Noise-Aware Uncertainty Quantification of MLGNR Interconnects using Fast Trained Artificial Neural Networks
2023 IEEE Electrical Design of Advanced Packaging and Systems (EDAPS)(2023)
摘要
This paper presents a prior knowledge accelerated transfer learning technique to efficiently train artificial neural networks (ANNs) to address uncertainty in on-chip multilayer graphene nanoribbon (MLGNR) interconnects. The salient feature of the proposed training technique is that it accounts for the variation in the amplitude, frequency, and phase angle of the power supply noise and ground bounce signals. Consequently, the resultant ANN model is noise-aware and can be used to anticipate the eye diagram attributes of the MLGNR interconnects for any arbitrary noise signal without the need for any retraining.
更多查看译文
关键词
Artificial neural networks (ANNs),ground bounce,multilayer graphene nanoribbons (MLGNRs),on-chip interconnects,power supply noise,uncertainty quantification
AI 理解论文
溯源树
样例
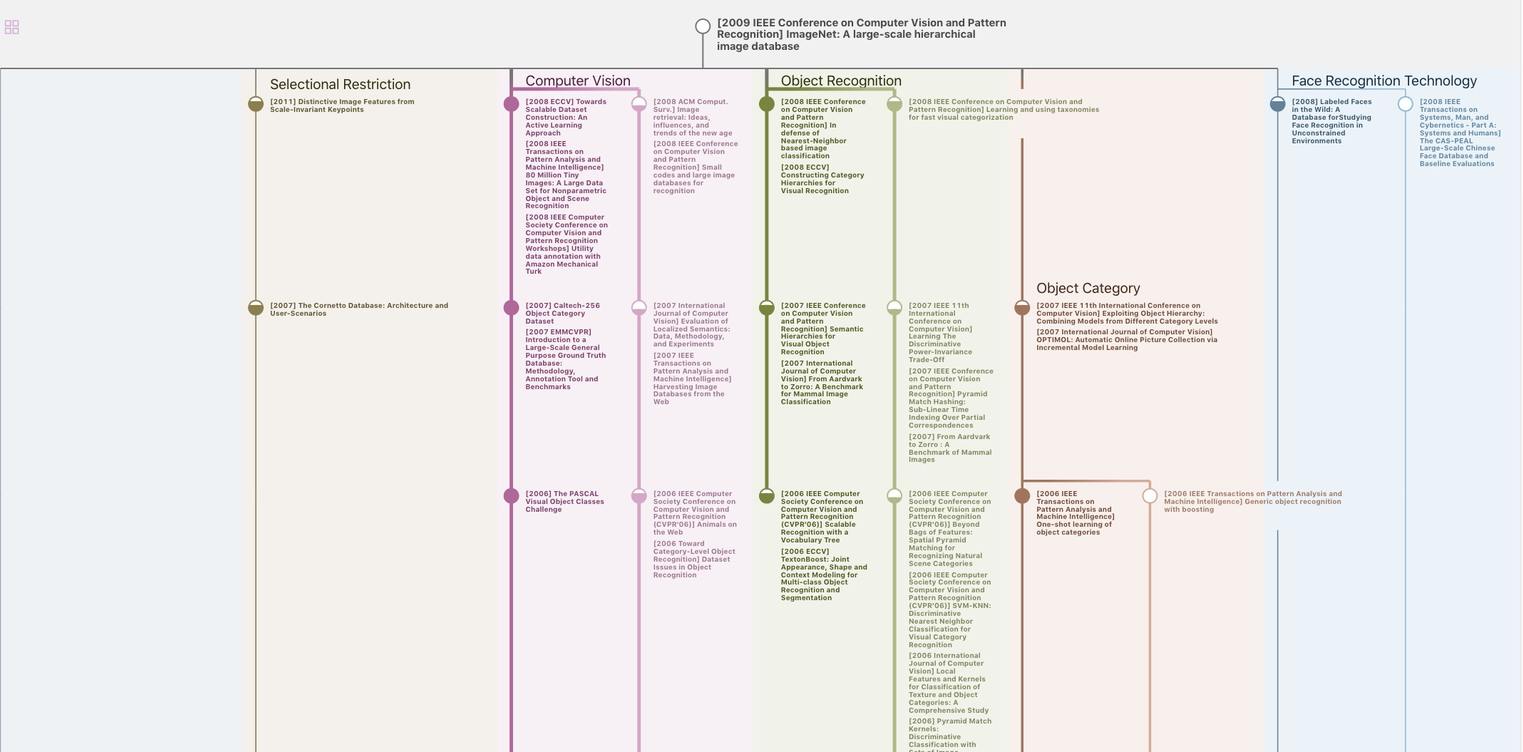
生成溯源树,研究论文发展脉络
Chat Paper
正在生成论文摘要