EvilELF: Evasion Attacks on Deep-Learning Malware Detection over ELF Files.
International Conference on Machine Learning and Applications(2023)
摘要
This paper investigates evasion attacks on end-to-end deep-learning malware detection over ELF (Executable and Linkable Format) binaries. We show that an attacker can deliberately modify bytes in a malware ELF binary such that a well-trained neural network is misled and predicts it as benign. We examine five methods that can modify ELF binaries without affecting functionalities and leverage them in evasion attacks. We explore two state-of-the-art end-to-end deep learning malware detectors, including MalConv and FireEyeNet, over a real-world dataset with 1,422 ELF binaries. Our experimental results show that evasion attacks with 3 out of the 5 methods are effective and can force the two CNNs to predict incorrectly. For instance, the most effective modification achieves up to 76.6% evasion rate on FireEyeNet and 8.4% evasion rate on MalConv. We also demonstrate that retraining CNNs with deliberately modified binaries can significantly mitigate evasion attacks.
更多查看译文
关键词
evasion attacks,malware detection,deep learning
AI 理解论文
溯源树
样例
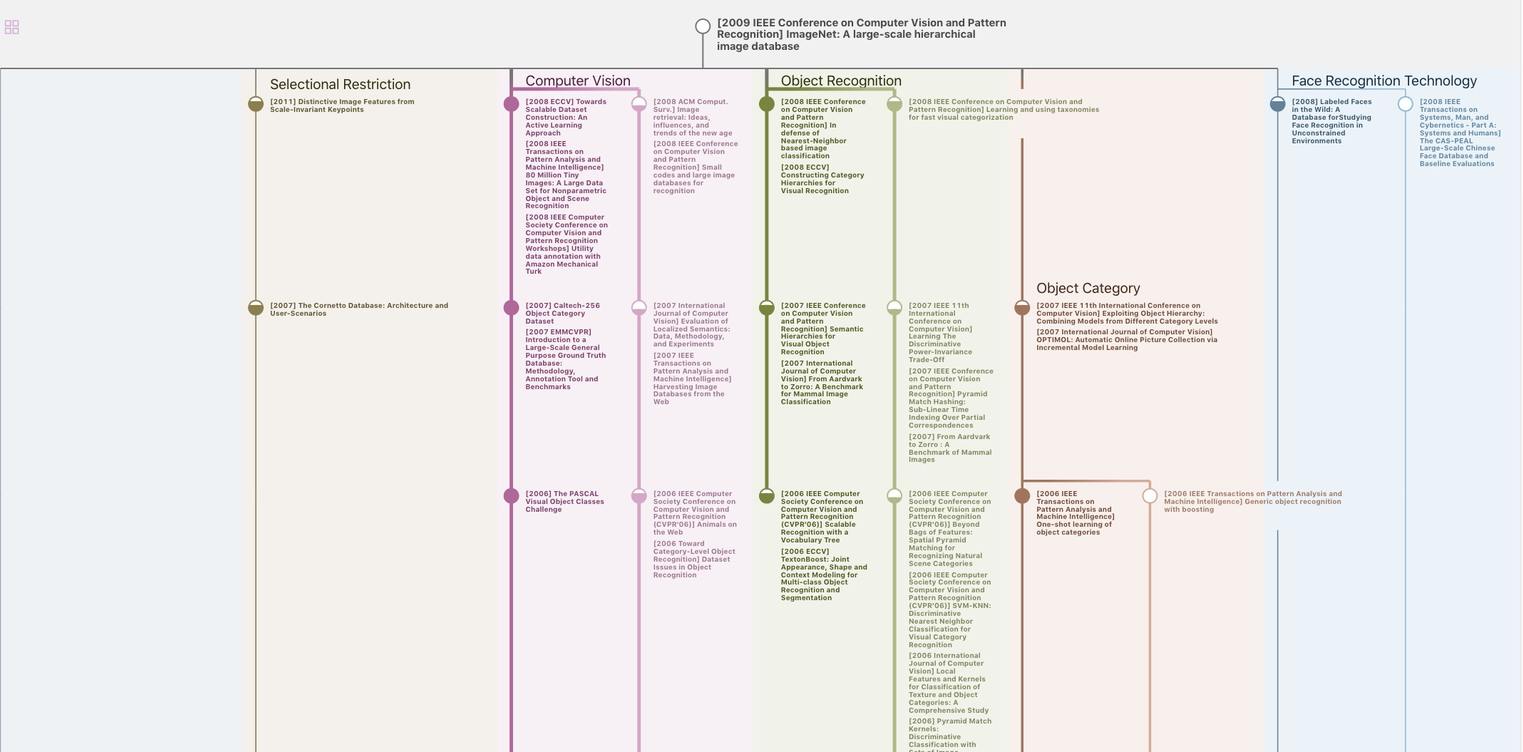
生成溯源树,研究论文发展脉络
Chat Paper
正在生成论文摘要