LiViT-Net: A U-Net-like, Lightweight Transformer Network for Retinal Vessel Segmentation
Computational and Structural Biotechnology Journal(2024)
摘要
The intricate task of precisely segmenting retinal vessels from images, which is critical for diagnosing various eye diseases, presents significant challenges for models due to factors such as scale variation, complex anatomical patterns, low contrast, and limitations in training data. Building on these challenges, we offer novel contributions spanning model architecture, loss function design, robustness, and real-time efficacy. To comprehensively address these challenges, a new U-Net-like, lightweight Transformer network for retinal vessel segmentation is presented. By integrating MobileViT+ and a novel local representation in the encoder, our design emphasizes lightweight processing while capturing intricate image structures, enhancing vessel edge precision. A novel joint loss is designed, leveraging the characteristics of weighted cross-entropy and Dice loss to effectively guide the model through the task's challenges, such as foreground-background imbalance and intricate vascular structures. Exhaustive experiments were performed on three prominent retinal image databases. The results underscore the robustness and generalizability of the proposed LiViT-Net, which outperforms other methods in complex scenarios, especially in intricate environments with fine vessels or vessel edges. Importantly, optimized for efficiency, LiViT-Net excels on devices with constrained computational power, as evidenced by its fast performance. To demonstrate the model proposed in this study, a freely accessible and interactive website was established (https://hz-t3.matpool.com:28765?token=aQjYR4hqMI), revealing real-time performance with no login requirements.
更多查看译文
关键词
Retinal Vessel Segmentation,Transformer,Lightweight,Joint Loss
AI 理解论文
溯源树
样例
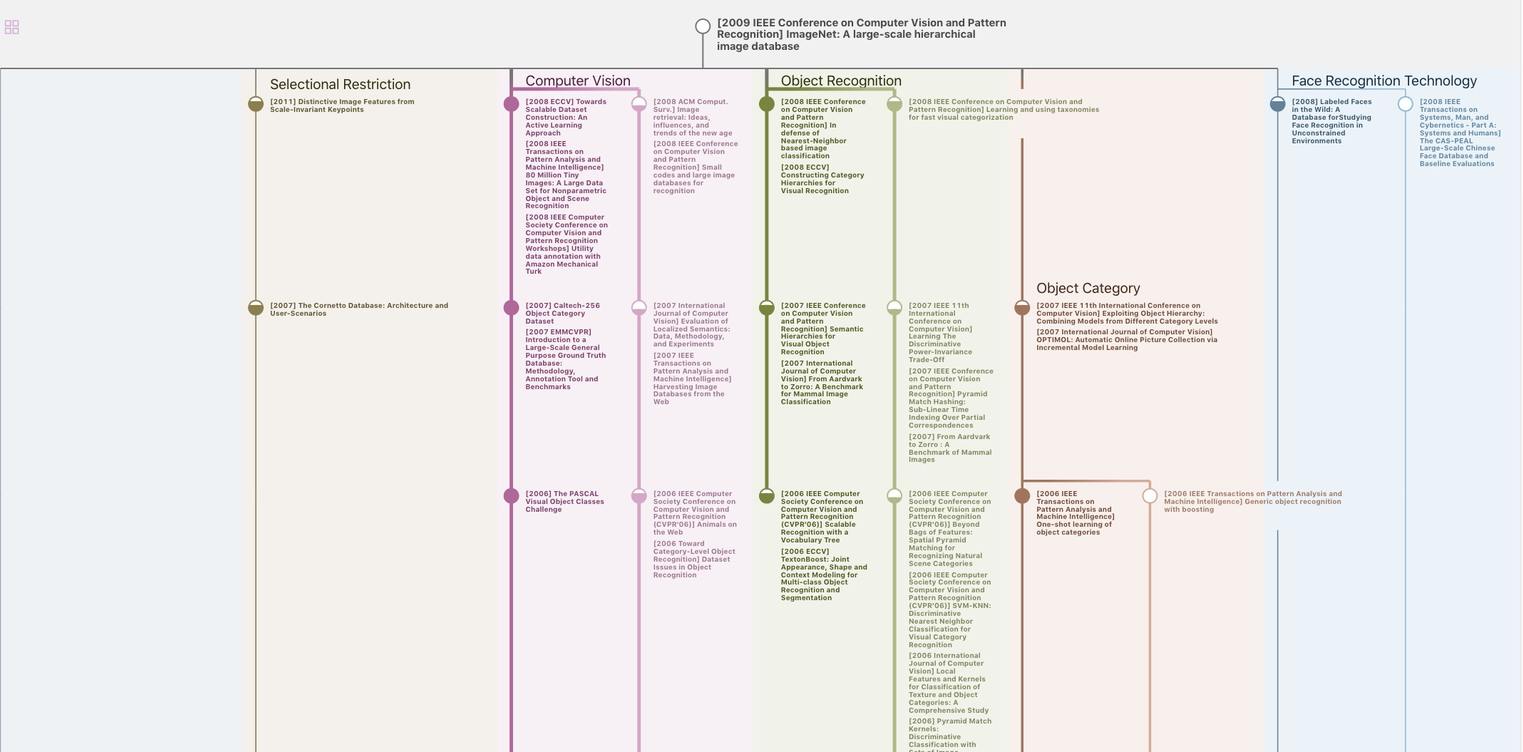
生成溯源树,研究论文发展脉络
Chat Paper
正在生成论文摘要