,NSGA2
AI 理解论文
溯源树
样例
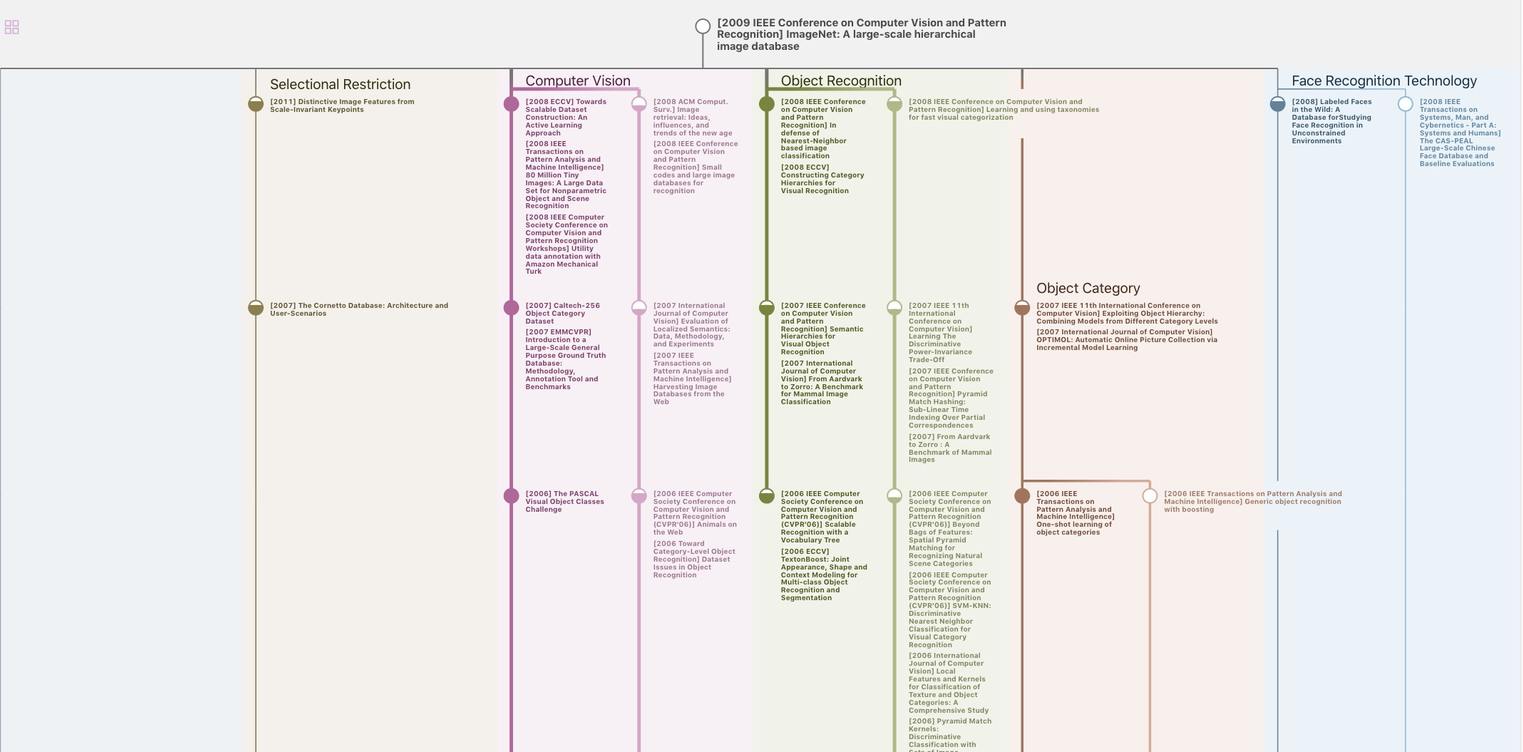
生成溯源树,研究论文发展脉络
Chat Paper
正在生成论文摘要