Video summarization via knowledge-aware multimodal deep networks
Knowledge-Based Systems(2024)
摘要
Video summarization has unprecedented importance in facilitating the rapid browsing, retrieval, and comprehension of large numbers of videos. Benefiting from possessing rich prior knowledge of the raw video and the capability to filter less crucial frames by employing multimodal information, humans can condense a lengthy video into a compact and reasonable video summary. However, existing automated video summarization approaches struggle to determine which shots in a video are significant concurrently and robustly, which is detrimental to the generation of high-quality summaries. To improve the quality of video summaries further, drawing inspiration from human abilities, we propose a novel video summarization approach based on a knowledge-aware multimodal network (KAMN). In particular, we present a knowledge-based encoder to obtain the corresponding representation for each frame. This representation is composed of captured descriptive content and affections, which are retrieved from large-scale external knowledge bases. Owing to these knowledge bases, rich implicit knowledge is provided to better understand the viewed video. Moreover, to integrate the visual, audio, and implicit knowledge features more effectively and to identify valuable information across different modalities further, we design a fusion module to learn these multimodal feature relationships more thoroughly. KAMN operates in both unsupervised and supervised training modes. Objective quantitative experiments and subjective user studies were conducted using four publicly available datasets. The results verified the effectiveness of the proposed modules and demonstrated the superior performance yielded by our framework.
更多查看译文
关键词
Video summarization,Multimodal information,External knowledge
AI 理解论文
溯源树
样例
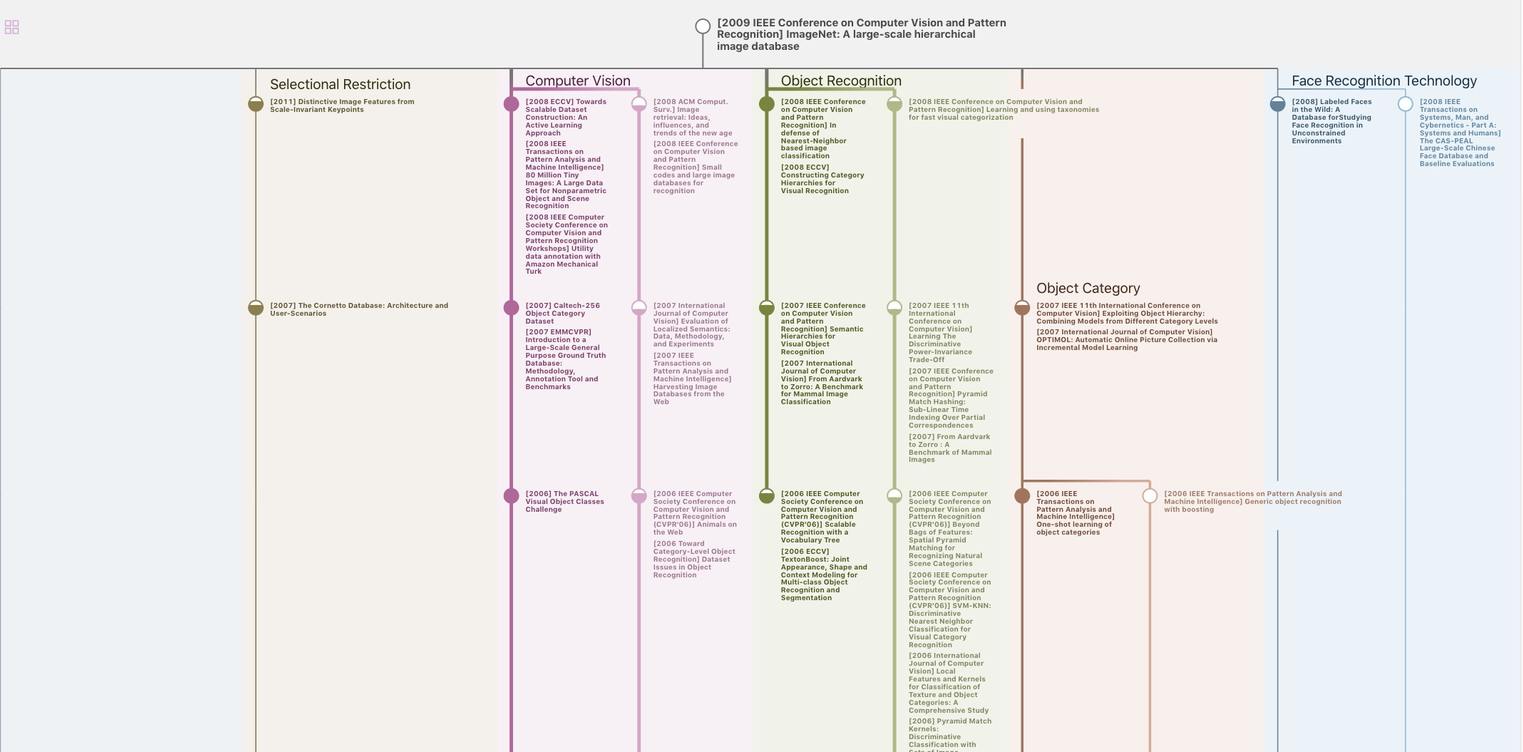
生成溯源树,研究论文发展脉络
Chat Paper
正在生成论文摘要