ALLFA: Active Learning Through Label and Feature Augmentation.
International Conference on Machine Learning and Applications(2023)
摘要
We introduce a novel feature-based active learning approach that leverages leading feature selection methods from explainable AI (XAI) to elicit user corrections on both the classification label and the leading features contributing to the classification decision. Uniquely, our approach can handle such user corrections, which identify missing and superfluous features in a model-agnostic manner that can be applied to any supervised classification model. Our approach generates augmented samples in a fine-grained manner according to user corrections, and then adds them to the training data to teach relevant and irrelevant features. Our results show that our approach outperforms traditional active learning and a leading baseline in two domains, which includes improvement in classification performance from 0.572 to 0.637 given the same number of queries.
更多查看译文
关键词
Active Learning,eXplainable AI,Human Activity Recognition (HAR)
AI 理解论文
溯源树
样例
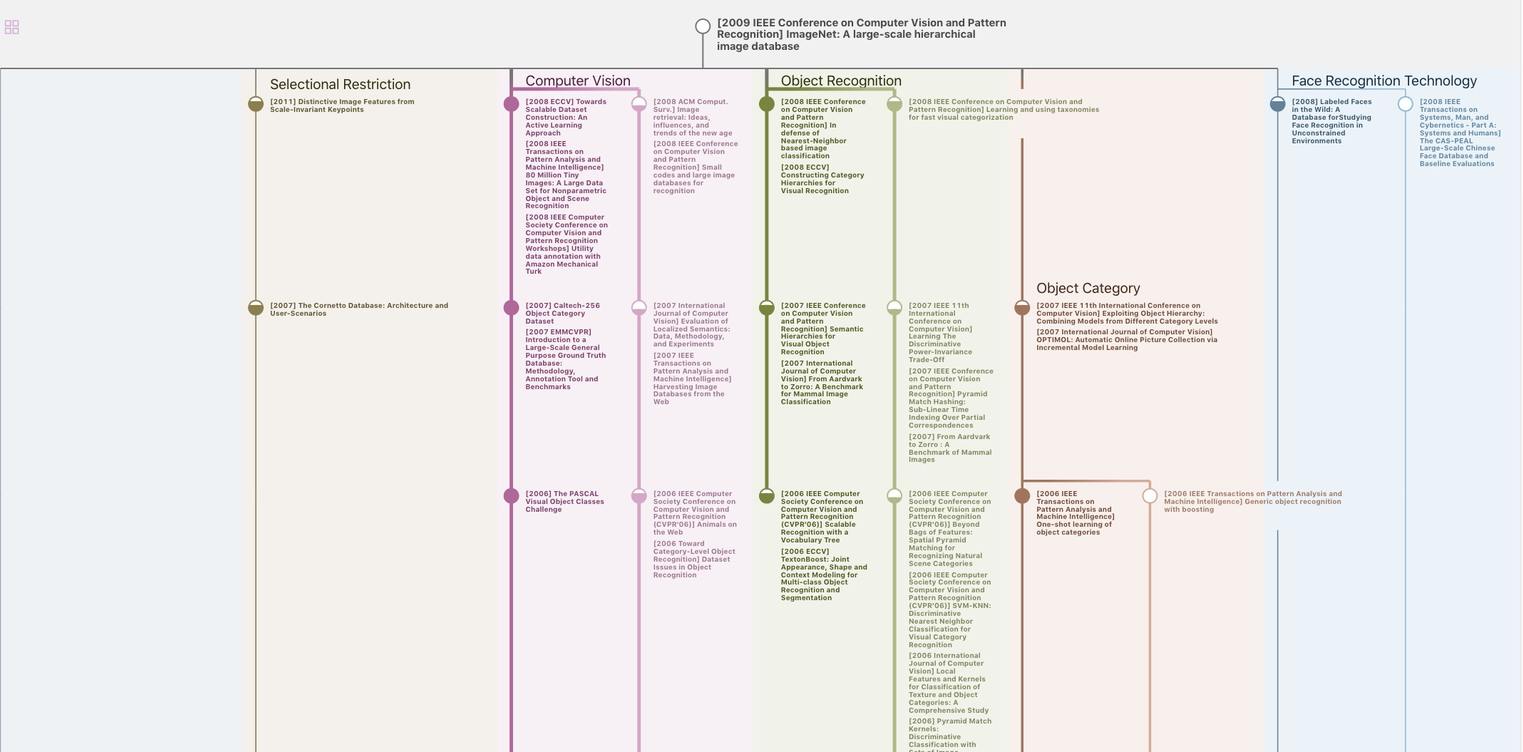
生成溯源树,研究论文发展脉络
Chat Paper
正在生成论文摘要