A Temporal Action Evaluation Algorithm for Medical Clinical Skill Operations
2023 3rd International Conference on Electronic Information Engineering and Computer Communication (EIECC)(2023)
摘要
The introduction of temporal action detection for standardised operations in medical clinical skills testing scenarios can both assist in guiding students' learning during teaching and increase the objectivity of the marking. Transformer is now becoming popular for temporal action detection, but Transformer focuses more on the temporal dimension of information. Meanwhile, the anchor-free based temporal action detection only generates a small number of proposals, and the accuracy of action boundary regression is relatively weak. According to the above problems, the Transformer algorithm based on feature enhancement and exact boundary regression (FEBRFormer) for temporal action evaluation is proposed. A multi-branch feature enhanced network (MuFEN) is utilised to enable features to be learned stronger at the channel level. A multi-stage channel regression head (MuCRH) is utilised to generate more accurate boundary regression locations in a smaller number of proposals. This is further used as a basis for proposing an action evaluation module (AEM), which mimics the teacher's scoring of a set number of rating points by training a weakly supervised based classifier. The experimental results demonstrate that FEBRFormer has exhibited performance enhancements of 0.8% and 0.5% in the context of action detection tasks when compared to the baseline model across two publicly available datasets, Thumos14 and ActivityNet1.3, respectively. In the self-made obstetric palpation and cardiopulmonary resuscitation datasets, there were 2.2% and 1.8% improvements compared to the baseline model, respectively.
更多查看译文
关键词
temporal action detection,medical clinical skill operations,action evaluation,attention mechanism
AI 理解论文
溯源树
样例
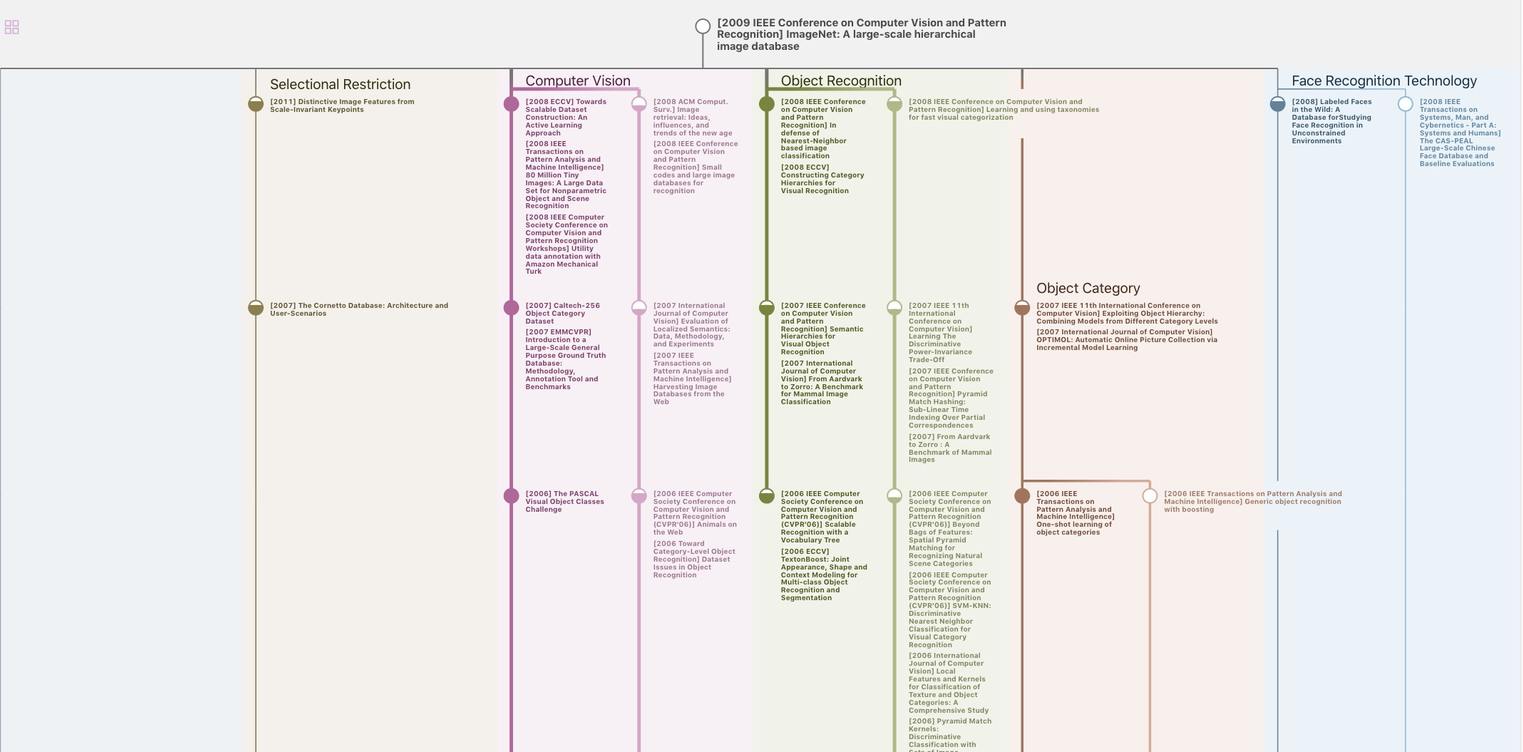
生成溯源树,研究论文发展脉络
Chat Paper
正在生成论文摘要