Domain Generalization of Chemical Process Fault Diagnosis by Maximizing Domain Feature Distribution Alignment
Process safety and environmental protection/Transactions of the Institution of Chemical Engineers Part B, Process safety and environmental protection/Chemical engineering research and design/Chemical engineering research & design(2024)
摘要
Fault detection and diagnosis (FDD) is crucial for ensuring safety and quality control in chemical industry. But data-driven FDD methods are usually trained with samples under certain operating conditions or data domains. This leads to their performance degradation caused by domain shifts in practice. To address this, a new FDD network, termed the DFDA model, has been designed to achieve domain generalization. Distribution of features extracted from fault samples is progressively aligned by supervised contrastive learning, learning vector quantization and gradient reversal adversarial learning in the DFDA model. So that FDD using such aligned features can potentially offer enhanced domain generalization capabilities. The performance of the DFDA model is benchmarked against other state-of-the-art FDD frameworks using data from various domains/modes of the Tennessee Eastman Process and an actual industrial process. The experimental outcomes indicate that the DFDA model consistently outperforms others in five distinct tasks, achieving an average Fault Detection Rate (FDR) that is 23% higher than that of ViT and 16% higher than CausalViT.
更多查看译文
关键词
Domain generalization,Fault detection and diagnosis,Feature distribution alignment,Vector quantization,Tennessee Eastman process
AI 理解论文
溯源树
样例
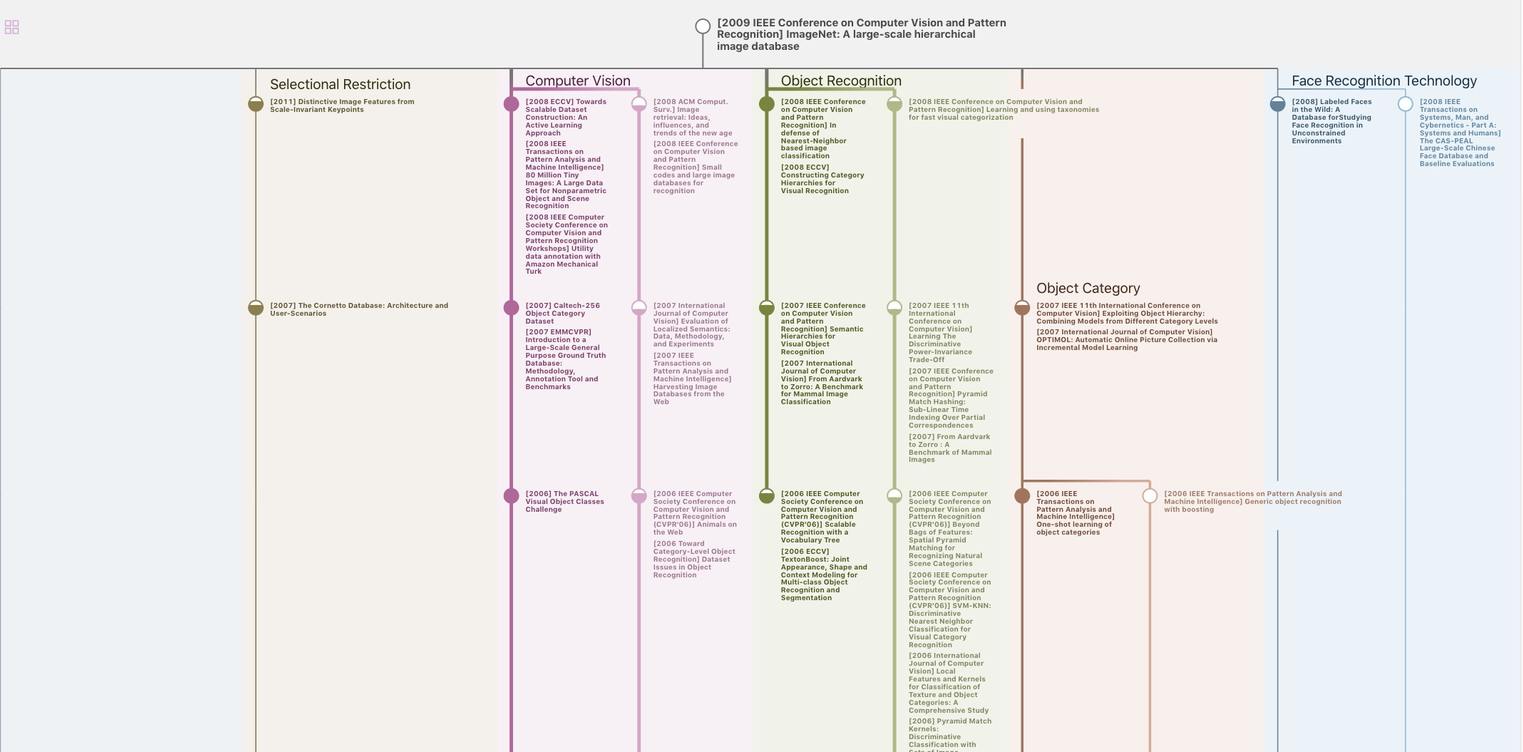
生成溯源树,研究论文发展脉络
Chat Paper
正在生成论文摘要