Unsupervised Segmentation and Data Augmentation in Image Sequences of Skeletal Muscle Contraction by Cycle-Consistent Generative Adversarial Network
2023 International Conference on Modeling, Simulation & Intelligent Computing (MoSICom)(2023)
摘要
This paper investigates a method addressing the unsupervised segmentation and joint data augmentation in medical ultrasound imaging based on the modified CycleGAN. Accurate quantification of fascia and muscle is the key for the diagnostics of neuromuscular disorders based on the analysis of image sequences of skeletal muscle contraction. Although the Deep Learning (DL) models represent encouraging results, some challenges exist. The traditional models don’t consider the complex interaction between tissues within a muscle and its surroundings, which reduces the performance of the fascia segmentation. Also, the DL requires many annotated datasets, which ignores dealing with noisy and complex ultrasound images. To overcome these issues, we propose a method to generate realistic images, and then present an unsupervised fascia segmentation method. The results show that our method improves the segmentation accuracy in noisy and complex ultrasound images compared to the traditional methods.
更多查看译文
关键词
unsupervised segmentation,ultrasound imaging,skeletal muscle contraction,fascia,CycleGAN,segmentation
AI 理解论文
溯源树
样例
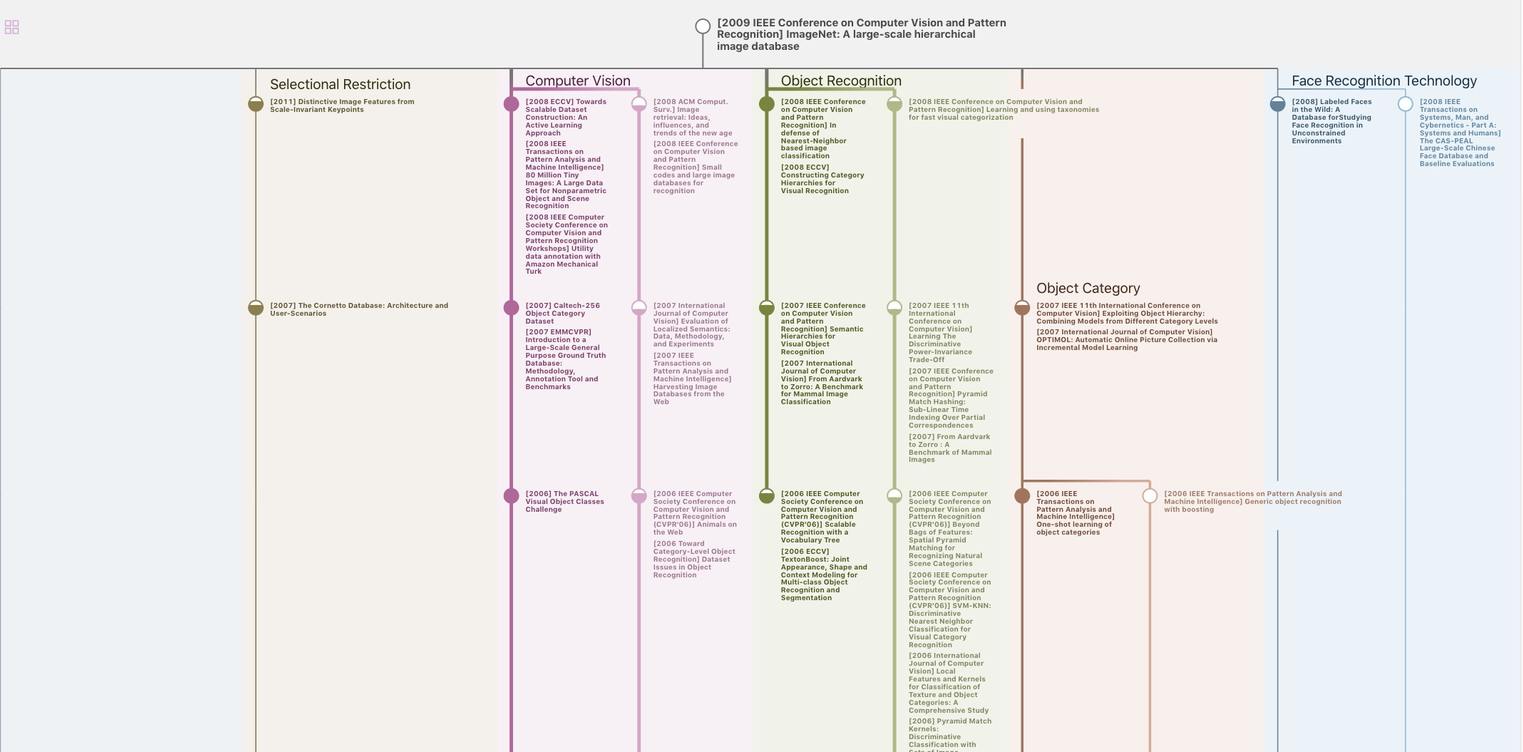
生成溯源树,研究论文发展脉络
Chat Paper
正在生成论文摘要