SIGMA: A Dataset for Text-to-Code Semantic Parsing with Statistical Analysis.
International Conference on Machine Learning and Applications(2023)
摘要
In the semantic parsing domain, significant progress has been achieved in Text-to-SQL and question-answering tasks, both focused on extracting information from data sources in their native format. However, the inherent constraints of their formal meaning representations, such as SQL programming language, hinder their ability to analyze data from various perspectives, such as conducting statistical analyses. To address this limitation and inspire research in this field, we design SIGMA, a new dataset for Text-to-Code semantic parsing with statistical analysis. SIGMA consists of 6000 questions with corresponding Python code labels. The Python code labels in our dataset cover 4 types of query patterns, which return data in their original format, and 40 types of statistical analysis patterns, which perform statistical operations on the data. We evaluated the SIGMA dataset using three different baseline models: LGESQL, 5mBoP, and SLSQL. The experimental results show that the LGESQL model with ELECTRA outperforms all other models, achieving 83.37% structure accuracy. In terms of execution accuracy, the 5mBoP model, when combined with GraPPa and T5, reaches 76.38%.
更多查看译文
关键词
Semantic Parsing,Statistical Analysis,Text-to-Code
AI 理解论文
溯源树
样例
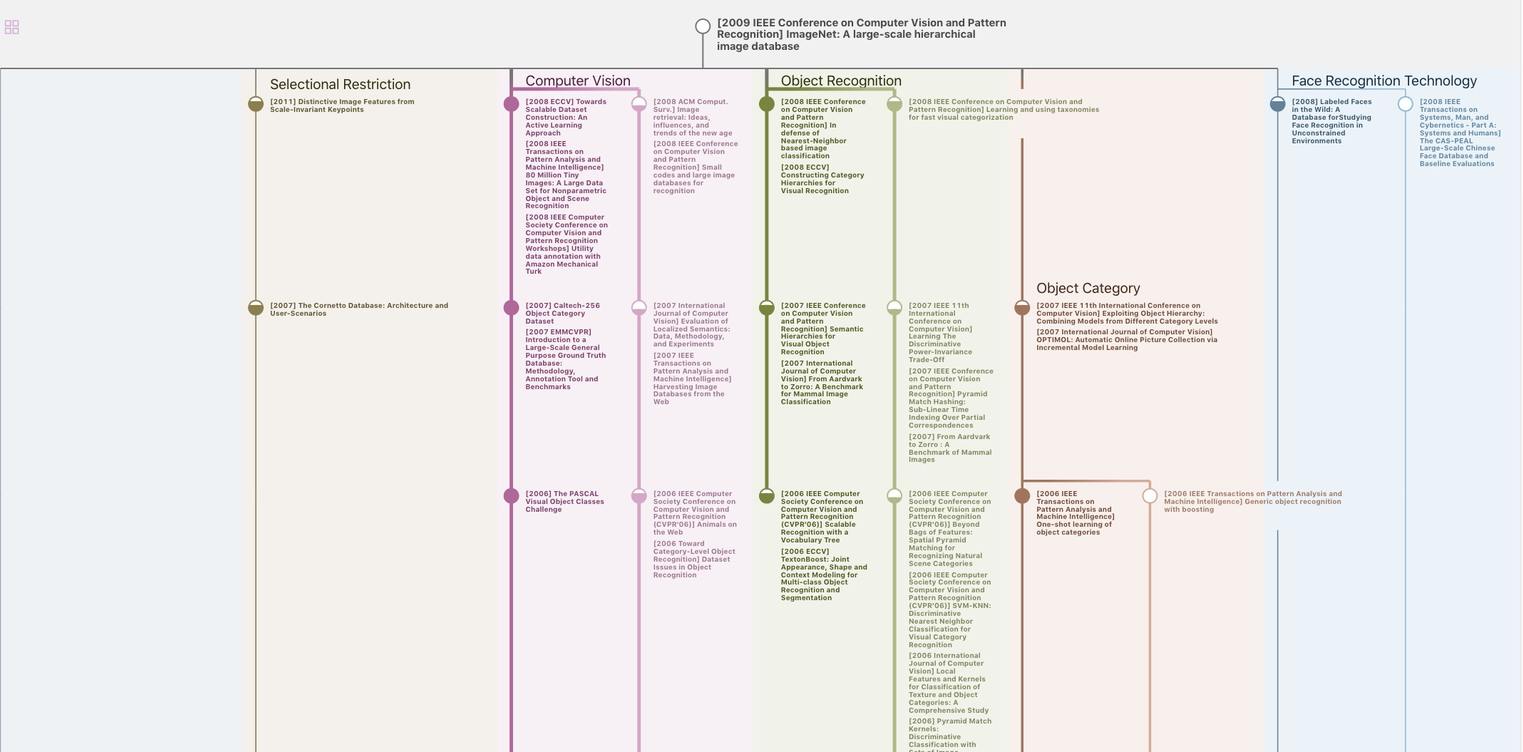
生成溯源树,研究论文发展脉络
Chat Paper
正在生成论文摘要