Robust Learning Under Label Noise by Optimizing the Tails of the Loss Distribution.
International Conference on Machine Learning and Applications(2023)
摘要
We introduce a novel optimization strategy that combines empirical risk minimization (ERM) with a sample weighting scheme to decrease the bias induced by training with noisy labels. We accomplish this by penalizing the tails of the loss distribution, as samples associated with the right tail (high loss values) are more likely to be mislabeled. We implement the proposed sample weighting scheme by minimizing a discriminatory risk that downweighs mislabeled samples. Moreover, we show that the proposed method enables us to optimize the location and shape of the loss distribution simultaneously. In addition, we couple our method with a distributionally robust optimization stage. The goal of this coupling is to increase the weight of correctly labeled but hard-to-Iearn samples that exhibit a high loss value. This coupling also improves model accuracy on underrepresented minority subsets when training with imbalanced classes. Experimental results show that for 60% noise contamination, our method, when compared to the ERM, improves the final accuracy on both Fashion-MNIST and CIFAR-10 by 18.4% and 8.5%, respectively.
更多查看译文
关键词
Higher-order statistics,image classification
AI 理解论文
溯源树
样例
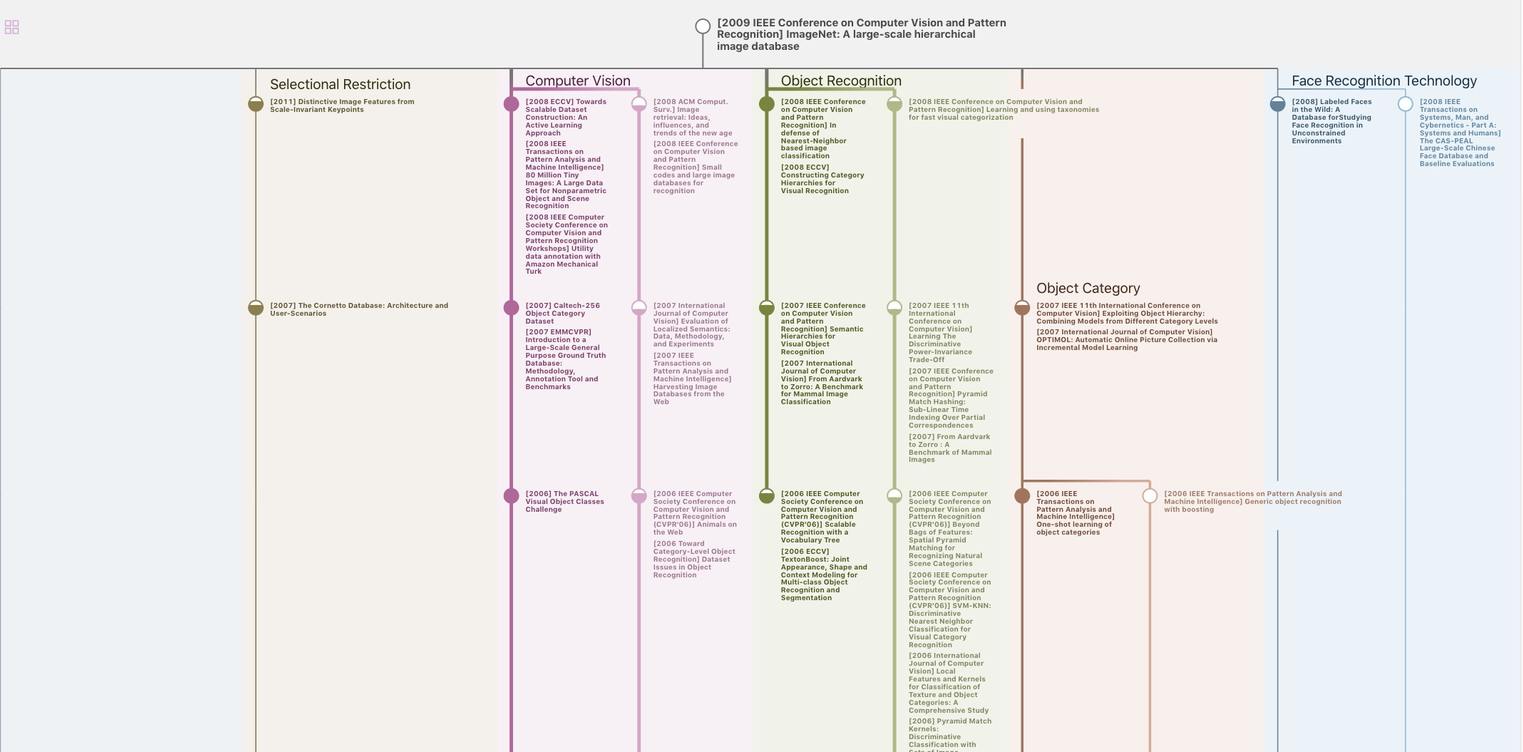
生成溯源树,研究论文发展脉络
Chat Paper
正在生成论文摘要