Image Inpainting Based on Efficient Group Attention Generative Adversarial Networks
2023 China Automation Congress (CAC)(2023)
摘要
Inpainting methods based on generative adversarial networks are an important class in the field, and their excellent generative ability can effectively improve the details of the inpainting results. However, generative adversarial networks inherently suffer from training difficulties and model collapse, leading to the large size of models based on such structures and equally difficult training. In addition, most methods incorporate an attention mechanism to improve the inpainting accuracy, which leads to the loss of boundary details of images in the process of restoration, affecting the results. To address the above problems, we propose an inpainting method based on efficient group attention generative adversarial networks (EGA-GAN). The proposed method uses cascaded group attention modules and feature-aligned pyramid networks in the generator. The cascaded group attention module can effectively save computational costs and reduce computational redundancy, and the feature-aligned pyramid network can mitigate the loss of boundary details in the downsampling operation to some extent. Experimental results on two commonly used inpainting datasets, Paris Street View and CelebA-HQ, show that the proposed method can effectively accelerate the speed of restoration and improve the accuracy of results.
更多查看译文
关键词
cascaded group attention,feature alignment,generative adversarial networks,image inpainting
AI 理解论文
溯源树
样例
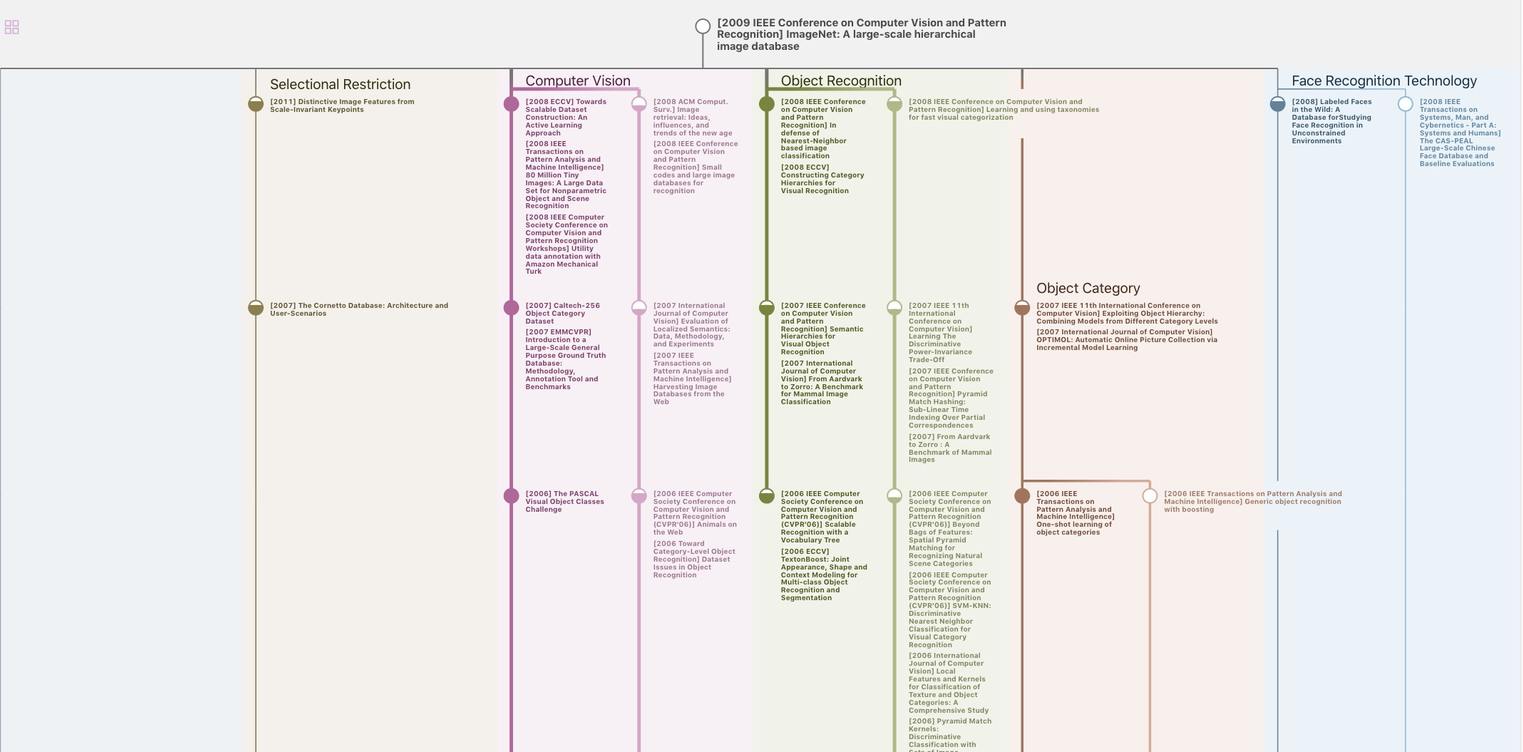
生成溯源树,研究论文发展脉络
Chat Paper
正在生成论文摘要