Node Selection in Federated Learning Using Sparsity Regularization and Compressive Sensing.
International Conference on Machine Learning and Applications(2023)
摘要
In the era of decentralized data and federated learning, node selection plays a pivotal role in ensuring efficient and effective model aggregation while optimizing communication overhead. In this research paper, we propose a novel approach for node selection in federated learning by integrating sparsity regularization and compressive sensing techniques. Federated learning allows training machine learning models on decentralized data sources while preserving privacy and data locality. The node selection process significantly influences model aggregation efficiency and effectiveness. Our approach utilizes sparsity regularization and compressive sensing with Bayesian learning to identify a sparse subset of informative nodes, emphasizing the inclusion of nodes with relevant and non-redundant information for model convergence, thereby reducing communication and computational overhead. Through extensive experimental evaluations on various datasets, we demonstrate significant improvements in model accuracy and convergence rates compared to existing methods, showcasing the remarkable effectiveness of our approach in selecting informative nodes for the success of federated learning applications. The results affirm the potential of our approach in addressing data heterogeneity while advancing efficient, and scalable decentralized machine learning across diverse domains.
更多查看译文
关键词
federated learning,node selection,compressive sensing
AI 理解论文
溯源树
样例
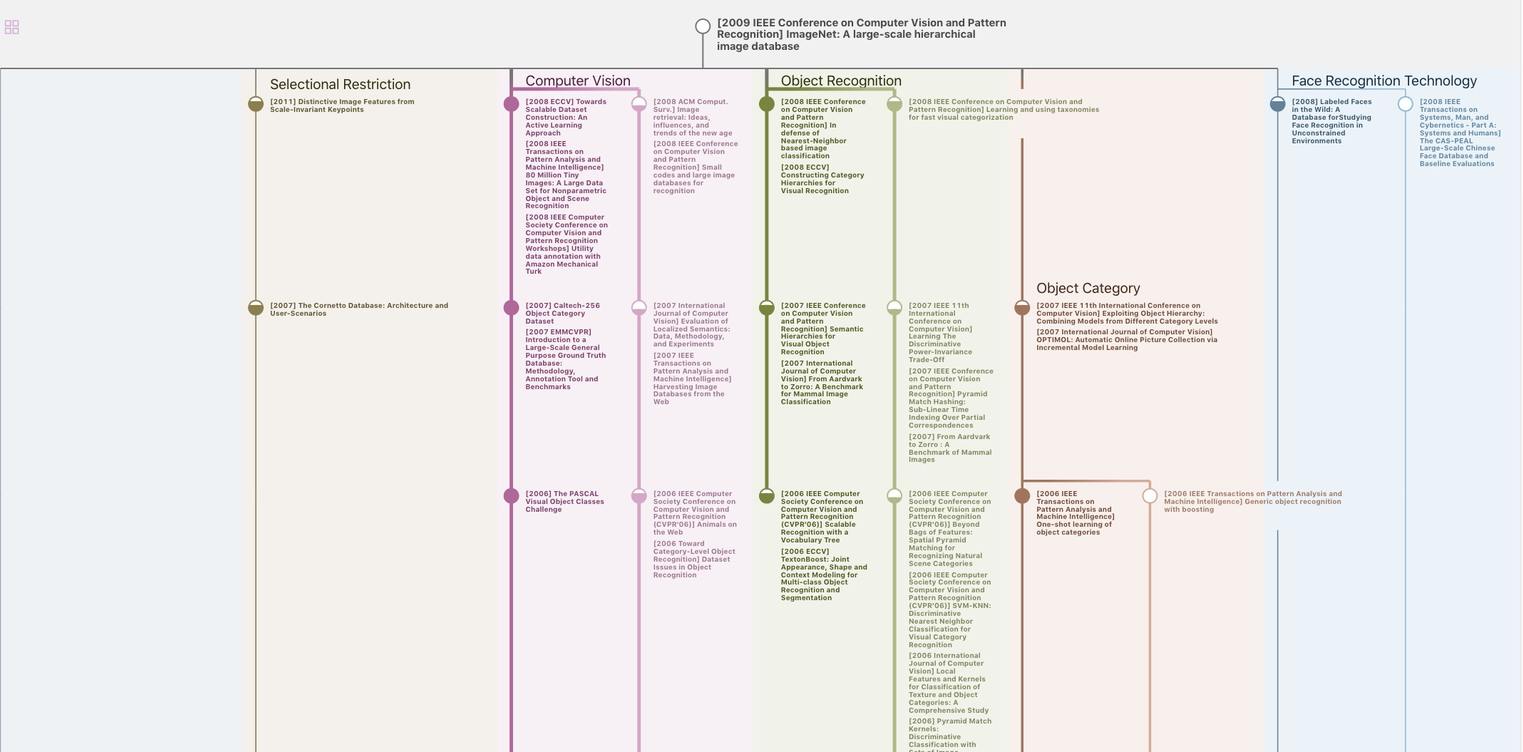
生成溯源树,研究论文发展脉络
Chat Paper
正在生成论文摘要